SRSC: Selective, Robust, and Supervised Constrained Feature Representation for Image Classification
IEEE Transactions on Neural Networks and Learning Systems(2020)
摘要
Feature representation learning, an emerging topic in recent years, has achieved great progress. Powerful learned features can lead to excellent classification accuracy. In this article, a selective and robust feature representation framework with a supervised constraint (SRSC) is presented. SRSC seeks a selective, robust, and discriminative subspace by transforming the original feature space into the category space. Particularly, we add a selective constraint to the transformation matrix (or classifier parameter) that can select discriminative dimensions of the input samples. Moreover, a supervised regularization is tailored to further enhance the discriminability of the subspace. To relax the hard zero-one label matrix in the category space, an additional error term is also incorporated into the framework, which can lead to a more robust transformation matrix. SRSC is formulated as a constrained least square learning (feature transforming) problem. For the SRSC problem, an inexact augmented Lagrange multiplier method (ALM) is utilized to solve it. Extensive experiments on several benchmark data sets adequately demonstrate the effectiveness and superiority of the proposed method. The proposed SRSC approach has achieved better performances than the compared counterpart methods.
更多查看译文
关键词
Training,Task analysis,Learning systems,Computational modeling,Optimization,Support vector machines,Principal component analysis
AI 理解论文
溯源树
样例
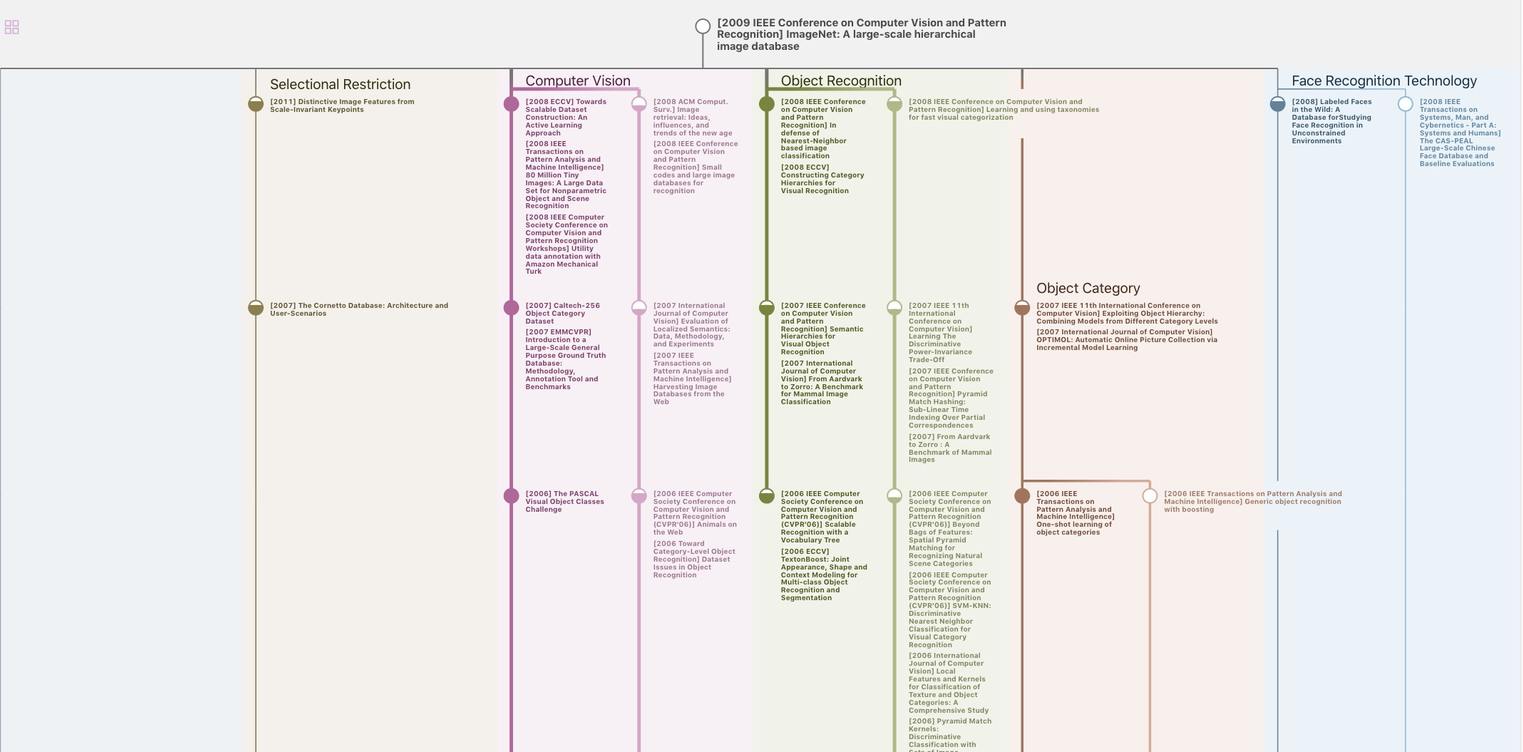
生成溯源树,研究论文发展脉络
Chat Paper
正在生成论文摘要