Quality of service (QoS) parameters prediction for web services using hybrid neural network and ensemble methods
International Journal of System Assurance Engineering and Management(2019)
摘要
Web services are an essential part of the everyday operation for a number of organizations. Applications within organizations frequently depend on web services to fulfill customers needs. Failed and slow online application depending on web services can cost a lot to organizations in terms of customer dissatisfaction. Estimation and improvement of the quality of service (QoS) parameters of web service serve as the primary objective of service providers. We hypothesize that analyzing the source code behind the web services helps in computing the QoS parameters. In this paper, we analyze the source code using thirty-seven different source code metrics (SCMs) to measure the internal structure of the software system. In order to develop a model, these SCMs are treated as input for predicting QoS parameters using nine different prediction techniques and three different ensemble methods. Since SCM act as an important parameter for assessing the performance of prediction techniques, hence for dimensionality reduction and removing irrelevant features, four distinct feature reduction techniques are considered in the study during implementation. Two distinct evaluation criteria such as MMRE and RMSE, are considered for evaluating and comparing the performance of the developed QoS parameters prediction models. The experimental results reveal that the weighted-based ensemble method produces better results. The results also depict that the ME, Ca, IC, DAM, and MFA metrics are commonly found relevant metrics for QoS parameters prediction. Furthermore, we also observe that the selected set of metrics achieves better results compared to all metrics.
更多查看译文
关键词
Web services, Service oriented computing, Ensemble methods, Feature selection technique, Neural network, Gradient descent algorithm, Genetic algorithm, Quality of service (QoS) parameters
AI 理解论文
溯源树
样例
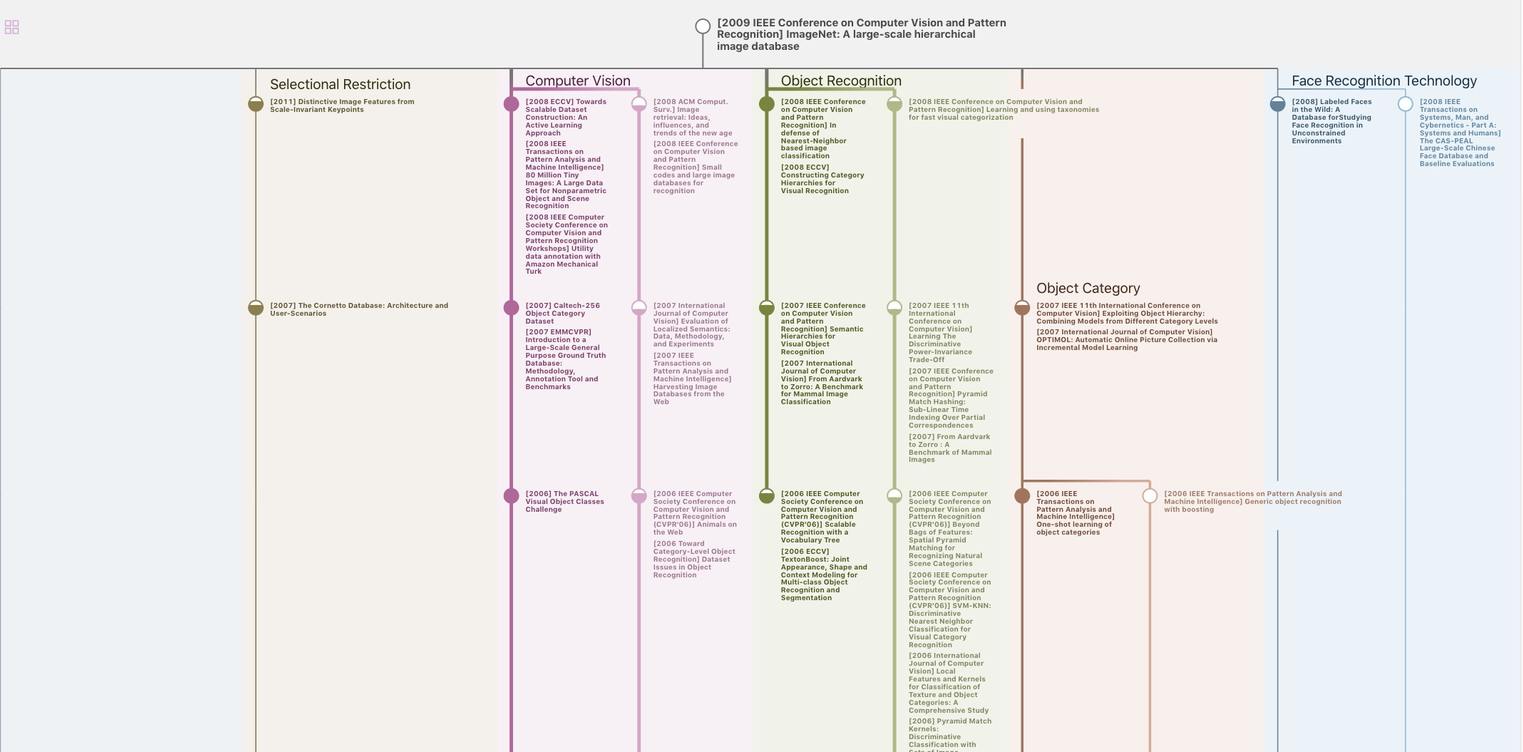
生成溯源树,研究论文发展脉络
Chat Paper
正在生成论文摘要