FIBS: A Generic Framework for Classifying Interval-based Temporal Sequences
DaWaK(2020)
摘要
We study the problem of classification of interval-based temporal sequences (IBTSs). Since common classification algorithms cannot be directly applied to IBTSs, the main challenge is to define a set of features that effectively represents the data such that learning classifiers are able to perform. Most prior work utilizes frequent pattern mining to define a feature set based on discovered patterns. However, frequent pattern mining is computationally expensive and often discovers many irrelevant patterns. To address this shortcoming, we propose the FIBS framework for classifying IBTSs. FIBS extracts features relevant to classification from IBTSs based on relative frequency and temporal relations. To avoid selecting irrelevant features, a filter-based selection strategy is incorporated into FIBS. Our empirical evaluation on five real-world datasets demonstrate the effectiveness of our methods in practice. The results provide evidence that FIBS framework effectively represents IBTSs for classification algorithms and it can even achieve better performance when the selection strategy is applied.
更多查看译文
关键词
Interval-based events, Temporal interval sequences, Feature-based classification framework
AI 理解论文
溯源树
样例
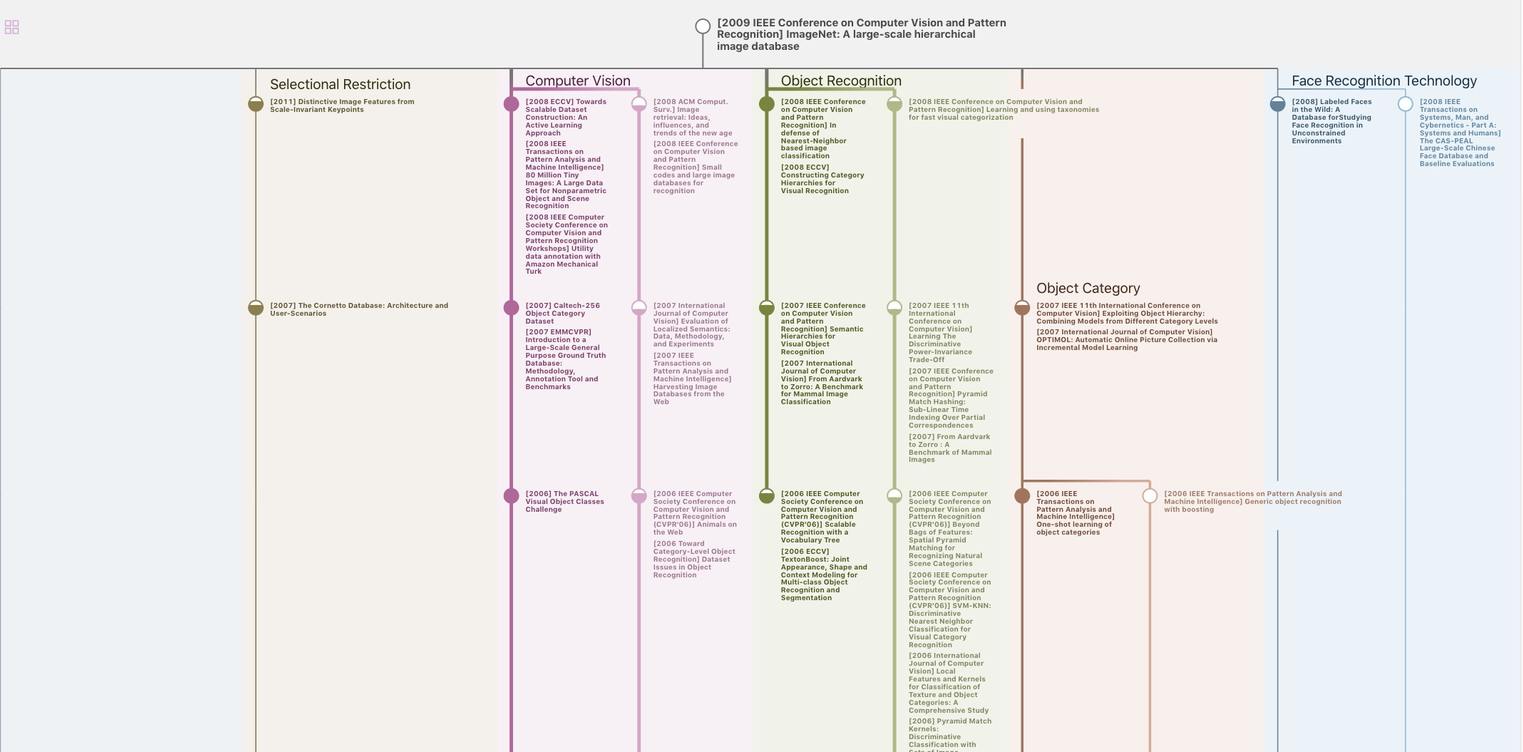
生成溯源树,研究论文发展脉络
Chat Paper
正在生成论文摘要