Benchmarking machine learning models for late-onset alzheimer’s disease prediction from genomic data
BMC Bioinformatics(2019)
摘要
Background Late-Onset Alzheimer’s Disease (LOAD) is a leading form of dementia. There is no effective cure for LOAD, leaving the treatment efforts to depend on preventive cognitive therapies, which stand to benefit from the timely estimation of the risk of developing the disease. Fortunately, a growing number of Machine Learning methods that are well positioned to address this challenge are becoming available. Results We conducted systematic comparisons of representative Machine Learning models for predicting LOAD from genetic variation data provided by the Alzheimer’s Disease Neuroimaging Initiative (ADNI) cohort. Our experimental results demonstrate that the classification performance of the best models tested yielded ∼72% of area under the ROC curve. Conclusions Machine learning models are promising alternatives for estimating the genetic risk of LOAD. Systematic machine learning model selection also provides the opportunity to identify new genetic markers potentially associated with the disease.
更多查看译文
关键词
Alzheimer’s disease,Machine learning,Benchmarking,Genome-wide association studies
AI 理解论文
溯源树
样例
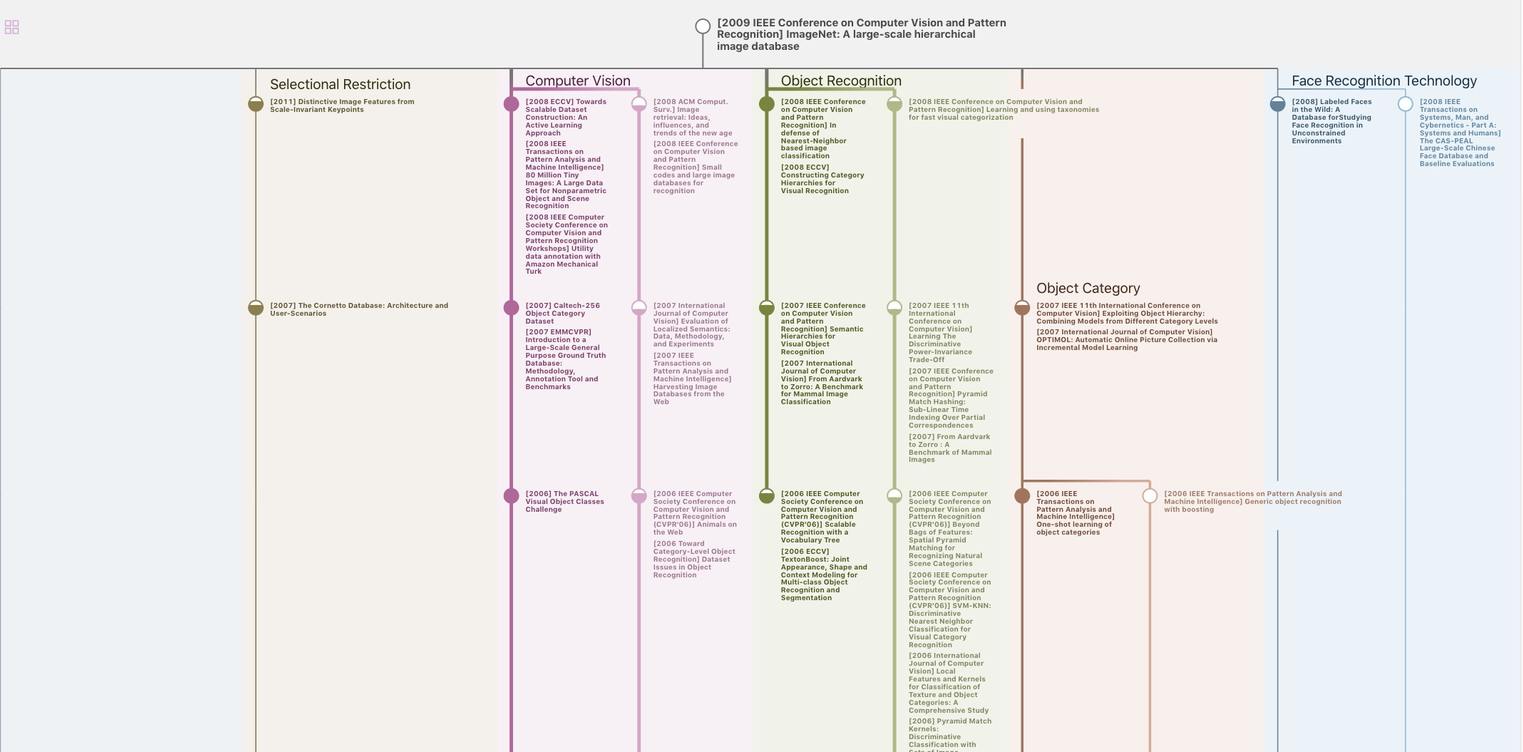
生成溯源树,研究论文发展脉络
Chat Paper
正在生成论文摘要