C-Flow: Conditional Generative Flow Models for Images and 3D Point Clouds
CVPR(2020)
摘要
Flow-based generative models have highly desirable properties like exact log-likelihood evaluation and exact latent-variable inference, however they are still in their infancy and have not received as much attention as alternative generative models. In this paper, we introduce C-Flow, a novel conditioning scheme that brings normalizing flows to an entirely new scenario with great possibilities for multi-modal data modeling. C-Flow is based on a parallel sequence of invertible mappings in which a source flow guides the target flow at every step, enabling fine-grained control over the generation process. We also devise a new strategy to model unordered 3D point clouds that, in combination with the conditioning scheme, makes it possible to address 3D reconstruction from a single image and its inverse problem of rendering an image given a point cloud. We demonstrate our conditioning method to be very adaptable, being also applicable to image manipulation, style transfer and multi-modal image-to-image mapping in a diversity of domains, including RGB images, segmentation maps, and edge masks.
更多查看译文
关键词
C-Flow,multimodal data modeling,source flow,target flow,unordered 3D point clouds,3D reconstruction,single image,image manipulation,multimodal image-to-image mapping,RGB images,conditional generative flow models,exact log-likelihood evaluation,latent-variable inference,alternative generative models,image rendering,segmentation maps,edge masks,inverse problem,parallel sequence,invertible mappings
AI 理解论文
溯源树
样例
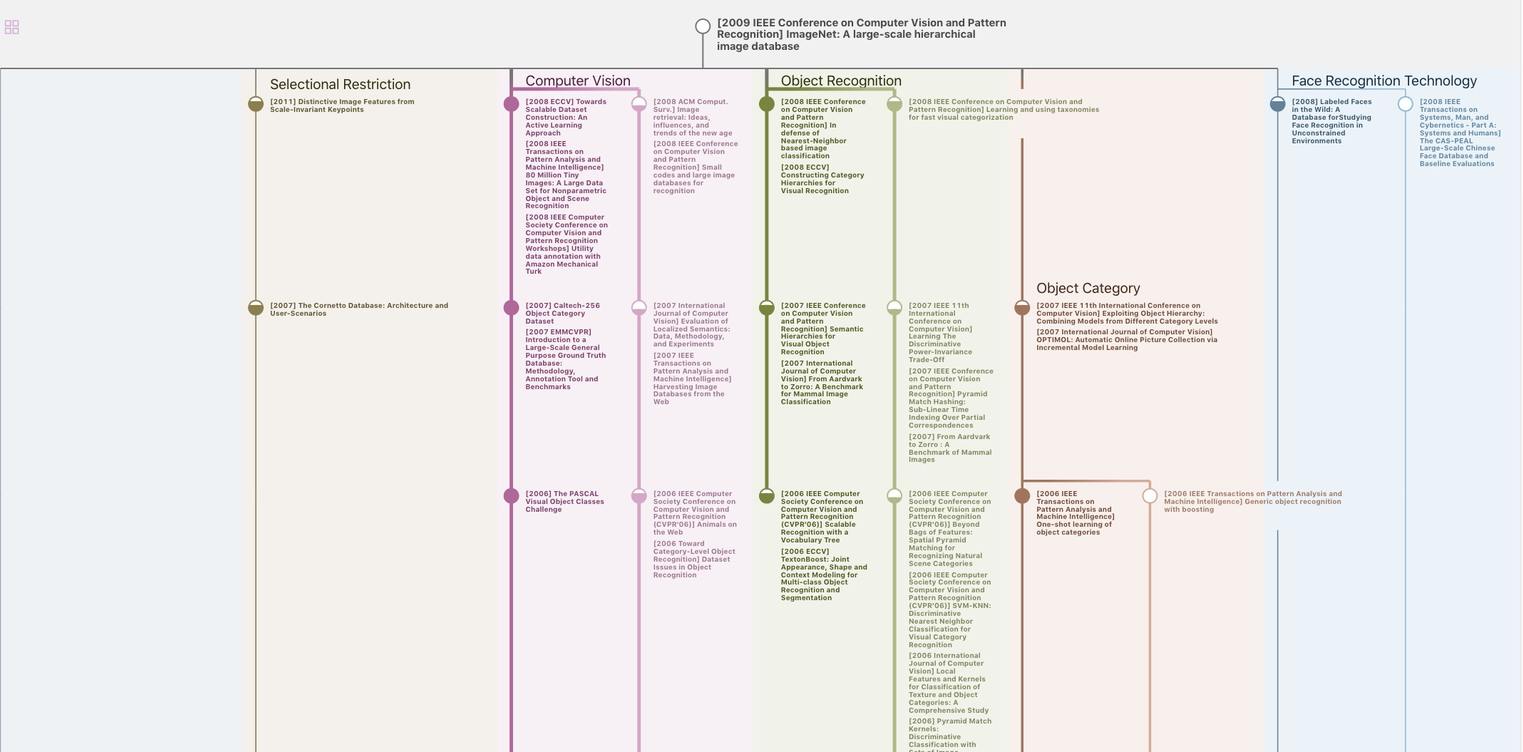
生成溯源树,研究论文发展脉络
Chat Paper
正在生成论文摘要