Deep Multi-Model Fusion For Single-Image Dehazing
2019 IEEE/CVF INTERNATIONAL CONFERENCE ON COMPUTER VISION (ICCV 2019)(2019)
摘要
This paper presents a deep multi-model fusion network to attentively integrate multiple models to separate layers and boost the performance in single-image dehazing. To do so, we first formulate the attentional feature integration module to maximize the integration of the convolutional neural network (CNN) features at different CNN layers and generate the attentional multi-level integrated features (AMLIF). Then, from the AMLIF, we further predict a haze-free result for an atmospheric scattering model, as well as for four haze-layer separation models, then fuse the results together to produce the final haze-free image. To evaluate the effectiveness of our method, we compare our network with several state-of-the-art methods on two widely-used dehazing benchmark datasets, as well as on two sets of real-world hazy images. Experimental results demonstrate clear quantitative and qualitative improvements of our method over the state-of-the-arts.
更多查看译文
关键词
convolutional neural network,different CNN layers,multilevel integrated features,AMLIF,haze-free result,atmospheric scattering model,haze-layer separation models,final haze-free image,real-world hazy images,single-image dehazing,deep multimodel fusion network,attentional feature integration module
AI 理解论文
溯源树
样例
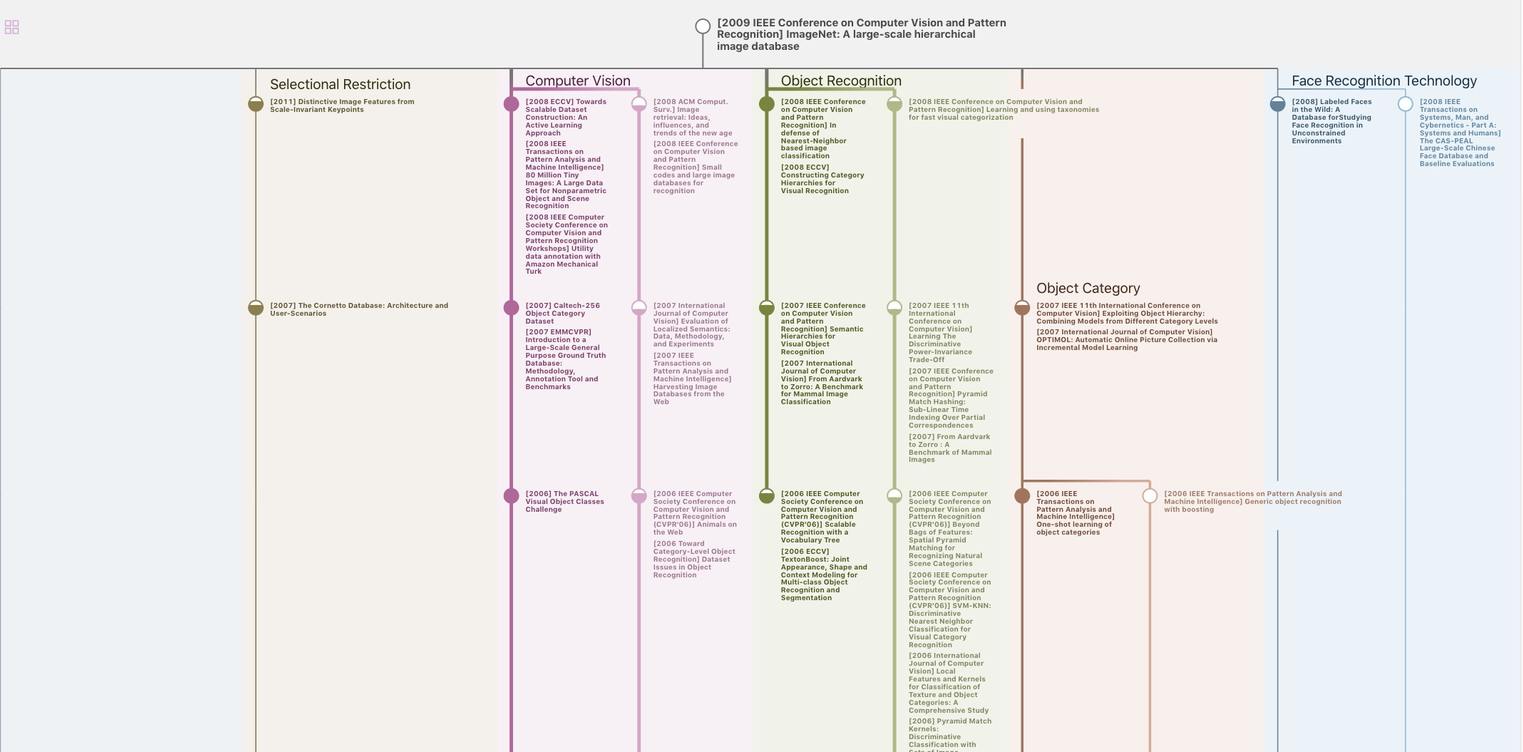
生成溯源树,研究论文发展脉络
Chat Paper
正在生成论文摘要