Visual Odometry with Deep Bidirectional Recurrent Neural Networks.
PRCV (3)(2019)
摘要
We propose a novel architecture for learning camera poses from image sequences with an extended 2D LSTM (Long Short-Term Memory). Unlike most of the previous deep learning based VO (Visual Odometry) methods, our model predicts the pose per frame with temporal information from image sequences by adopting a forward-backward process. In addition, we use 3D tensors as basic structures to generate spatial information. The network learns poses in a bottom-up manner by coupling local and global constraints. Experiments demonstrate that on the public KITTI benchmark dataset, our architecture outperforms the state-of-the-art end-to-end methods in term of camera motion prediction and is comparable with model-based methods. The network generalizes well on the Málaga dataset without extra training or fine-tuning.
更多查看译文
关键词
Visual Odometry, Motion estimation, Convolutional Neural Networks, Recurrent Neural Network
AI 理解论文
溯源树
样例
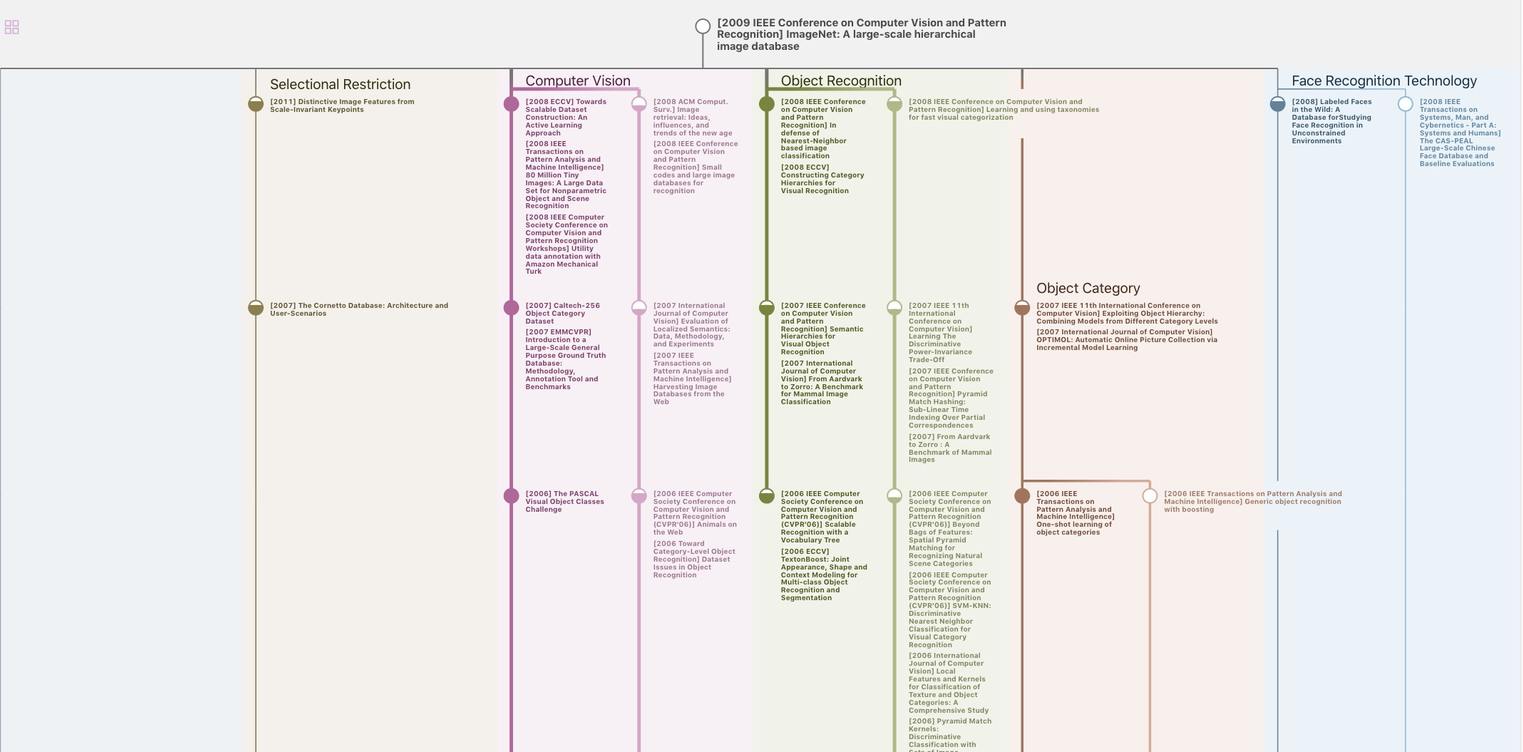
生成溯源树,研究论文发展脉络
Chat Paper
正在生成论文摘要