Dynamic Imposter Based Online Instance Matching for Person Search
Pattern Recognition(2020)
摘要
Person search aims to locate the target person matching a given query from a list of unconstrained whole images. It is a challenging task due to the unavailable bounding boxes of pedestrians, limited samples for each labeled identity and large amount of unlabeled persons in existing datasets. To address these issues, we propose a novel end-to-end learning framework for person search. The proposed framework settles pedestrian detection and person re-identification concurrently. To achieve the goal of co-learning and utilize the information of unlabeled persons, a novel yet extremely efficient Dynamic Imposter based Online Instance Matching (DI-OIM) loss is formulated. The DI-OIM loss is inspired by the observation that pedestrians appearing in the same image obviously have different identities. Thus we assign the unlabeled persons with dynamic pseudo-labels. The pseudo-labeled persons along with the labeled persons can be used to learn powerful feature representations. Experiments on CUHK-SYSU and PRW datasets demonstrate that our method outperforms other state-of-the-art algorithms. Moreover, it is superior and efficient in terms of memory capacity comparing with existing methods.
更多查看译文
关键词
Person search,Pedestrian detection,Person re-identification,Dynamic pseudo-label
AI 理解论文
溯源树
样例
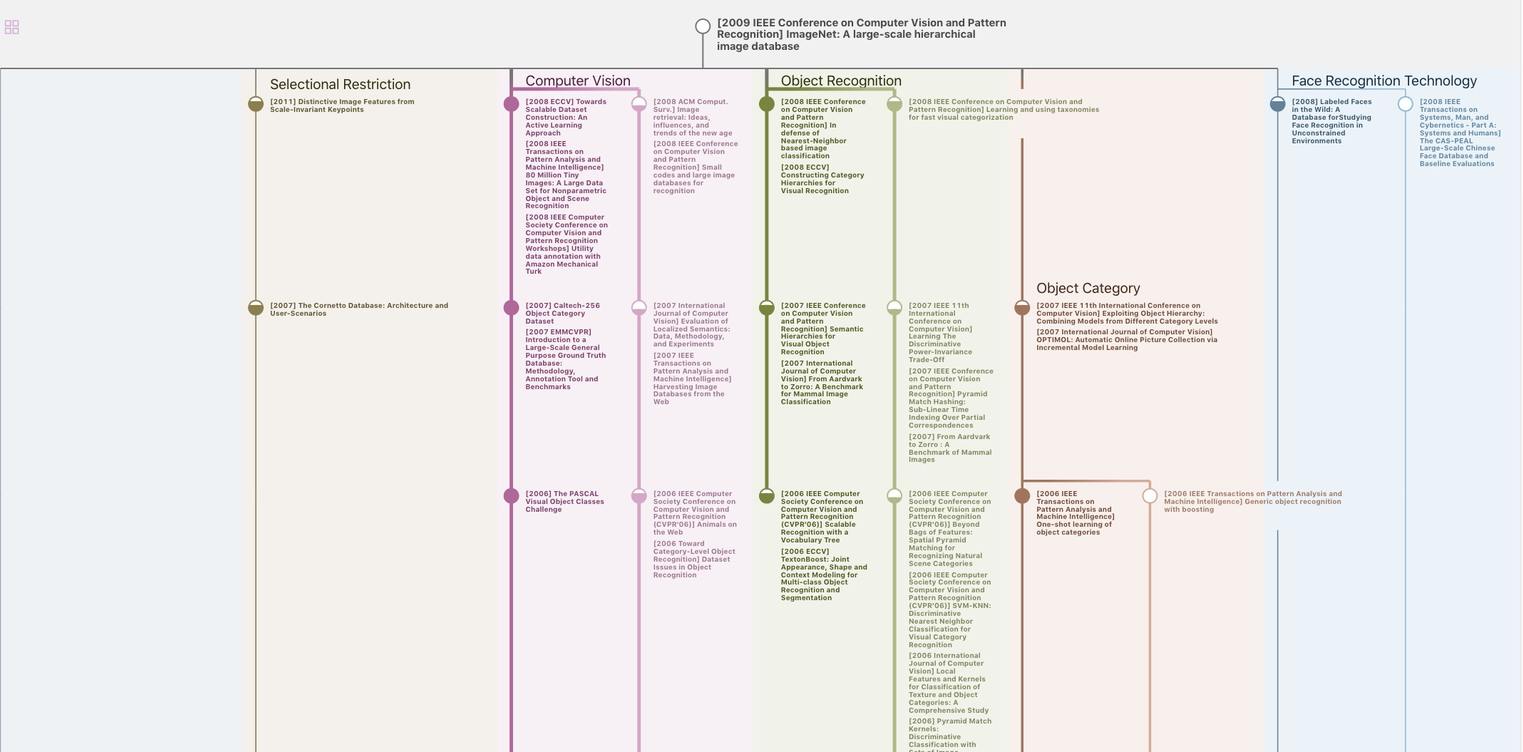
生成溯源树,研究论文发展脉络
Chat Paper
正在生成论文摘要