Hyperspectral Anomaly Detection With Kernel Isolation Forest
IEEE Transactions on Geoscience and Remote Sensing(2020)
摘要
In this article, a novel hyperspectral anomaly detection method with kernel Isolation Forest (iForest) is proposed. The method is based on an assumption that anomalies rather than background can be more susceptible to isolation in the kernel space. Based on this idea, the proposed method detects anomalies as follows. First, the hyperspectral data are mapped into the kernel space, and the first
$K$
principal components are used. Then, the isolation samples in the image are detected with the iForest constructed using randomly selected samples in the principal components. Finally, the initial anomaly detection map is iteratively refined with locally constructed iForest in connected regions with large areas. Experimental results on several real hyperspectral data sets demonstrate that the proposed method outperforms other state-of-the-art methods.
更多查看译文
关键词
Kernel,Anomaly detection,Hyperspectral imaging,Detectors,Vegetation,Forestry
AI 理解论文
溯源树
样例
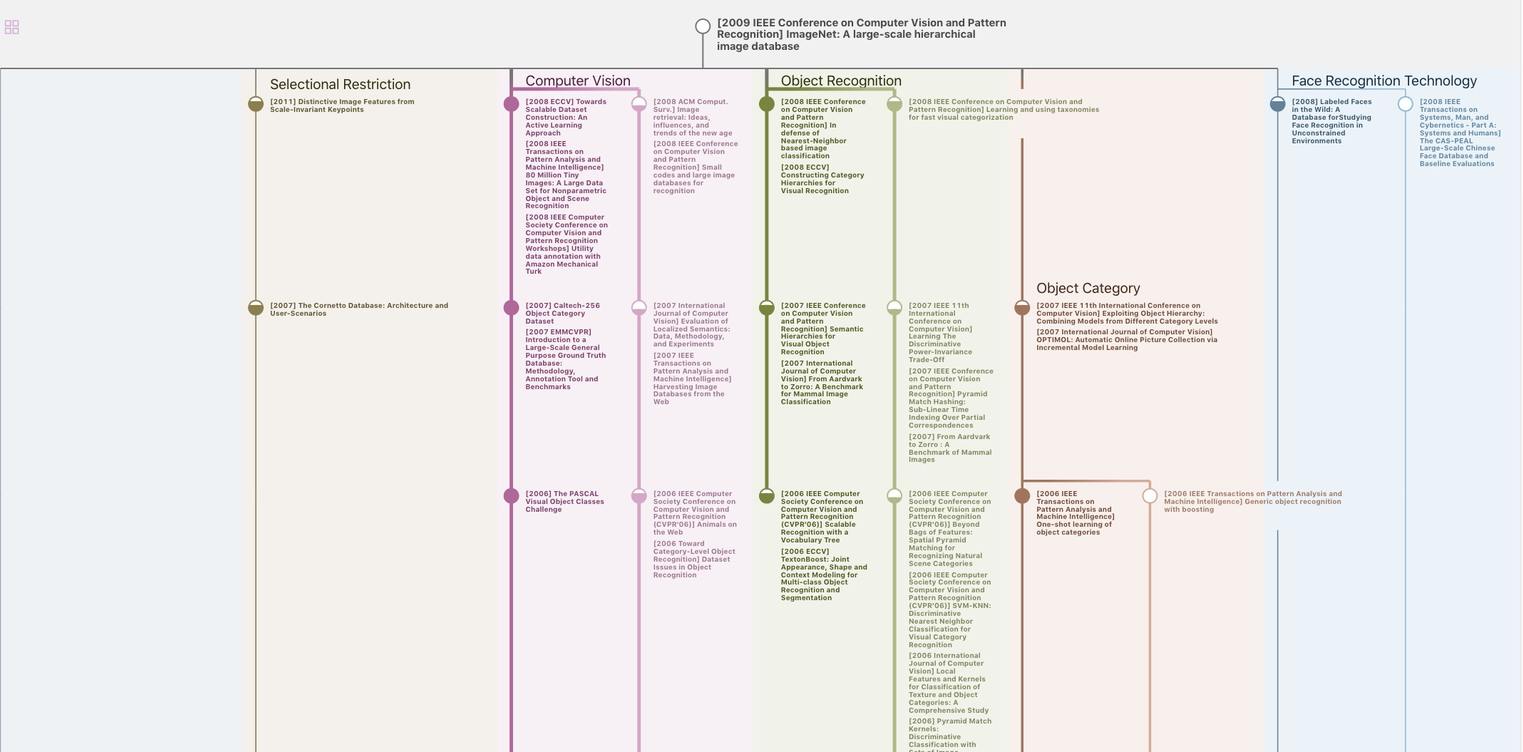
生成溯源树,研究论文发展脉络
Chat Paper
正在生成论文摘要