Efficient Modelling of Presence-Only Species Data via Local Background Sampling
Journal of Agricultural, Biological and Environmental Statistics(2019)
摘要
In species distribution modelling, records of species presence are often modelled as a realization of a spatial point process whose intensity is a function of environmental covariates. One way to fit a spatial point process model is to apply logistic regression to an artificial case–control sample consisting of the observed presence records combined with a simulated pattern of background points, usually a uniform random sample from within the study’s spatial domain. In this paper we propose local background sampling as an alternative to uniform background sampling when using logistic regression to fit spatial point process models to data. Our method is similar to the local case–control sampling procedure of Fithian and Hastie (Ann Appl Stat 42:1693–1724, 2014), but differs in that background points are sampled with probability proportional to an initial intensity estimate based on a pilot point process model. We compare local background sampling with uniform background sampling in a simulation study and in an example modelling the distributions of bumble bees (genus Bombus ) in Ontario, Canada. Our results show local background sampling to be more efficient than uniform background sampling in all simulated settings and across all species analysed. Supplementary materials accompanying this paper appear online.
更多查看译文
关键词
Case–control sampling, Logistic regression, Spatial point processes, Species distribution modelling
AI 理解论文
溯源树
样例
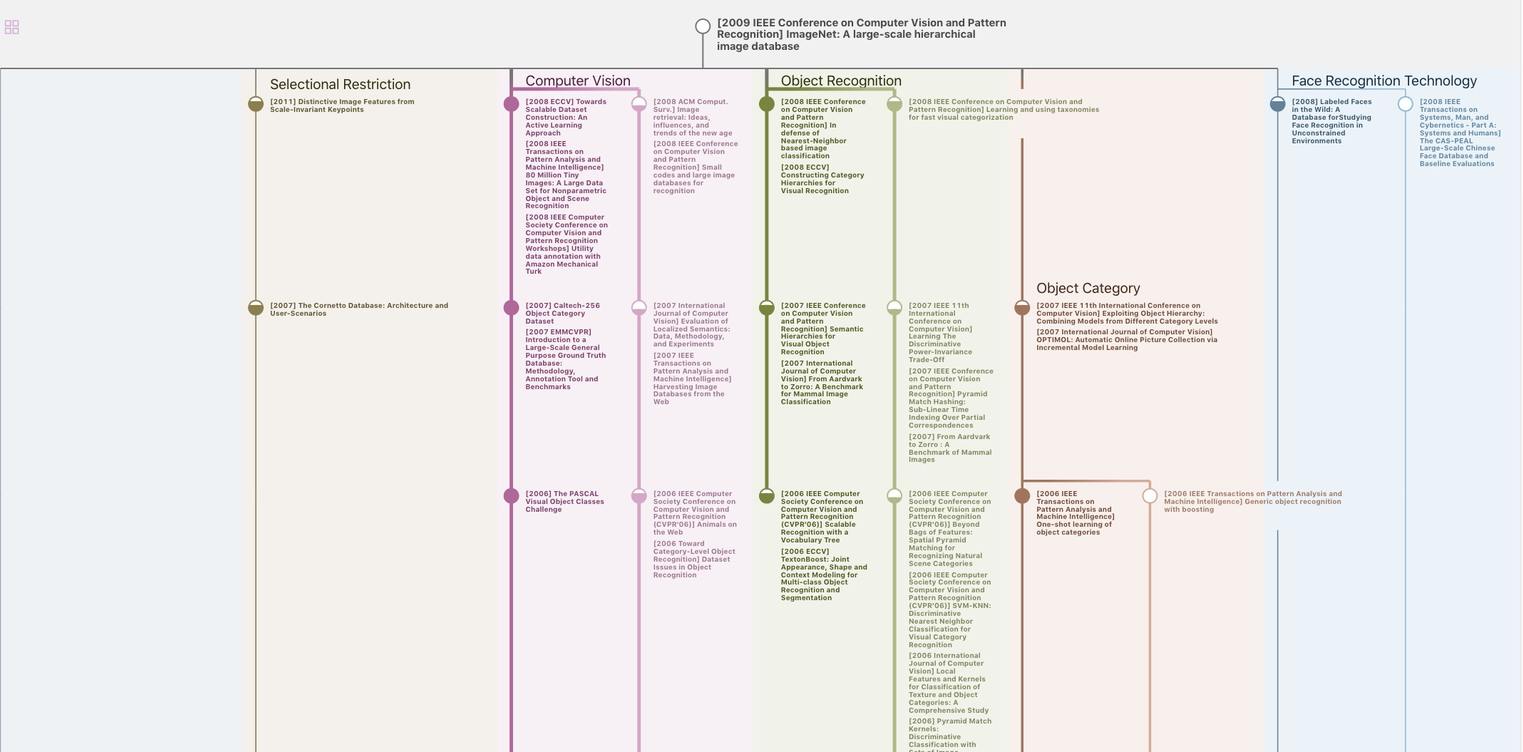
生成溯源树,研究论文发展脉络
Chat Paper
正在生成论文摘要