LIBS spectral feature selection to enhance classification capabilities: A t-test filter approach
Spectrochimica Acta Part B: Atomic Spectroscopy(2019)
摘要
Extracting classification-relevant information from complex spectral data usually takes extensive expert knowledge and a lot of time and effort. The main challenge remains processing, in a practically feasible manner, huge amounts of highly variable data to recognize patterns useful to explain away classifications. In this paper we present a statistical hypothesis testing approach to select, with minimum knowledge regarding the spectral matrix, the LIBS spectral features potentially most useful to feed supervised classification algorithms. In probing the capabilities of our approach, starting from raw spectral data, a bio-LIBS application was used in an attempt to uncover the chemistry behind the severity diagnosis of liver fibrosis. By coupling our approach with Kernel Density Estimation and Gradient Boosting as normalizing strategy and classification algorithm, respectively, we managed to correctly classify 84% of the spectra, 91% of spots and 95% of samples with error rates comparable to computationally costlier learning-methods such as Random Forest. A definite advantage of the proposed approach is that it is highly scalable, parallelizable, and susceptible to carry-out in a piecemeal fashion.
更多查看译文
关键词
Feature selection,Filter methods,Supervised classification,Machine learning methods,Laser-induced breakdown spectroscopy
AI 理解论文
溯源树
样例
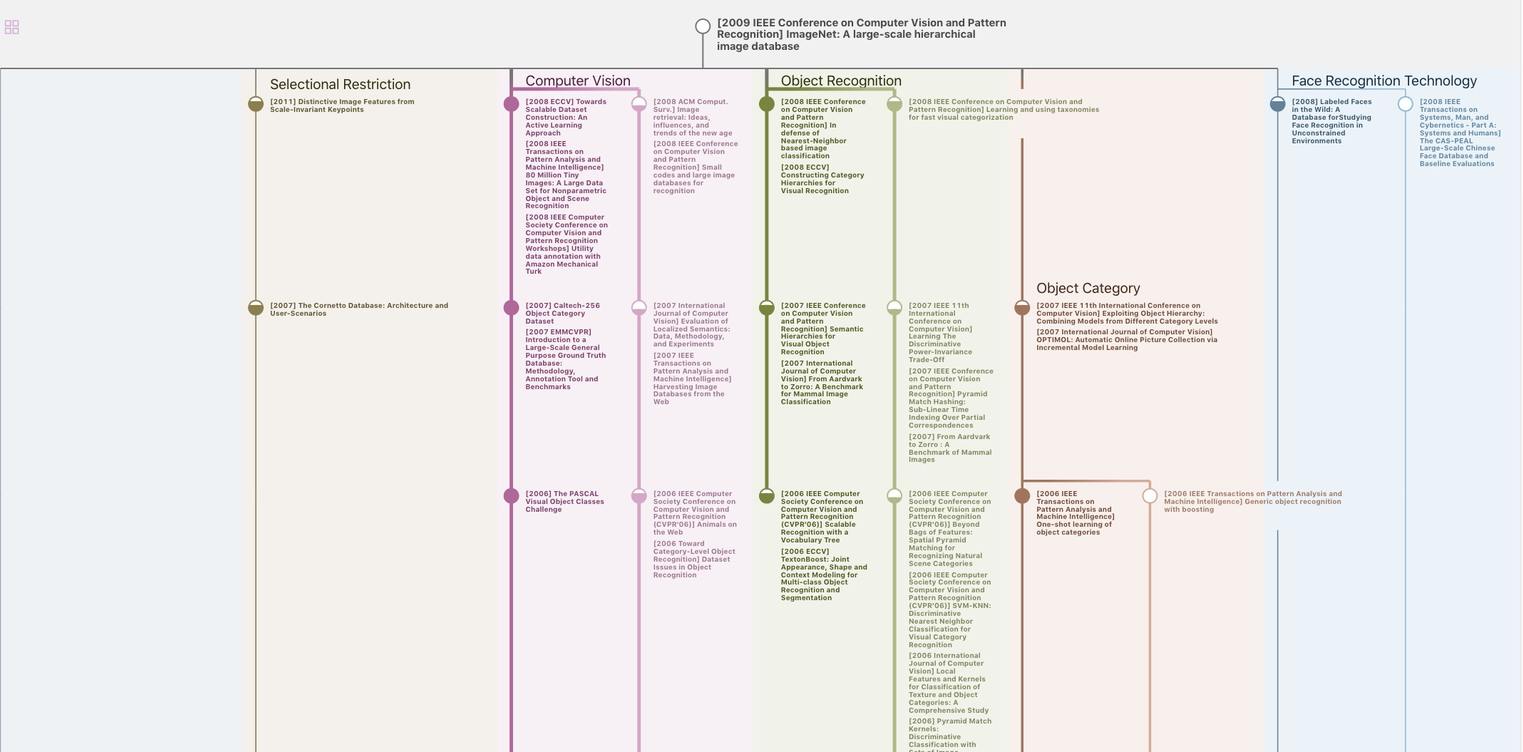
生成溯源树,研究论文发展脉络
Chat Paper
正在生成论文摘要