Online Reinforcement Learning of Optimal Threshold Policies for Markov Decision Processes
IEEE Transactions on Automatic Control(2022)
摘要
To overcome the
curses of dimensionality and modeling
of dynamic programming methods to solve Markov decision process problems, reinforcement learning (RL) methods are adopted in practice. Contrary to traditional RL algorithms, which do not consider the structural properties of the optimal policy, we propose a structure-aware learning algorithm to exploit the ordered multithreshold structure of the optimal policy, if any. We prove the asymptotic convergence of the proposed algorithm to the optimal policy. Due to the reduction in the policy space, the proposed algorithm provides remarkable improvements in storage and computational complexities over classical RL algorithms. Simulation results establish that the proposed algorithm converges faster than other RL algorithms.
更多查看译文
关键词
Markov decision process (MDP),online learning of threshold policies,reinforcement learning (RL),stochastic approximation (SA) algorithms,stochastic control
AI 理解论文
溯源树
样例
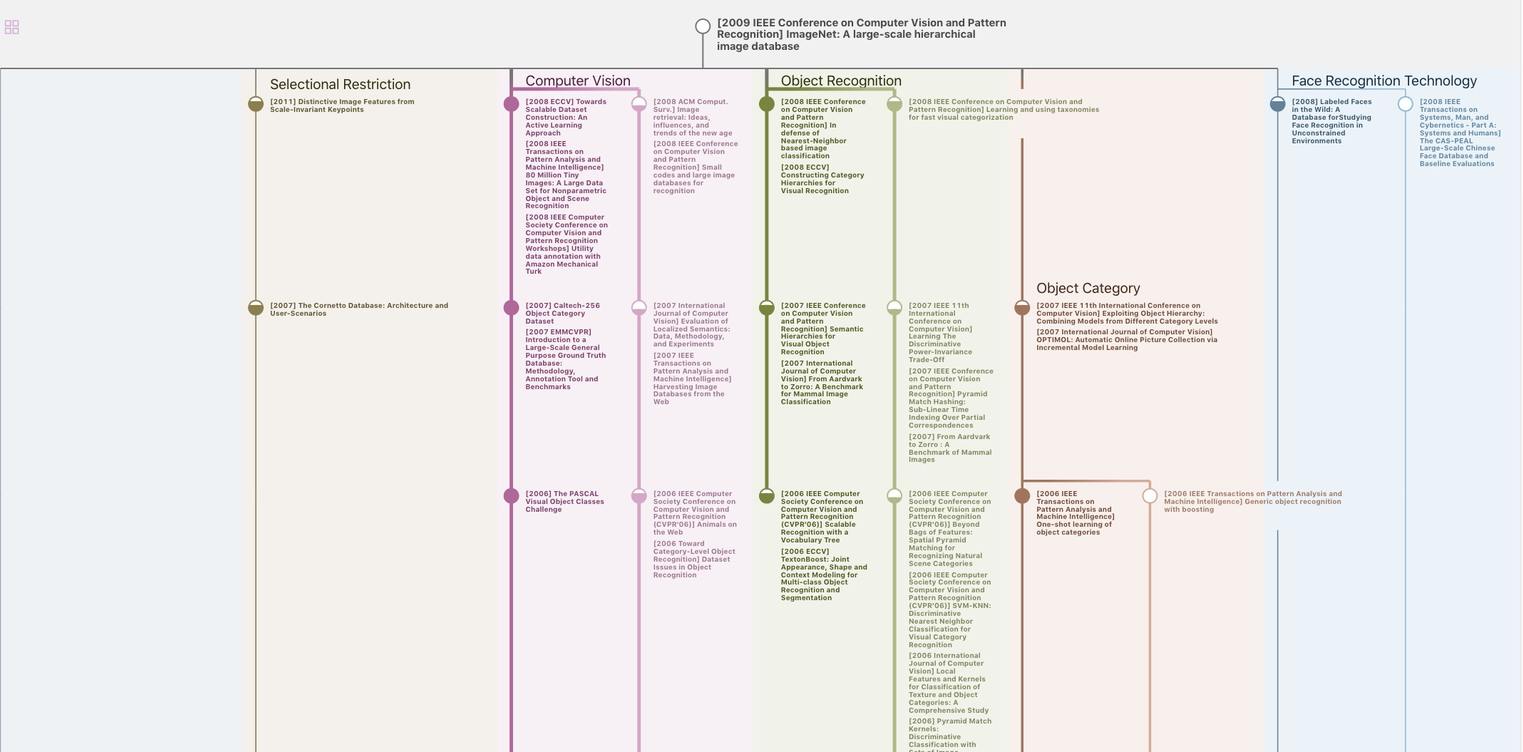
生成溯源树,研究论文发展脉络
Chat Paper
正在生成论文摘要