Monitoring soil organic carbon in alpine soils using in situ vis‐NIR spectroscopy and a multilayer perceptron
LAND DEGRADATION & DEVELOPMENT(2020)
摘要
Soil quality in alpine ecosystems requires regular monitoring to assess its dynamics under changes in climate and land use. Visible near-infrared (vis-NIR) spectroscopy could offer an option, as sampling and transporting large numbers of soil samples in the Qinghai-Tibet Plateau is extremely difficult. However, the potential for in situ vis-NIR spectra and the optimal algorithms need to be defined in this region. We have therefore evaluated the performance of a deep learning method, multilayer perceptron (MLP), for in situ spectral measurement of soil organic carbon (SOC) with in situ vis-NIR spectroscopy in southeastern Tibet, China. A total of 39 soil cores (maximum depth 1 m), including 547 soil samples taken from each 5-cm depth interval, were collected. The spectra were also measured at each 5-cm depth interval accordingly. After spectral preprocessing, 4,096 MLP models were generated by taking all the combinations from six parameters defined in the MLP. The 10-fold-core cross-validation showed that MLP had a good performance for in situ SOC prediction, and the best MLP model had an R-2 of .92, which were much better than those of the partial least squares regression model (R-2 = .80). The results also suggested that the number of epochs, number of neurons, and dropout rate were the most important parameters in the MLP model. We concluded that in situ vis-NIR spectroscopy coupled with an MLP model has high potential for large-scale SOC monitoring in the Qinghai-Tibet Plateau. Our results also provide a reference for rapid hyperparameter optimization using MLP for future soil spectroscopic modeling.
更多查看译文
关键词
deep learning,hyperparameter optimization,proximal soil sensing,Qinghai-Tibet Plateau,soil monitoring
AI 理解论文
溯源树
样例
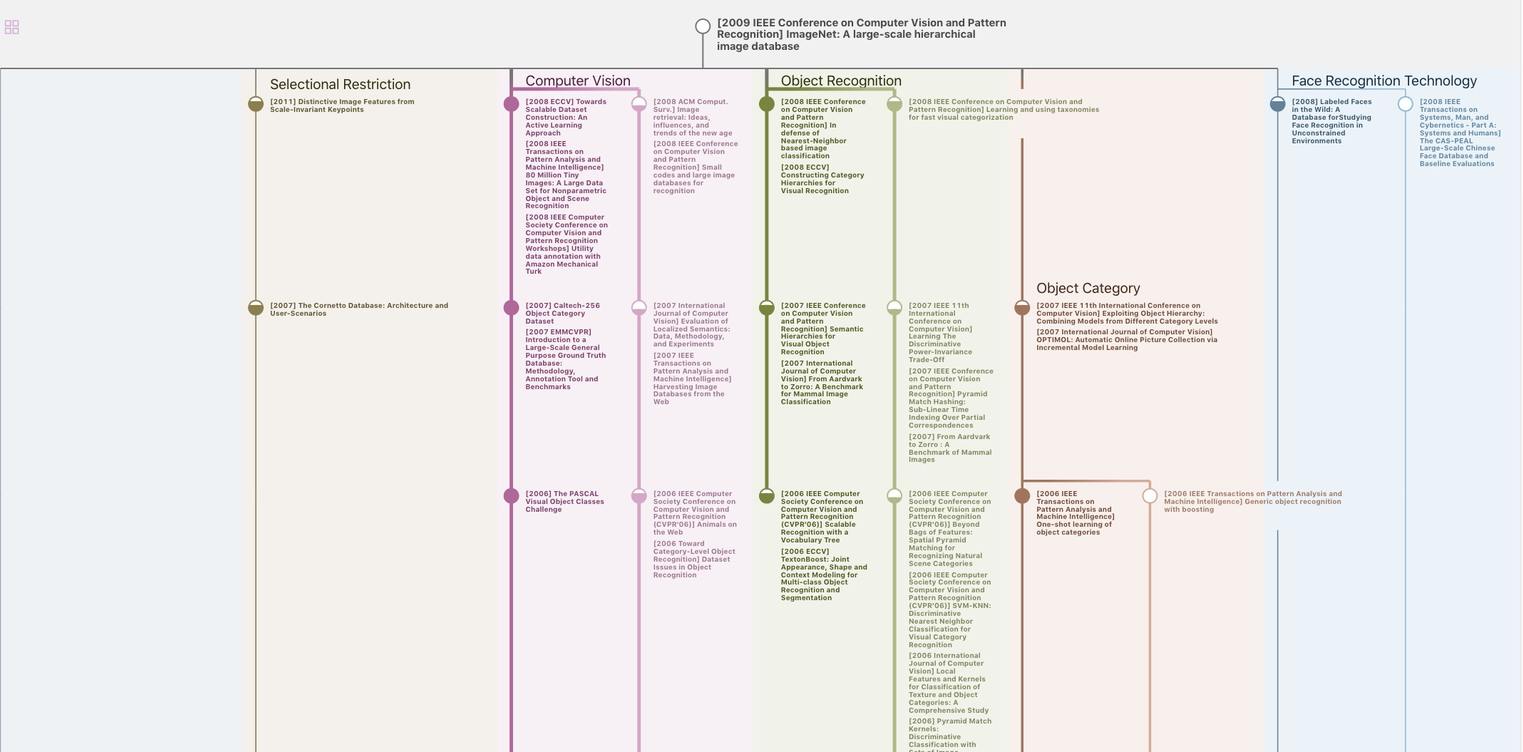
生成溯源树,研究论文发展脉络
Chat Paper
正在生成论文摘要