DeepSFM: Structure from Motion via Deep Bundle Adjustment
European Conference on Computer Vision(2020)
摘要
Structure from motion (SfM) is an essential computer vision problem which has not been well handled by deep learning. One of the promising trends is to apply explicit structural constraint, e.g. 3D cost volume, into the network. However, existing methods usually assume accurate camera poses either from GT or other methods, which is unrealistic in practice. In this work, we design a physical driven architecture, namely DeepSFM, inspired by traditional Bundle Adjustment (BA), which consists of two cost volume based architectures for depth and pose estimation respectively, iteratively running to improve both. The explicit constraints on both depth (structure) and pose (motion), when combined with the learning components, bring the merit from both traditional BA and emerging deep learning technology. Extensive experiments on various datasets show that our model achieves the state-of-the-art performance on both depth and pose estimation with superior robustness against less number of inputs and the noise in initialization.
更多查看译文
关键词
deepsfm bundle adjustment,motion,structure
AI 理解论文
溯源树
样例
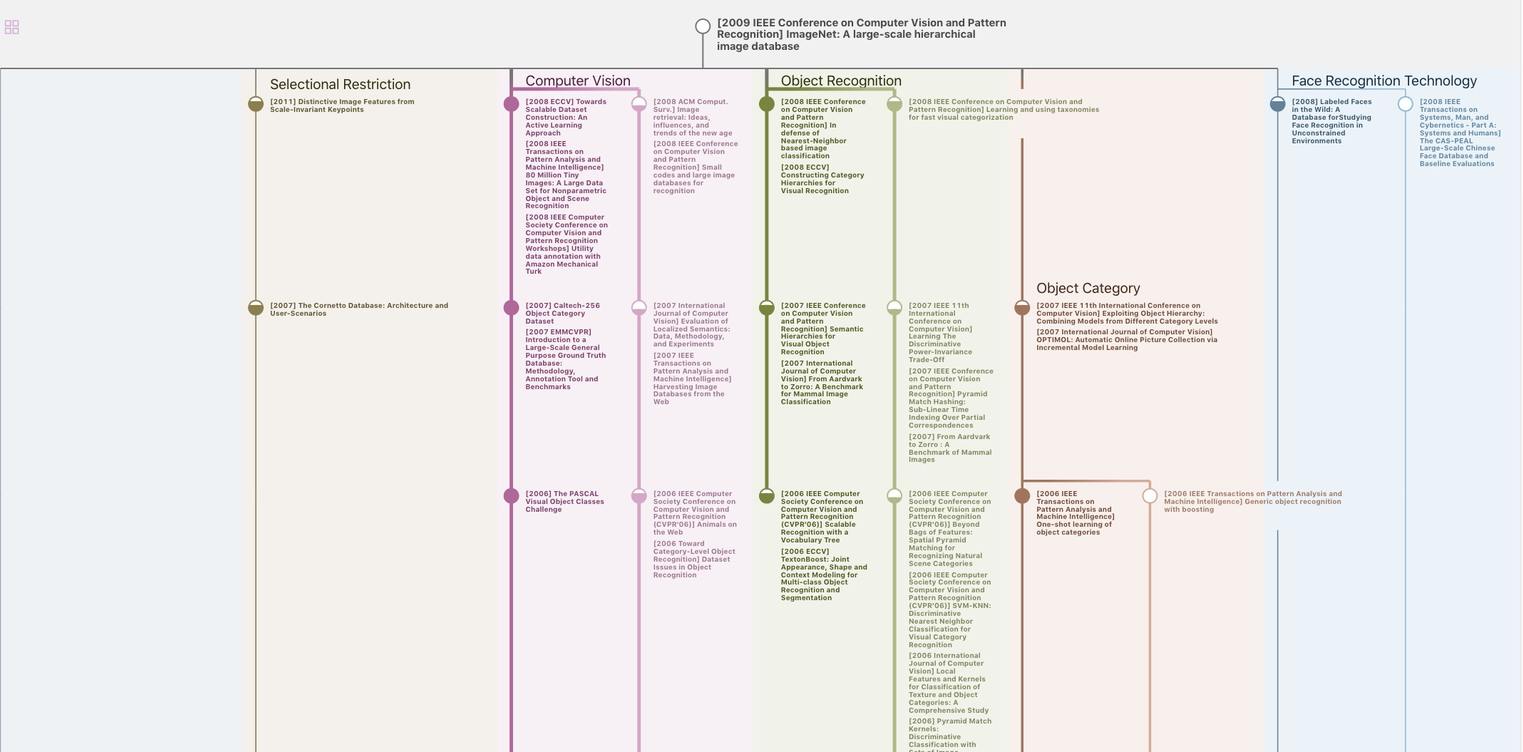
生成溯源树,研究论文发展脉络
Chat Paper
正在生成论文摘要