3D Design Using Generative Adversarial Networks and Physics-based Validation
JOURNAL OF MECHANICAL DESIGN(2020)
摘要
The authors present a generative adversarial network (GAN) model that demonstrates how to generate 3D models in their native format so that they can be either evaluated using complex simulation environments or realized using methods such as additive manufacturing. Once initially trained, the GAN can create additional training data itself by generating new designs, evaluating them in a physics-based virtual environment, and adding the high performing ones to the training set. A case study involving a GAN model that is initially trained on 4045 3D aircraft models is used for demonstration, where a training data set that has been updated with GAN-generated and evaluated designs results in enhanced model generation, in both the geometric feasibility and performance of the designs. Z-tests on the performance scores of the generated aircraft models indicate a statistically significant improvement in the functionality of the generated models after three iterations of the training-evaluation process. In the case study, a number of techniques are explored to structure the generate-evaluate process in order to balance the need to generate feasible designs with the need for innovative designs.
更多查看译文
关键词
computer-aided design,conceptual design,design automation
AI 理解论文
溯源树
样例
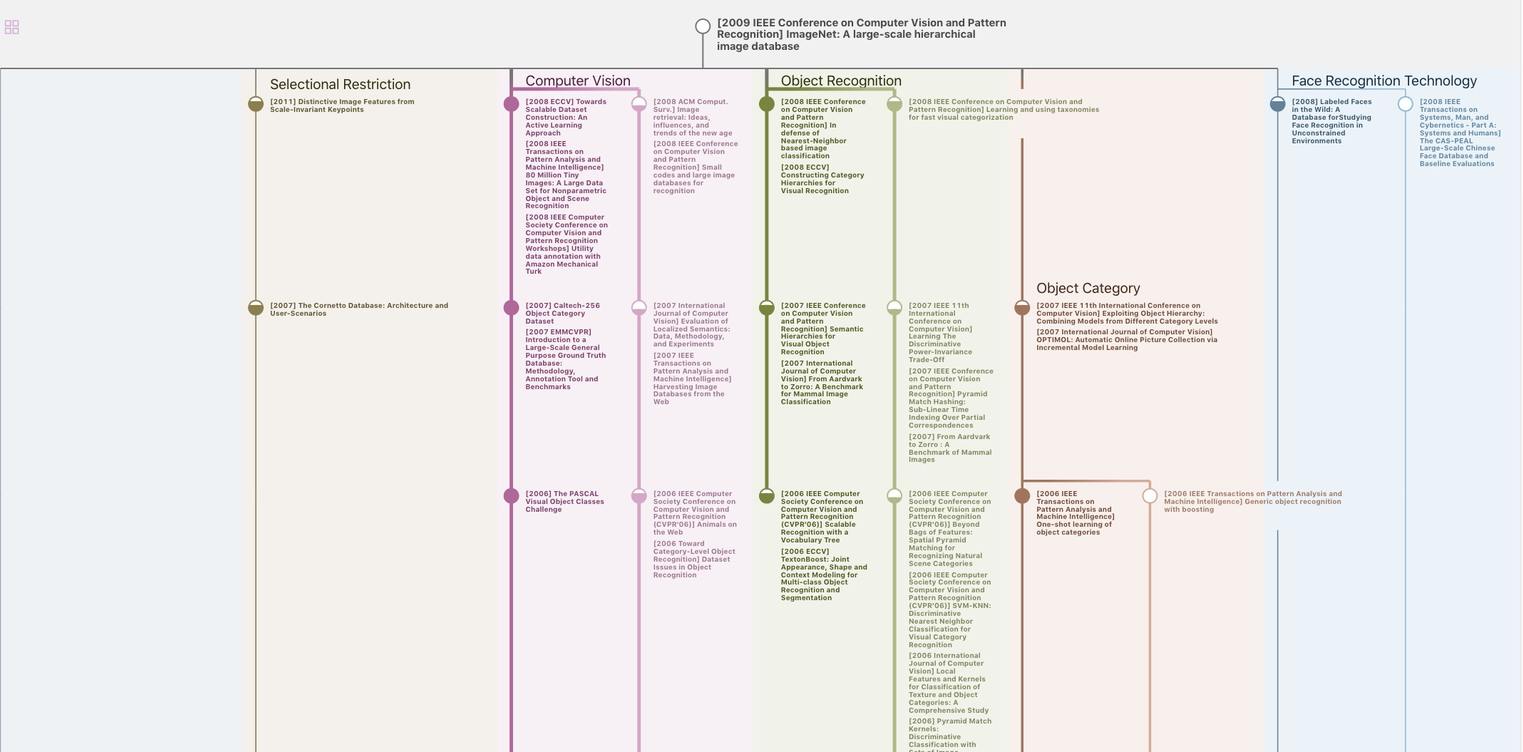
生成溯源树,研究论文发展脉络
Chat Paper
正在生成论文摘要