Regionalization Of Anthropogenically Forced Changes In 3hourly Extreme Precipitation Over Europe
ENVIRONMENTAL RESEARCH LETTERS(2019)
摘要
Future extreme precipitation events are expected to be influenced by climate change; however, the robustness of this anthropogenically forced response in respect to projection uncertainty especially for sub-daily extremes is not fully understood. We analyze the impact of anthropogenic climate change on 3 hourly extreme precipitation with return periods ranging between 5 and 50 years over Europe using the RCA4 model ensemble simulations at 0.11 resolution. The robustness of the signals is examined based on a regionalized signal-to-noise (S2N) technique by taking the spatial pooling into account and the efficacy of the regionalization is tested by a sensitivity analysis. The results show an increasing signal in 3 hourly extreme precipitation over Europe for all seasons except summer for which a bipolar pattern (increase in the north and decrease in the south) is discerned. For the business as -usual scenario RCP8.5, the regionalized winter 3 hourly extreme precipitation signals over 9 x 9 model grid cells are statistically significant in roughly 72%, 65%, 59% and 48% of the European area for 5, 15, 25 and 50 year return periods respectively, while 16%-21% of the area will experience significant changes in summer. The S2N values for 3 hourly extreme precipitation changes rise after the spatial pooling by about a factor of 1.4-1.7 for all seasons except summer when they decline by about a factor of 0.78. The results of sensitivity analysis reveal that the regionalization influence is sensitive in order of decreasing importance to season, precipitation time scale, precipitation intensity, emission scenario and model spatial resolution. The precipitation time scale is particularly important seasonally in summer and regionally in south Europe when/where short-duration convective precipitation is dominant.
更多查看译文
关键词
sub-daily precipitation extremes, EURO-CORDEX, signal robustness, internal variability, spatial pooling, climate change
AI 理解论文
溯源树
样例
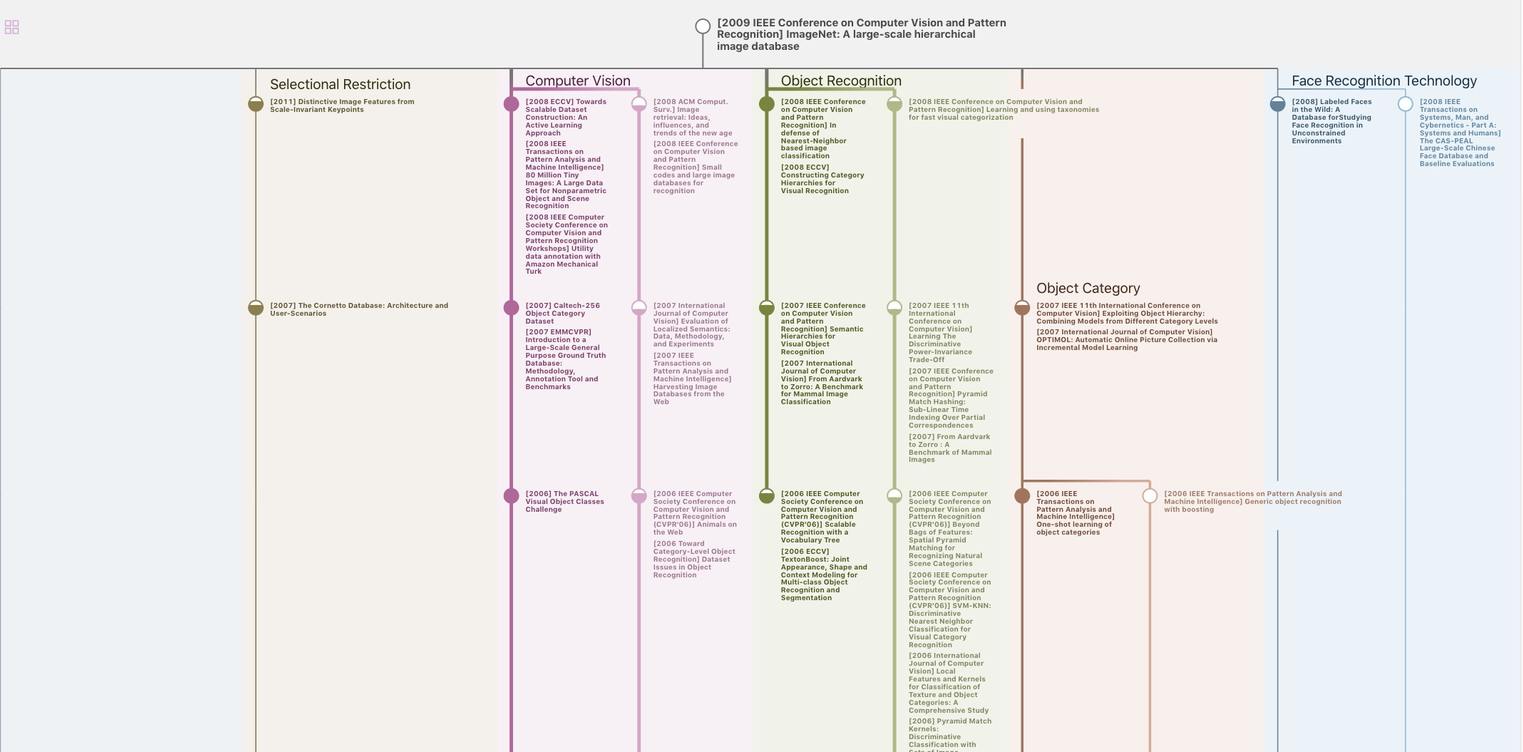
生成溯源树,研究论文发展脉络
Chat Paper
正在生成论文摘要