Parseval Proximal Neural Networks
Journal of Fourier Analysis and Applications(2020)
摘要
The aim of this paper is twofold. First, we show that a certain concatenation of a proximity operator with an affine operator is again a proximity operator on a suitable Hilbert space. Second, we use our findings to establish so-called proximal neural networks (PNNs) and stable tight frame proximal neural networks. Let ℋ and 𝒦 be real Hilbert spaces, b ∈𝒦 and T ∈ℬ (ℋ,𝒦) a linear operator with closed range and Moore–Penrose inverse T^† . Based on the well-known characterization of proximity operators by Moreau, we prove that for any proximity operator Prox:𝒦→𝒦 the operator T^† Prox( T · + b) is a proximity operator on ℋ equipped with a suitable norm. In particular, it follows for the frequently applied soft shrinkage operator Prox= S_λ:ℓ _2 →ℓ _2 and any frame analysis operator T:ℋ→ℓ _2 that the frame shrinkage operator T^† S_λ T is a proximity operator on a suitable Hilbert space. The concatenation of proximity operators on ℝ^d equipped with different norms establishes a PNN. If the network arises from tight frame analysis or synthesis operators, then it forms an averaged operator. In particular, it has Lipschitz constant 1 and belongs to the class of so-called Lipschitz networks, which were recently applied to defend against adversarial attacks. Moreover, due to its averaging property, PNNs can be used within so-called Plug-and-Play algorithms with convergence guarantee. In case of Parseval frames, we call the networks Parseval proximal neural networks (PPNNs). Then, the involved linear operators are in a Stiefel manifold and corresponding minimization methods can be applied for training of such networks. Finally, some proof-of-the concept examples demonstrate the performance of PPNNs.
更多查看译文
关键词
Lipschitz neural networks, Averaged operators, Proximal operators, Frame shrinkage, Adverserial robustness, Optimization on Stiefel manifolds, 68T07, 90C26
AI 理解论文
溯源树
样例
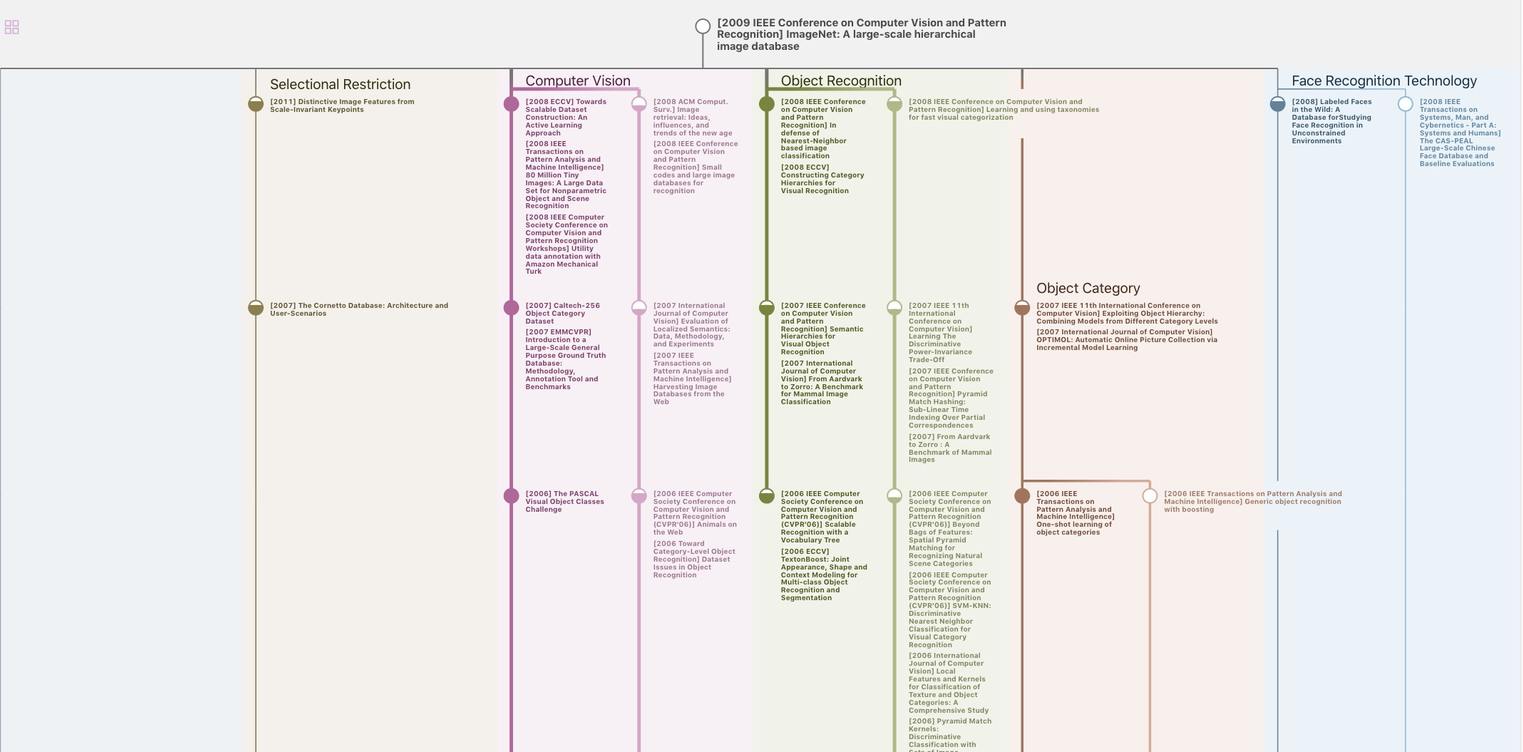
生成溯源树,研究论文发展脉络
Chat Paper
正在生成论文摘要