Real-time HR Estimation from wrist PPG using Binary LSTMs.
BioCAS(2019)
摘要
Wrist-worn photoplethysmography (PPG) sensors present a popular alternative to electrocardiogram recording for heart rate (HR) estimation. However, their accuracy is limited by motion artifacts inherent in ambulatory settings. In this paper, we propose a binarized neural network framework, b-CorNET, to efficiently estimate HR from single-channel wrist PPG signals during intense physical activity. The model comprises two binary convolution neural network layers followed by two binary long short-term memory (b-LSTM) layers and a dense layer working on quantized PPG data. The proposed framework achieves an MAE of 3.75±3.05 bpm when evaluated on 12 IEEE SPC subjects. Furthermore, a novel, low-complexity architecture for the b-LSTM layers is proposed and efficiently mapped on a Xilinx Virtex5 FPGA, enabling HR computation.
更多查看译文
关键词
PPG, Binary LSTM, CNN, FPGA
AI 理解论文
溯源树
样例
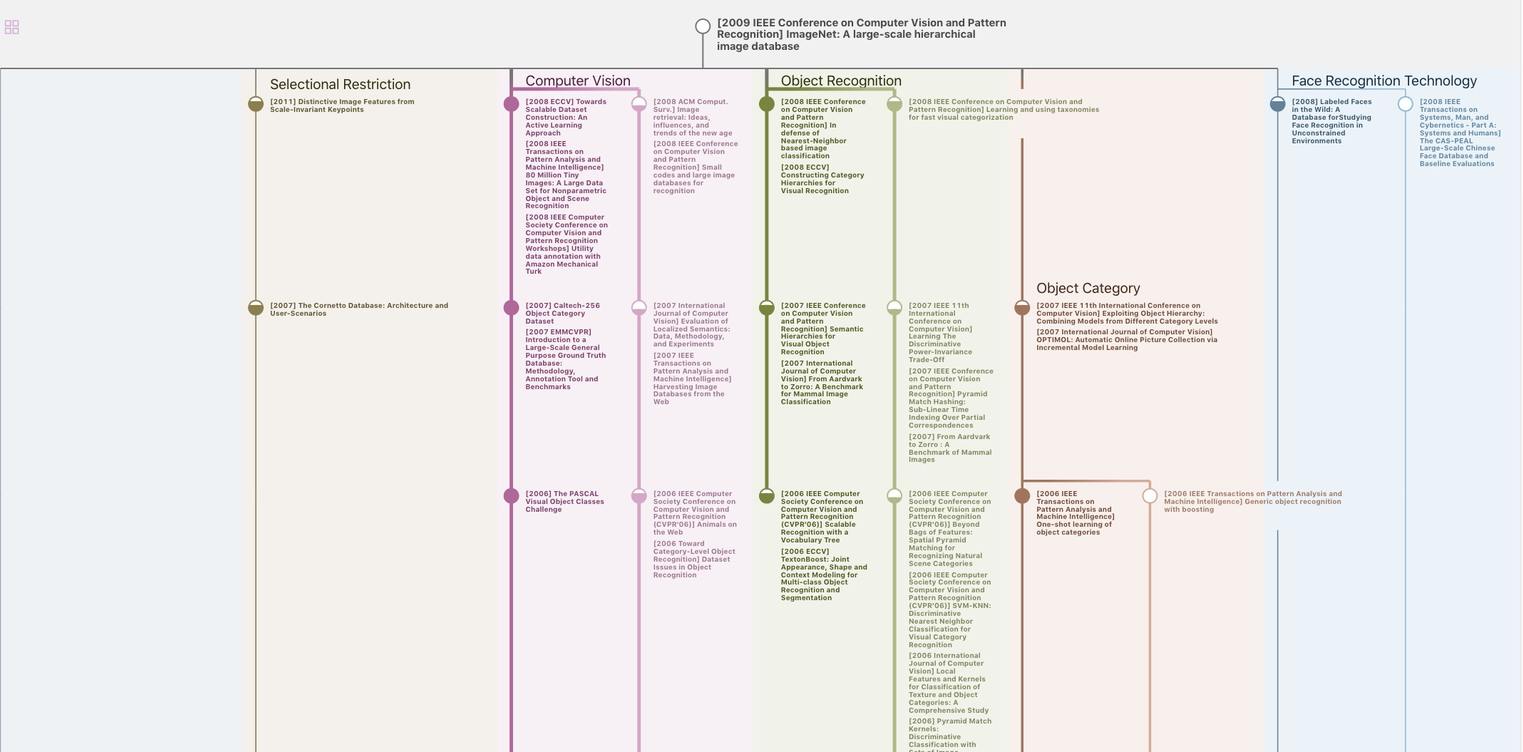
生成溯源树,研究论文发展脉络
Chat Paper
正在生成论文摘要