Boosting dynamic ensemble’s performance in Twitter
Neural Computing and Applications(2019)
摘要
Many text classification problems in social networks, and other contexts, are also dynamic problems, where concepts drift through time, and meaningful labels are dynamic. In Twitter-based applications in particular, ensembles are often applied to problems that fit this description, for example sentiment analysis or adapting to drifting circumstances. While it can be straightforward to request different classifiers' input on such ensembles, our goal is to boost dynamic ensembles by combining performance metrics as efficiently as possible. We present a twofold performance-based framework to classify incoming tweets based on recent tweets. On the one hand, individual ensemble classifiers' performance is paramount in defining their contribution to the ensemble. On the other hand, examples are actively selected based on their ability to effectively contribute to the performance in classifying drifting concepts. The main step of the algorithm uses different performance metrics to determine both each classifier strength in the ensemble and each example importance, and hence lifetime, in the learning process. We demonstrate, on a drifted benchmark dataset, that our framework drives the classification performance considerably up for it to make a difference in a variety of applications.
更多查看译文
关键词
Dynamic ensembles, Text classification, Twitter
AI 理解论文
溯源树
样例
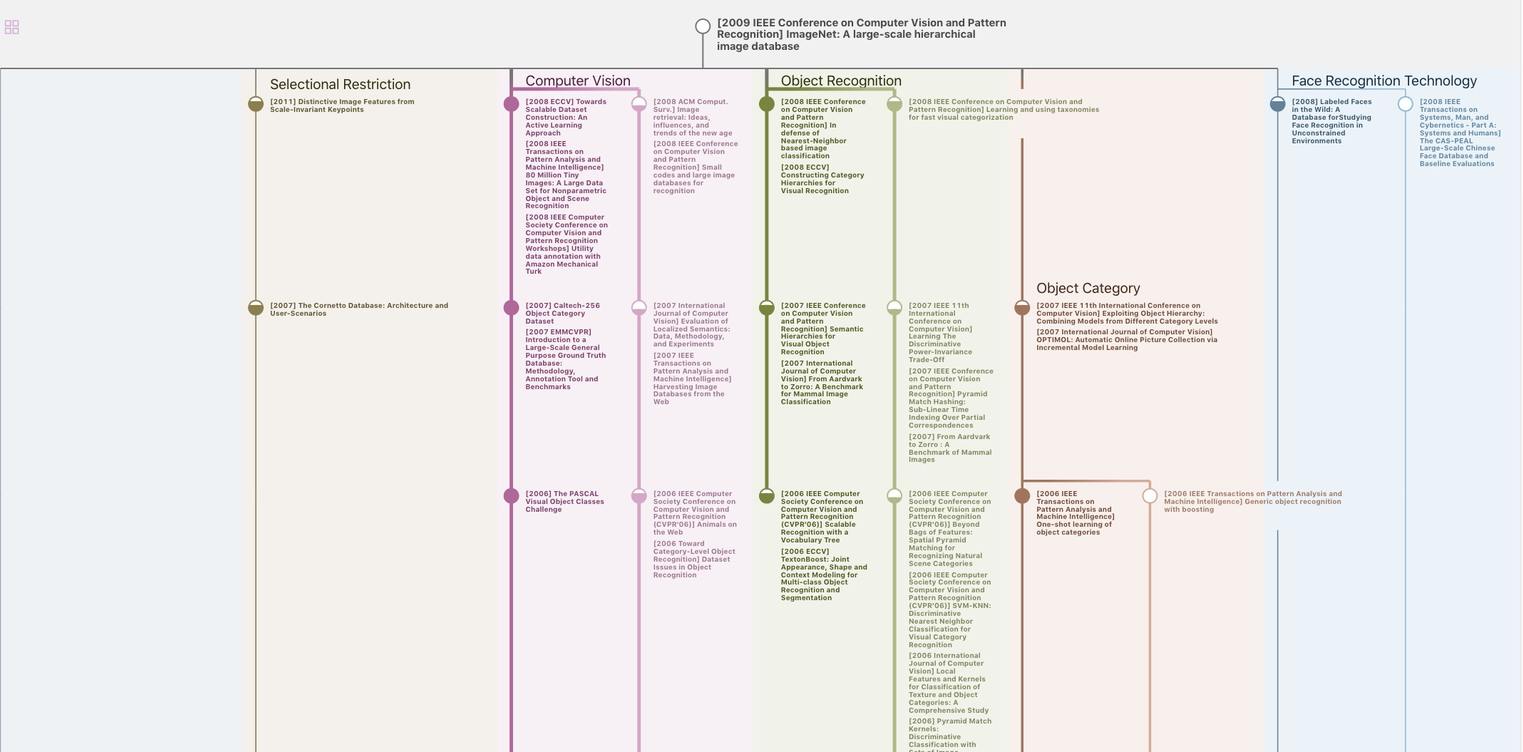
生成溯源树,研究论文发展脉络
Chat Paper
正在生成论文摘要