O2u-Net: A Simple Noisy Label Detection Approach For Deep Neural Networks
2019 IEEE/CVF INTERNATIONAL CONFERENCE ON COMPUTER VISION (ICCV 2019)(2019)
摘要
This paper proposes a novel noisy label detection approach, named O2U-net, for deep neural networks without human annotations. Different from prior work which requires specifically designed noise-robust loss functions or networks, O2U-net is easy to implement but effective. It only requires adjusting the hyper-parameters of the deep network to make its status transfer from overfitting to underfitting (O2U) cyclically. The losses of each sample are recorded during iterations. The higher the normalized average loss of a sample, the higher the probability of being noisy labels. O2U-net is naturally compatible with active learning and other human annotation approaches. This introduces extra flexibility for learning with noisy labels. We conduct sufficient experiments on multiple datasets in various settings. The experimental results prove the state-of-the-art of O2S-net.
更多查看译文
关键词
deep neural networks,human annotations,noise-robust loss functions,deep network,normalized average loss,human annotation approaches,noisy label detection approach,O2U-Net,O2S-net,overfitting to underfitting
AI 理解论文
溯源树
样例
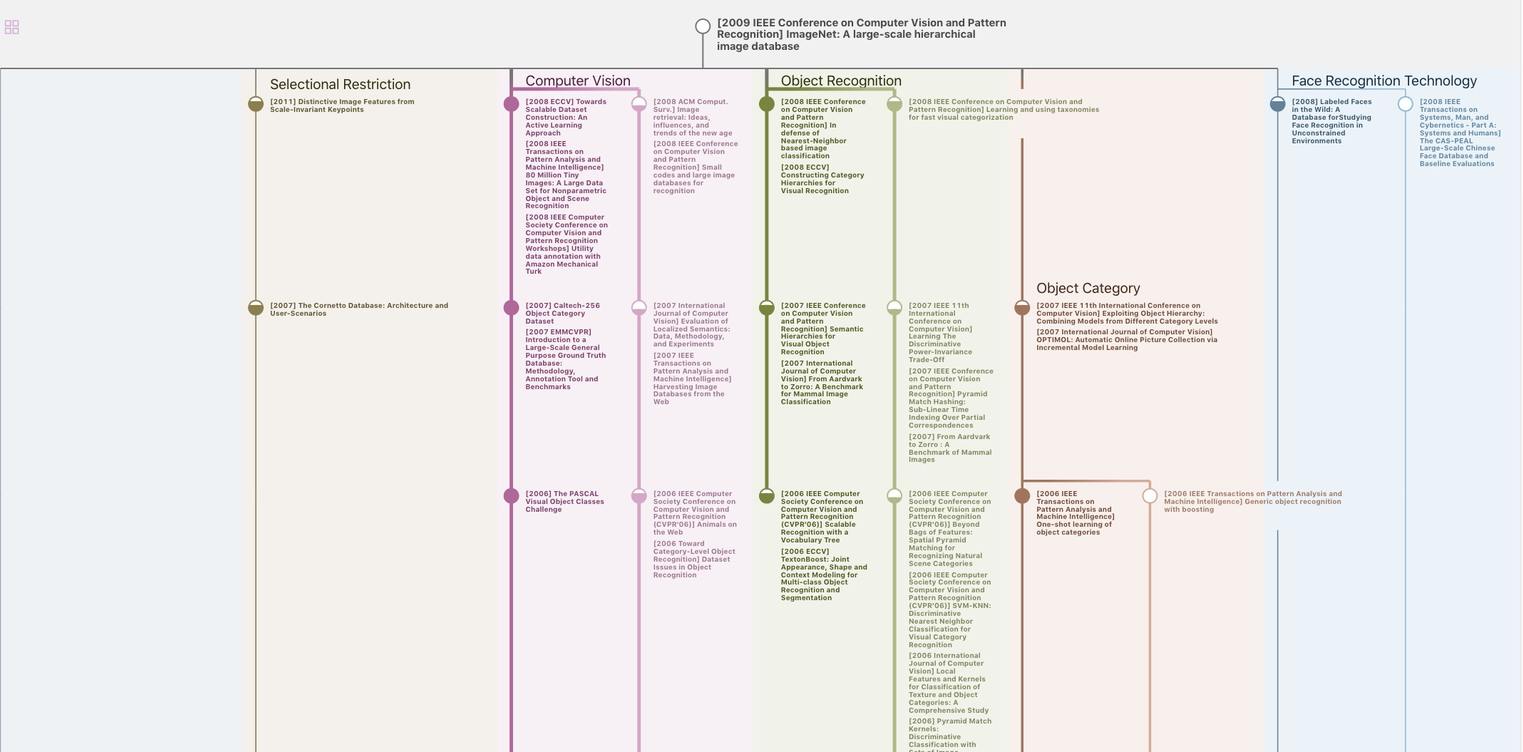
生成溯源树,研究论文发展脉络
Chat Paper
正在生成论文摘要