DeLHCA: Deep transfer learning for high-content analysis of the effects of drugs on immune cells
TENCON IEEE Region 10 Conference Proceedings(2019)
摘要
Analysis of high-content screening (HCS) data mostly relies on supervised machine learning based approaches employing user-defined image features. This strategy has limited applications due to the requirement of a priori knowledge of expected cellular phenotypes / perturbations and the time-consuming process of manually annotating these phenotypes. To address these issues, we propose a machine learning based unsupervised framework for high-content analysis. The framework performs anomaly detection using features transferred from natural images to the cellular images by deep learning models. We applied this framework to detect anomalous effects of FDA approved drugs on human monocytic cells. Drug anomaly detection based on image features derived using three deep learning architectures, DenseNet-121, ResNet-50 and VGG-16, is compared with the anomaly scores computed from user-defined features extracted from individually segmented cells. The drug anomaly scores of automatically extracted deep features and user-defined features were found to be comparable. Our method has broad implications for faster and reliable analysis of high-content data with limited human interaction which can provide new biological insights and identification of drug candidates for repurposing of FDA approved drugs for new clinical conditions.
更多查看译文
关键词
High-Content Imaging,Drug Discovery,Unsupervised Machine Learning,Convolutional Neural Networks
AI 理解论文
溯源树
样例
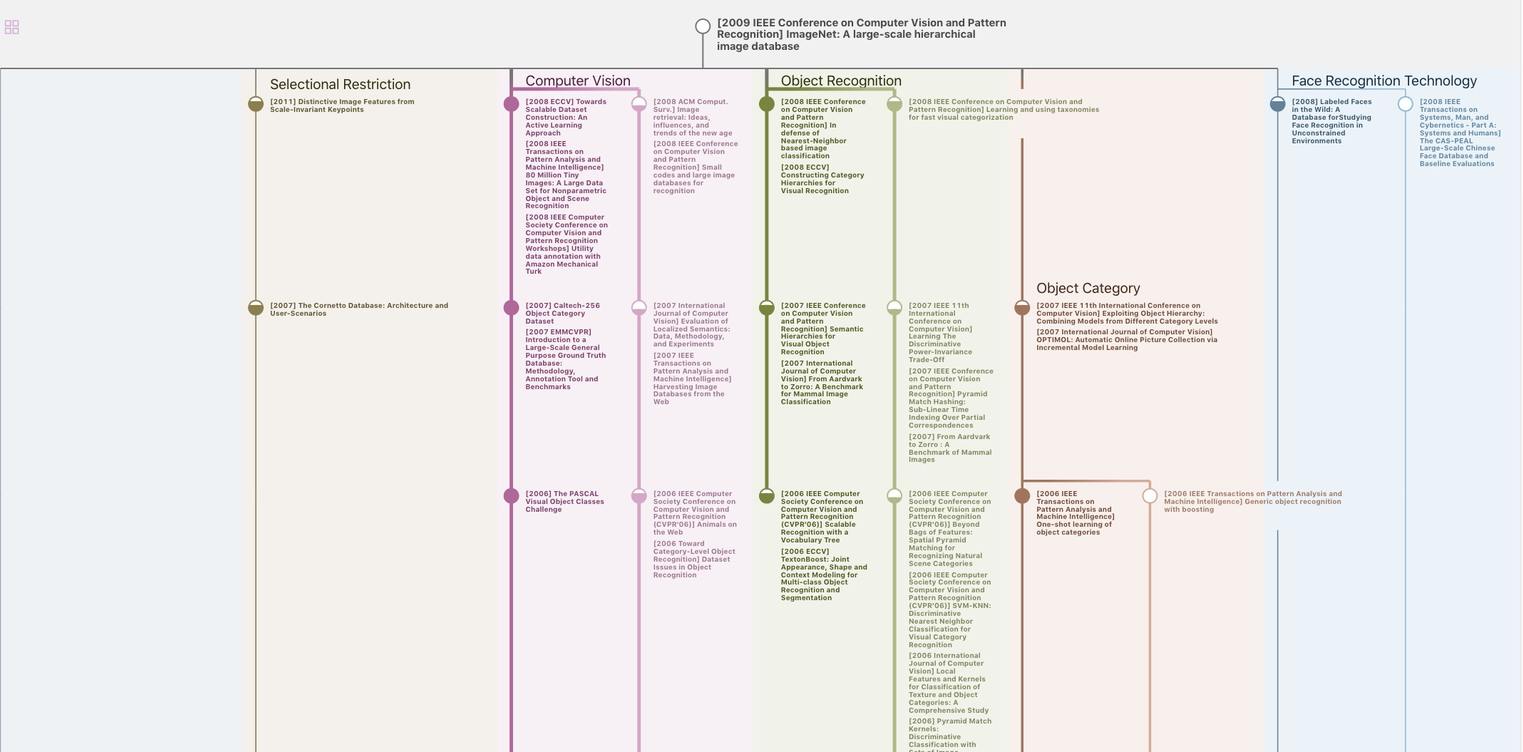
生成溯源树,研究论文发展脉络
Chat Paper
正在生成论文摘要