JPEG Artifacts Reduction via Deep Convolutional Sparse Coding
2019 IEEE/CVF INTERNATIONAL CONFERENCE ON COMPUTER VISION (ICCV 2019)(2019)
摘要
To effectively reduce JPEG compression artifacts, we propose a deep convolutional sparse coding (DCSC) network architecture. We design our DCSC in the framework of classic learned iterative shrinkage-threshold algorithm. To focus on recognizing and separating artifacts only, we sparsely code the feature maps instead of the raw image. The final de-blocked image is directly reconstructed from the coded features. We use dilated convolution to extract multi-scale image features, which allows our single model to simultaneously handle multiple JPEG compression levels. Since our method integrates model-based convolutional sparse coding with a learning-based deep neural network, the entire network structure is compact and more explainable. The resulting lightweight model generates comparable or better de-blocking results when compared with state-of-the-art methods.
更多查看译文
关键词
feature maps,raw image,multiscale image features,multiple JPEG compression levels,learning-based deep neural network,entire network structure,JPEG artifacts reduction,JPEG compression artifacts,deep convolutional sparse coding network architecture,DCSC,classic learned iterative shrinkage-threshold algorithm,recognizing separating artifacts
AI 理解论文
溯源树
样例
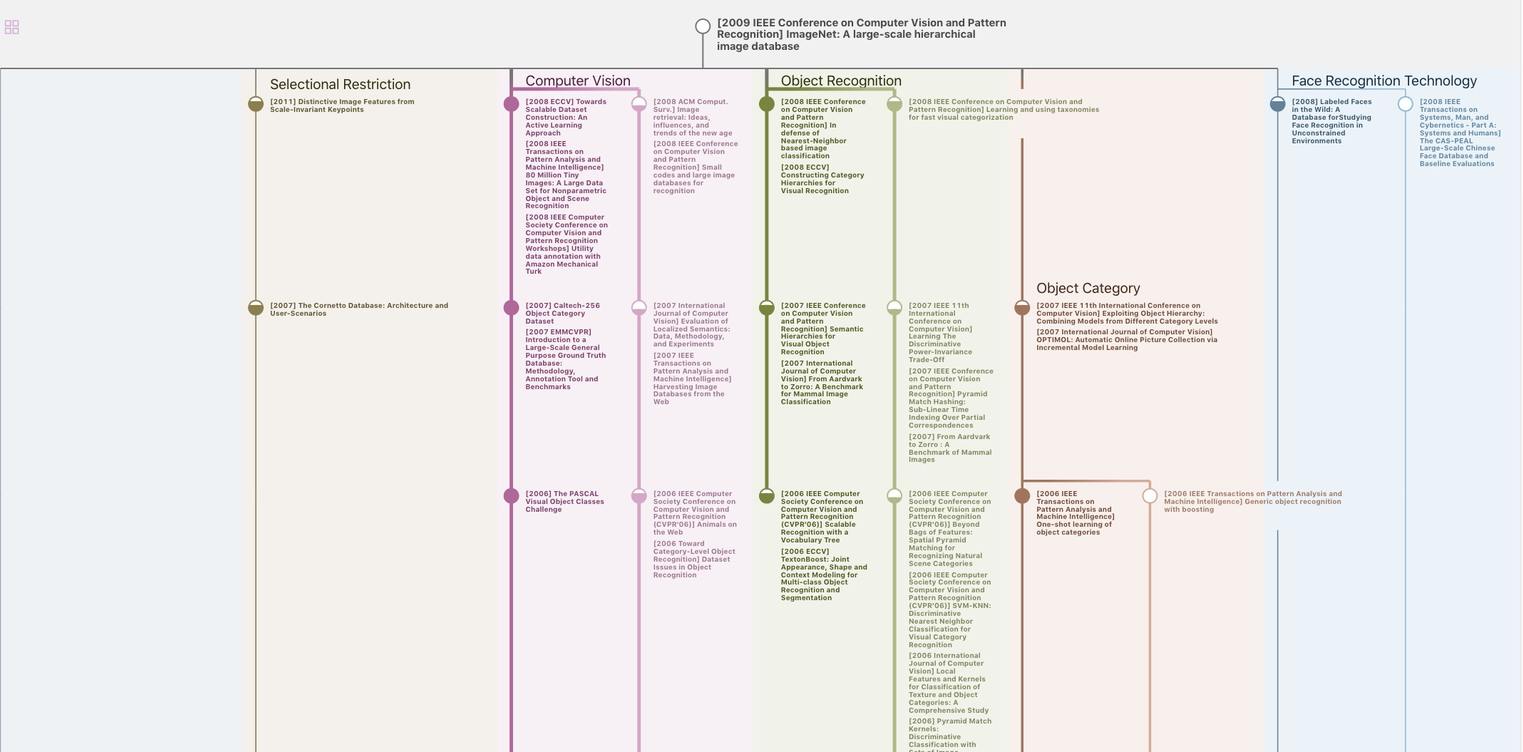
生成溯源树,研究论文发展脉络
Chat Paper
正在生成论文摘要