Classifying Prostate Histological Images Using Deep Gaussian Processes On A New Optical Density Granulometry-Based Descriptor
INTELLIGENT DATA ENGINEERING AND AUTOMATED LEARNING - IDEAL 2019, PT I(2019)
摘要
The increasing use of whole slide digital scanners has led to an enormous interest in the application of machine learning techniques to detect prostate cancer using eosin and hematoxylin stained histopathological images. In this work the above problem is approached as follows: the optical density of each whole slide image is calculated and its eosin and hematoxylin concentration components estimated. Then, hand-crafted features, which are expected to capture the expertise of pathologists, are extracted from patches of these two concentration components. Finally, patches are classified using a Deep Gaussian Process on the extracted features. The new approach outperforms current state of the art shallow as well as deep classifiers like InceptionV3, Xception and VGG19 with an AUC value higher than 0.98.
更多查看译文
关键词
Prostate cancer, Optical Density, Texture features, Morphological features, Deep Gaussian Processes
AI 理解论文
溯源树
样例
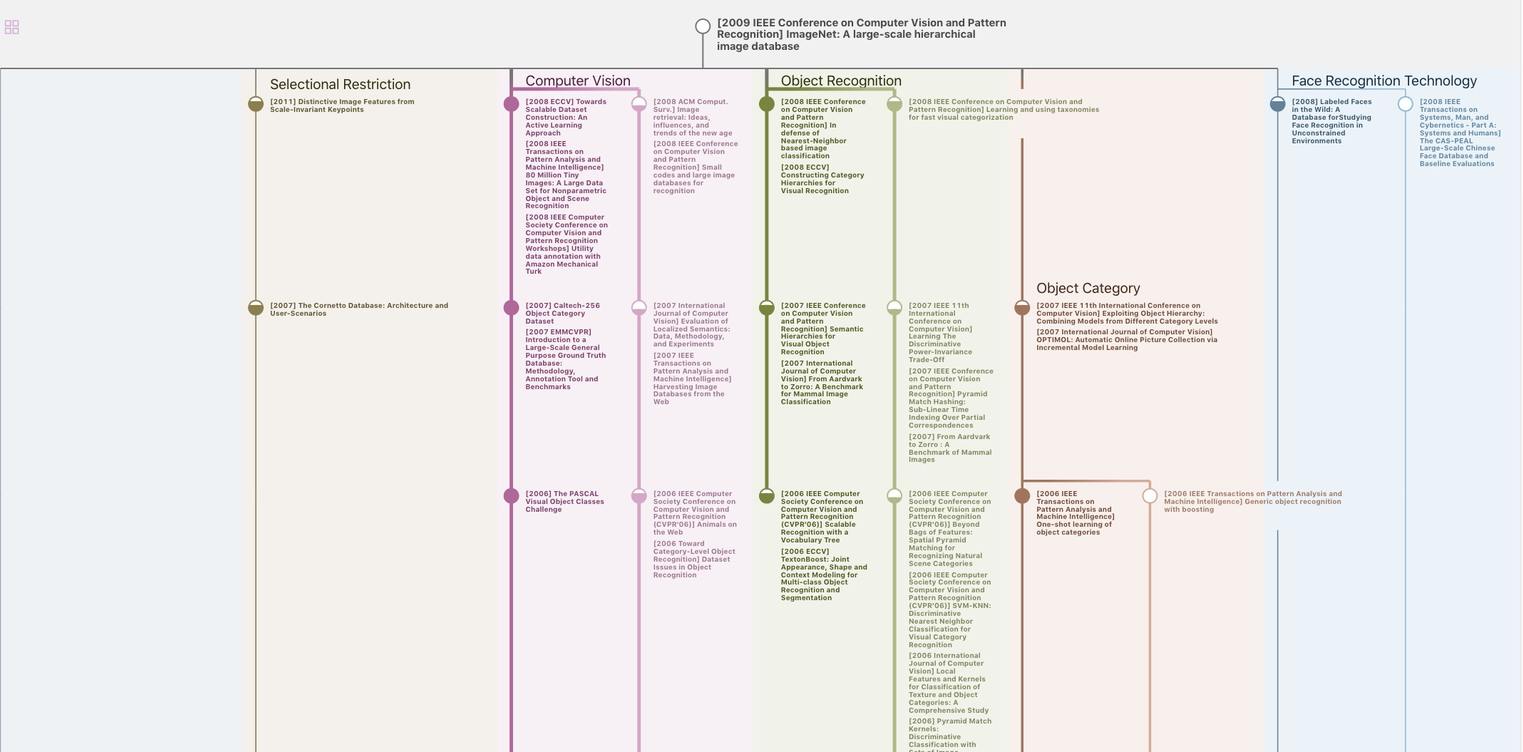
生成溯源树,研究论文发展脉络
Chat Paper
正在生成论文摘要