Deep Reinforcement Learning Based Mobility–Aware Robust Proactive Resource Allocation in Heterogeneous Networks
IEEE Transactions on Cognitive Communications and Networking(2020)
摘要
Proactive resource allocation (PRA) is an essential technology boosting intelligent communication, as it can make full use of prediction and significantly improve network performance. However, most promising gains base on perfect prediction which is unrealistic. How to make PRA robust against prediction uncertainty and maximize benefits brought by prediction becomes an important issue. In this paper, we tackle this problem and propose a mobility-aware robust PRA approach (MRPRA) in heterogeneous networks. MRPRA pre-allocates resources in both time and frequency domains among mobile users with users’ trajectories predicted by hidden Markov model. The objective is to minimize service delay under constraints of different levels of quality-of-service (QoS) requirement and mobility intensity. MRPRA is robust against prediction uncertainty by exploiting probabilistic constraint programming to model QoS requirements in a probabilistic sense. To this end, the probabilistic distribution of achievable rate is derived. To flexibly coordinate resource allocation among multiple mobile users over time horizon, a deep reinforcement learning based multi-actor deep deterministic policy gradient algorithm is designed. It learns robust PRA policies by distributed acting and centralized criticizing. Extensive numerical simulations are performed to analyze performances of the proposed approach.
更多查看译文
关键词
Heterogeneous networks,proactive resource allocation,mobility prediction,deep reinforcement learning,robustness
AI 理解论文
溯源树
样例
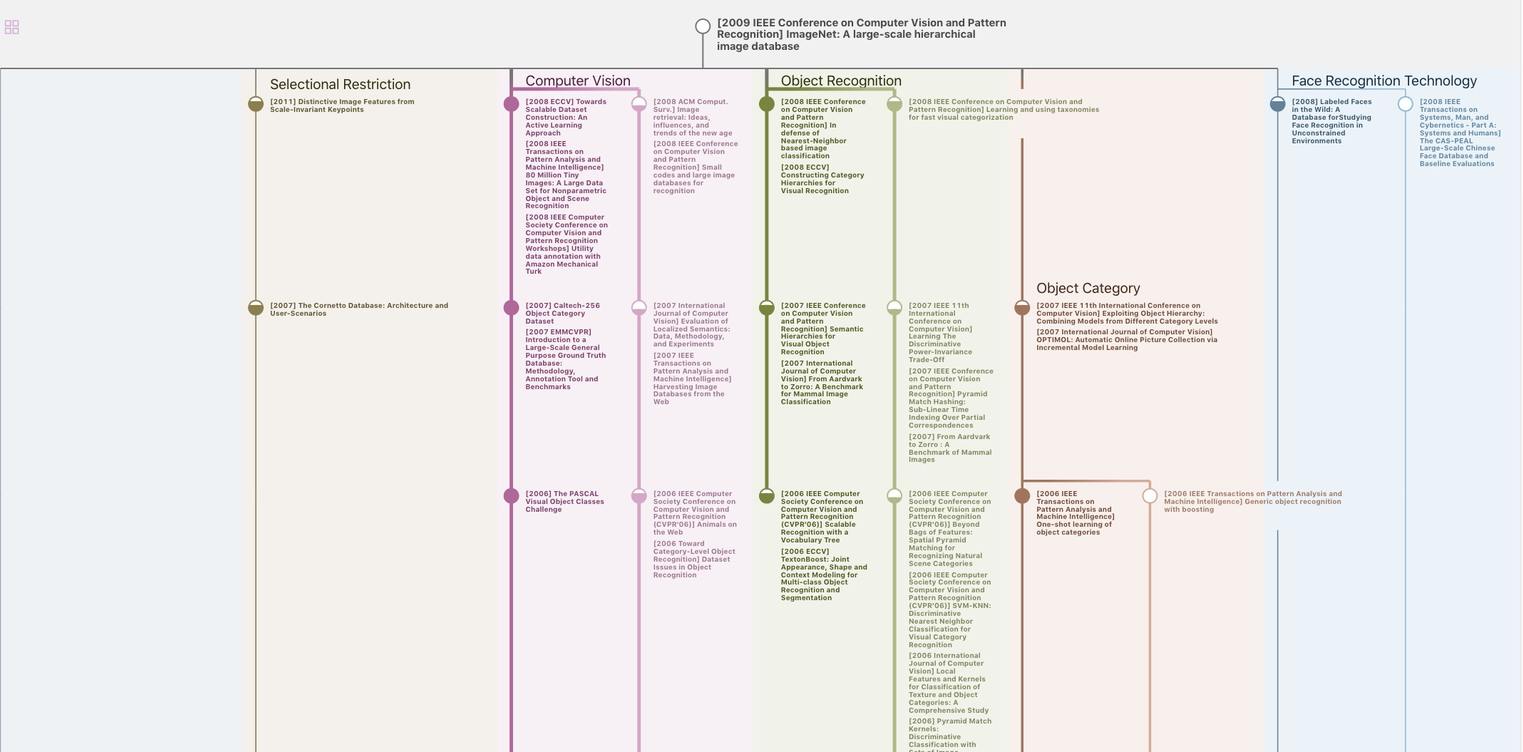
生成溯源树,研究论文发展脉络
Chat Paper
正在生成论文摘要