Pencil Drawing Video Rendering Using Convolutional Networks
COMPUTER GRAPHICS FORUM(2019)
摘要
Traditional pencil drawing rendering algorithms when applied to video may suffer from temporal inconsistency and shower-door effect due to the stochastic noise models employed. This paper attempts to resolve these problems with deep learning. Recently, many research endeavors have demonstrated that feed-forward Convolutional Neural Networks (CNNs) are capable of using a reference image to stylize a whole video sequence while removing the shower-door effect in video style transfer applications. Compared with video style transfer, pencil drawing video is more sensitive to the inconsistency of texture and requires a stronger expression of pencil hatching. Thus, in this paper we develop an approach by combining a latest Line Integral Convolution (LIC) based method, specializing in realistically simulating pencil drawing images, with a new feed-forward CNN that can eliminate the shower-door effect successfully. Taking advantage of optical flow, we adopt a feature-map-level temporal loss function and propose a new framework to avoid the temporal inconsistency between consecutive frames, enhancing the visual impression of pencil strokes and tone. Experimental comparisons with the existing feed-forward CNNs have demonstrated that our method can generate temporally more stable and visually more pleasant pencil drawing video results in a faster manner.
更多查看译文
AI 理解论文
溯源树
样例
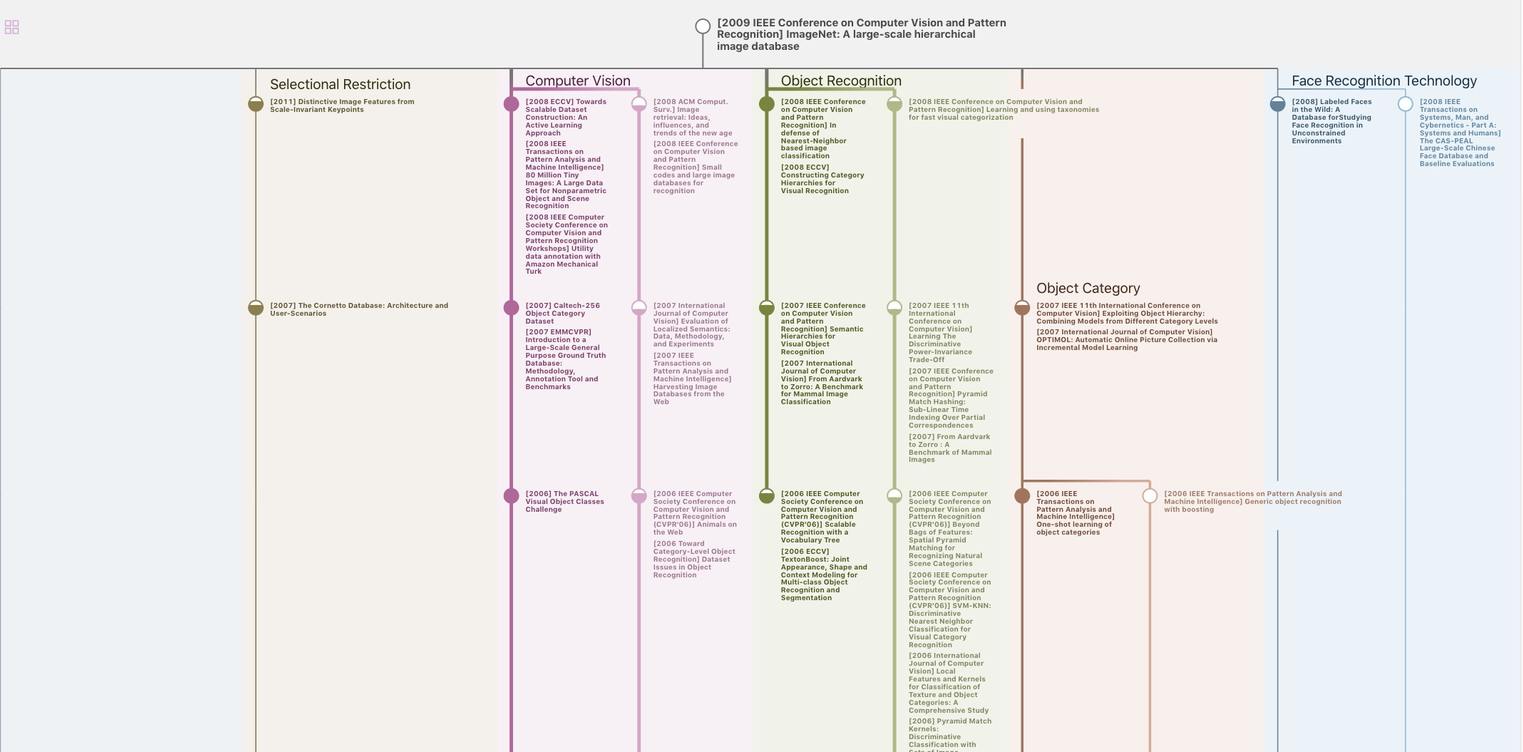
生成溯源树,研究论文发展脉络
Chat Paper
正在生成论文摘要