Bayesian inference of non-linear multiscale model parameters accelerated by a Deep Neural Network
Computer Methods in Applied Mechanics and Engineering(2020)
摘要
We develop a Bayesian Inference (BI) of the parameters of a non-linear multiscale model and of its material constitutive laws using experimental composite coupon tests as observation data. In particular we consider non-aligned Short Fibers Reinforced Polymer (SFRP) as a composite material system and Mean-Field Homogenization (MFH) as a multiscale model. Although MFH is computationally efficient, when considering non-aligned inclusions, the evaluation cost of a non-linear response for a given set of model and material parameters remains too prohibitive to be coupled with the sampling process required by the BI. Therefore, a Neural-Network (NNW) is first trained using the MFH model, and is then used as a surrogate model during the BI process, making the identification process affordable.
更多查看译文
关键词
Multiscale,Composites,Bayesian inference,Neural Network,Non-linear
AI 理解论文
溯源树
样例
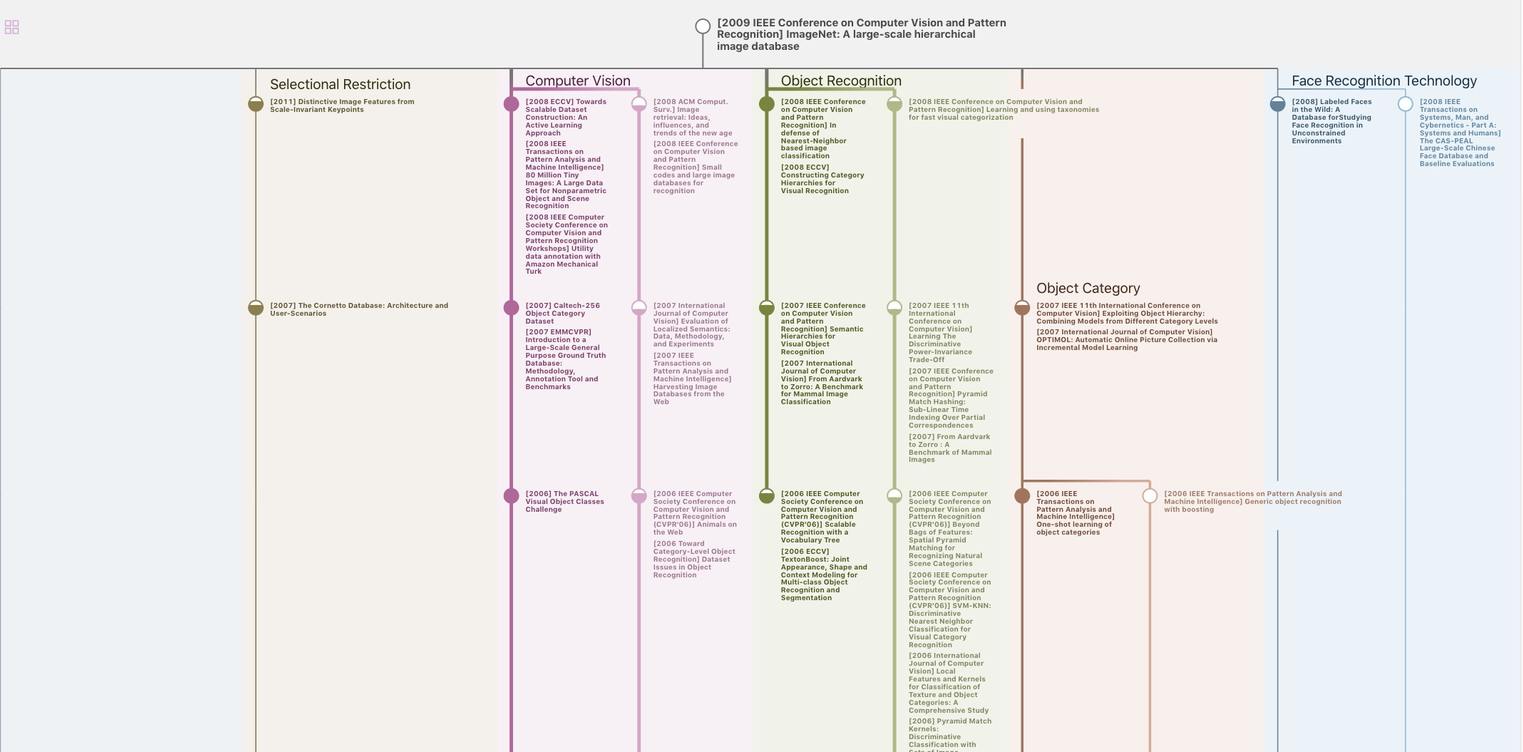
生成溯源树,研究论文发展脉络
Chat Paper
正在生成论文摘要