Deep Head Pose Estimation Using Synthetic Images And Partial Adversarial Domain Adaption For Continuous Label Spaces
2019 IEEE/CVF INTERNATIONAL CONFERENCE ON COMPUTER VISION (ICCV 2019)(2019)
摘要
Head pose estimation aims at predicting an accurate pose from an image. Current approaches rely on supervised deep learning, which typically requires large amounts of labeled data. Manual or sensor-based annotations of head poses are prone to errors. A solution is to generate synthetic training data by rendering 3D face models. However, the differences (domain gap) between rendered (source-domain) and real-world (target-domain) images can cause low performance. Advances in visual domain adaptation allow reducing the influence of domain differences using adversarial neural networks, which match the feature spaces between domains by enforcing domain-invariant features. While previous work on visual domain adaptation generally assumes discrete and shared label spaces, these assumptions are both invalid for pose estimation tasks. We are the first to present domain adaptation for head pose estimation with a focus on partially shared and continuous label spaces. More precisely, we adapt the predominant weighting approaches to continuous label spaces by applying a weighted resampling of the source domain during training. To evaluate our approach, we revise and extend existing datasets resulting in a new benchmark for visual domain adaption. Our experiments show that our method improves the accuracy of head pose estimation for real-world images despite using only labels from synthetic images.
更多查看译文
关键词
deep head pose estimation,synthetic images,partial adversarial domain adaption,continuous label spaces,supervised deep learning,head poses,synthetic training data,rendering 3D face models,visual domain adaptation,domain differences,domain-invariant features,shared label spaces,source domain,visual domain adaption
AI 理解论文
溯源树
样例
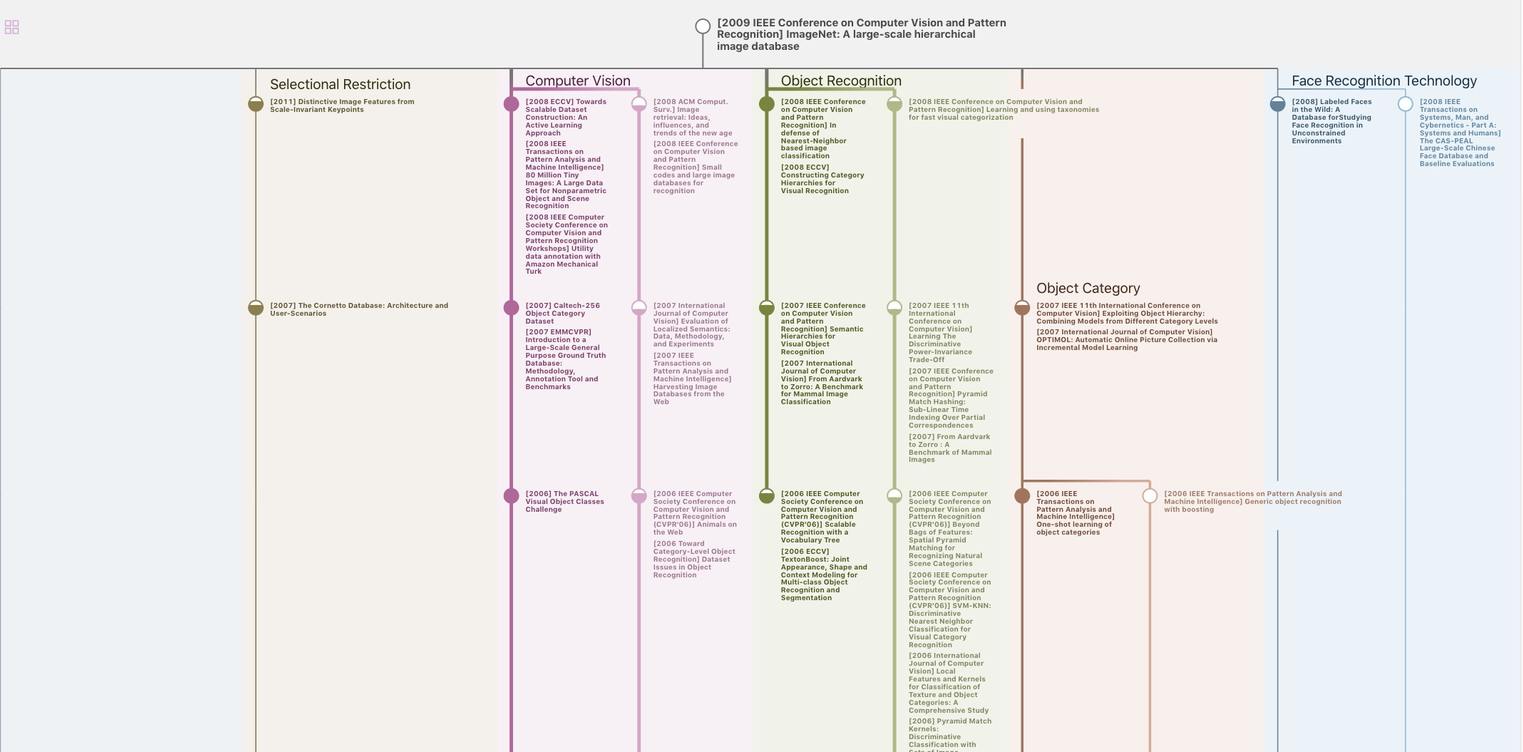
生成溯源树,研究论文发展脉络
Chat Paper
正在生成论文摘要