Interestingness elements for explainable reinforcement learning: Understanding agents' capabilities and limitations
Artificial Intelligence(2020)
摘要
We propose an explainable reinforcement learning (XRL) framework that analyzes an agent's history of interaction with the environment to extract interestingness elements that help explain its behavior. The framework relies on data readily available from standard RL algorithms, augmented with data that can easily be collected by the agent while learning. We describe how to create visual summaries of an agent's behavior in the form of short video-clips highlighting key interaction moments, based on the proposed elements. We also report on a user study where we evaluated the ability of humans to correctly perceive the aptitude of agents with different characteristics, including their capabilities and limitations, given visual summaries automatically generated by our framework. The results show that the diversity of aspects captured by the different interestingness elements is crucial to help humans correctly understand an agent's strengths and limitations in performing a task, and determine when it might need adjustments to improve its performance.
更多查看译文
关键词
Explainable AI,Reinforcement learning,Interestingness elements,Autonomy,Video highlights,Visual explanations
AI 理解论文
溯源树
样例
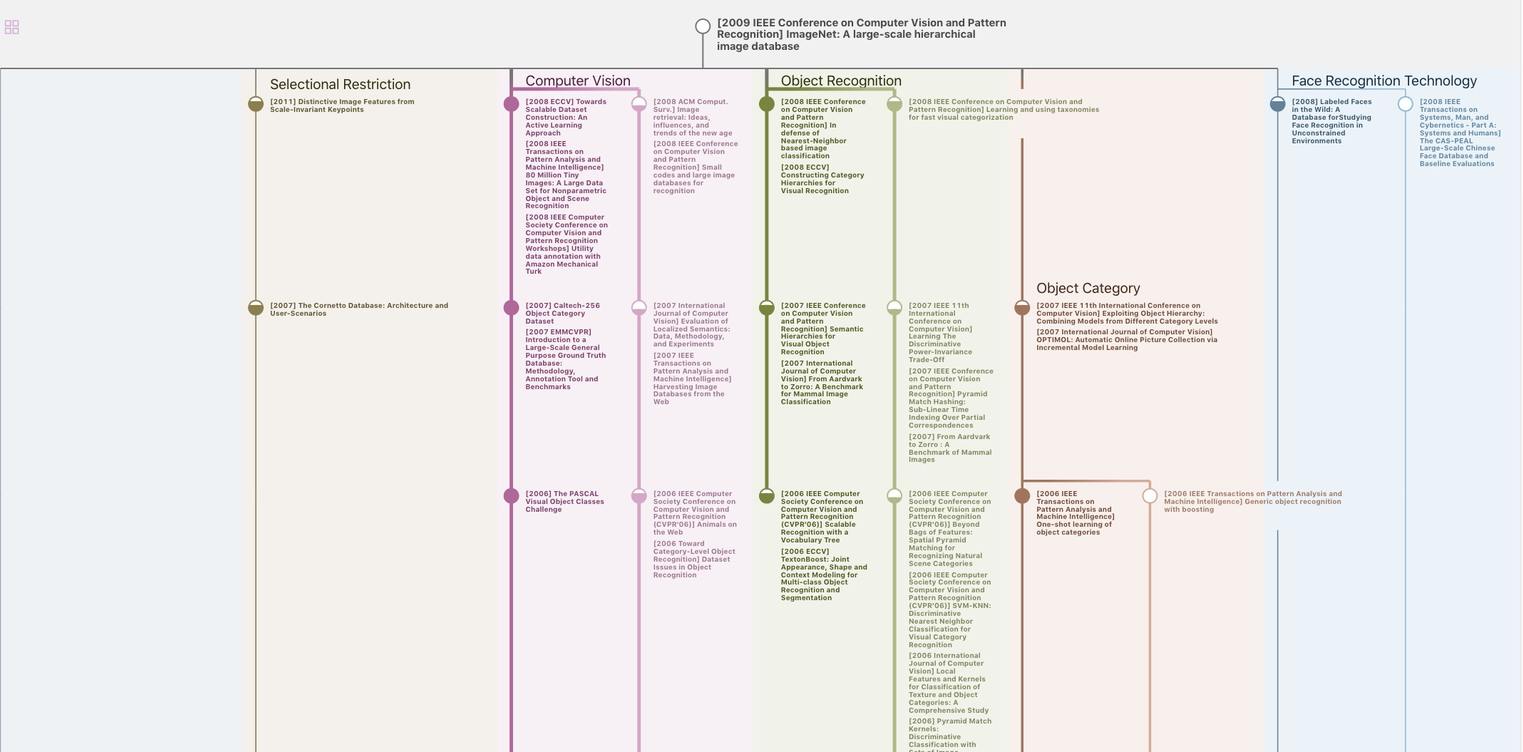
生成溯源树,研究论文发展脉络
Chat Paper
正在生成论文摘要