ADVERSE EFFECTS OF IMAGE TILING FOR AUTOMATIC DEEP LEARNING GLIOMA SEGMENTATION IN MRI
Neuro-Oncology(2019)
摘要
Abstract BACKGROUND Application of deep learning to neuro-oncology has shown promising clinically relevant results for tumor classification, localization, and segmentation. Hardware limitations, typically memory size of graphics cards, prevent magnetic resonance imaging (MRI) volumes from being processed as a whole, and hence they are divided into smaller, overlapping tiles. Deep learning algorithms (e.g., U-Net) can then be trained and applied for predictions on such tiles, followed by their combination/stitching as the final prediction for the whole volume. We investigate the hypothesis that image tiling options, such as tile placing, size, overlap, and stitching, introduce variations with adverse effects on predictions, both in terms of inconsistency and accuracy. METHODS We utilized the publicly available BraTS 2018 dataset of 285 baseline pre-operative MRI glioma scans, with corresponding expert tumor boundary annotations. We implemented a 3D U-Net to predict boundaries of the whole tumor extent, by virtue of the abnormal hyper-intense signal of T2-FLAIR scans. RESULTS Simply flipping the tile horizontally, or translating it by one voxel, produces different predictions. Use of small tiles (64x64x64 voxels) yields substantially more false positive predictions than when using larger tile size (i.e., 128x128x128 voxels). Overlapping tiles produce conflicting predictions, leading to ambiguous interpretations upon their stitching. In areas of overlapping tiles, rounding followed by averaging the overlapping predictions produce superior results to the inverse sequence. All these are particularly noticeable in the margins of the abnormal signal and in areas of large contrast variation. CONCLUSIONS Although tiling is a workaround for hardware limitations, it introduces variations detrimental to accuracy. Tiling of neuro-oncology scans for computational analysis using deep learning leads to non-generalizable, non-reproducible results, thereby affecting the performance and potential clinical translatability of such algorithms. Careful considerations and standardization recommendations should be established and appropriately documented for performing such analyses, in order to avoid misinterpretation of results.
更多查看译文
AI 理解论文
溯源树
样例
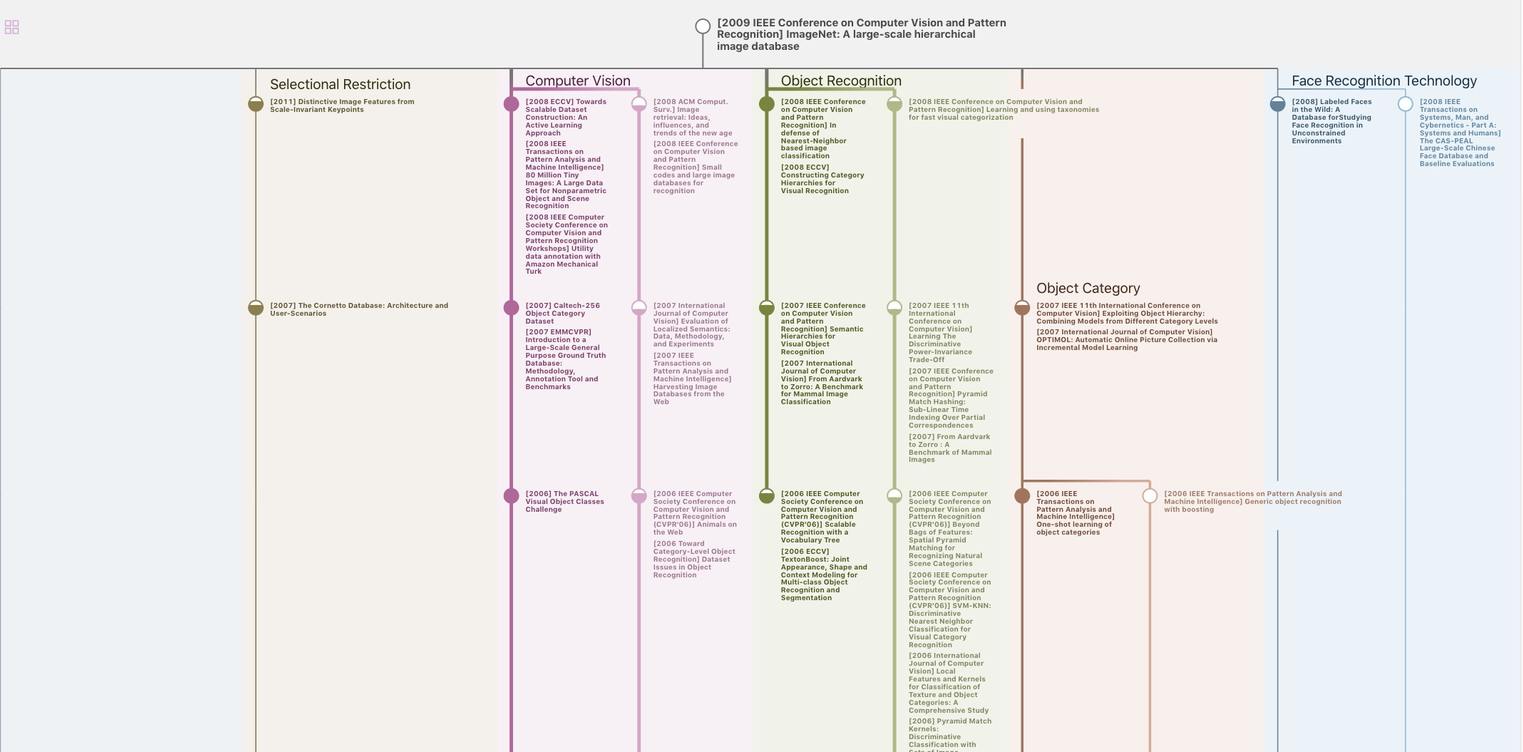
生成溯源树,研究论文发展脉络
Chat Paper
正在生成论文摘要