Performance Models for Heterogeneous Systems Applied to the Dark Silicon-Aware Design Space Exploration
2019 31st International Symposium on Computer Architecture and High Performance Computing (SBAC-PAD)(2019)
摘要
The design of computing systems requires tools for modeling, configuration, and simulation of multiple architectural parameters and their interconnections. Simulators provide estimated values of the system performance, power, and physical parameters, which are useful in future design decisions. Moreover, as target systems become complex, the number of combinations of architectural parameters that influence their performance increases exponentially, making a full architectural exploration a time-consuming or even impractical activity. This paper presents performance models for heterogeneous computing systems based on machine learning techniques. The models' inputs are sets of architectural parameters and the outputs are estimates of the platform performance. By delivering performance results with low computational cost and controlled error rates, our models become an efficient alternative to the costly process of estimating performance based on simulation. Our best model achieves an accuracy of 97.7% and relative mean error of 20.34%. We implement our models into the MultiExplorer, a dark siliconaware design space exploration tool that allows designers to explore the architecture and microarchitecture of a multicore system design. The adoption of an efficient performance predictor allows MultiExplorer to provide better performance accuracy while performing the design space exploration.
更多查看译文
关键词
Performance prediction,Machine Learning,Multiprocessor Systems,Design Space Exploration,Dark silicon
AI 理解论文
溯源树
样例
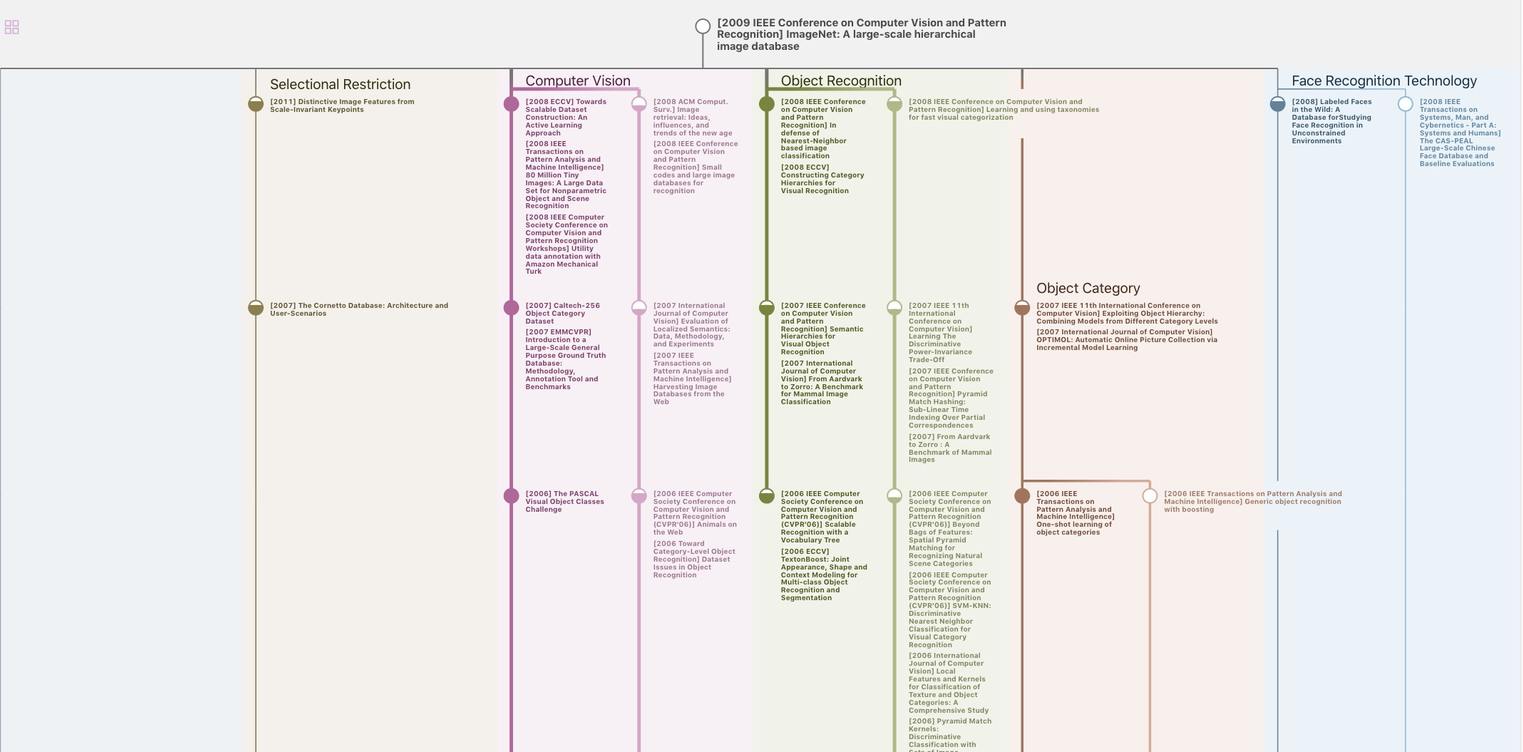
生成溯源树,研究论文发展脉络
Chat Paper
正在生成论文摘要