Recovering Noisy-Pseudo-Sparse Signals From Linear Measurements via l∞.
Allerton(2019)
摘要
Compressive sensing (CS) can recover a sparse signal x reliably under an indefinite linear system. However, corruption with noise can severely damage the system performance. For Gaussian deviation, a noise whitening method is often used which leads to the noise folding phenomenon, increasing the noise energy greatly. In this paper, we introduce a different approach and design a new optimization model to recover x with f, norm. Moreover, in our setup, the signal (prior to corruption by noise) is only pseudo sparse. We analyze the solution exactness and show that a unique solution close to the true values of pseudo -sparse signals can he obtained in an indefinite system with unibwm magnitude noise. We then relax the uniform -magnitude assumption and use Gaussian noise in simulations. We show that, compared to the noise -whitening method, our method can reduce almost 50% of the noise by only sacrificing less than 0.3% of the support -set recovery rate.
更多查看译文
关键词
noisy-pseudosparse signals,linear measurements,compressive sensing,sparse signal x,indefinite linear system,corruption,system performance,Gaussian deviation,noise whitening method,noise folding phenomenon,noise energy,solution exactness,unique solution close,indefinite system,uniform magnitude noise,uniform-magnitude assumption,noise-whitening method
AI 理解论文
溯源树
样例
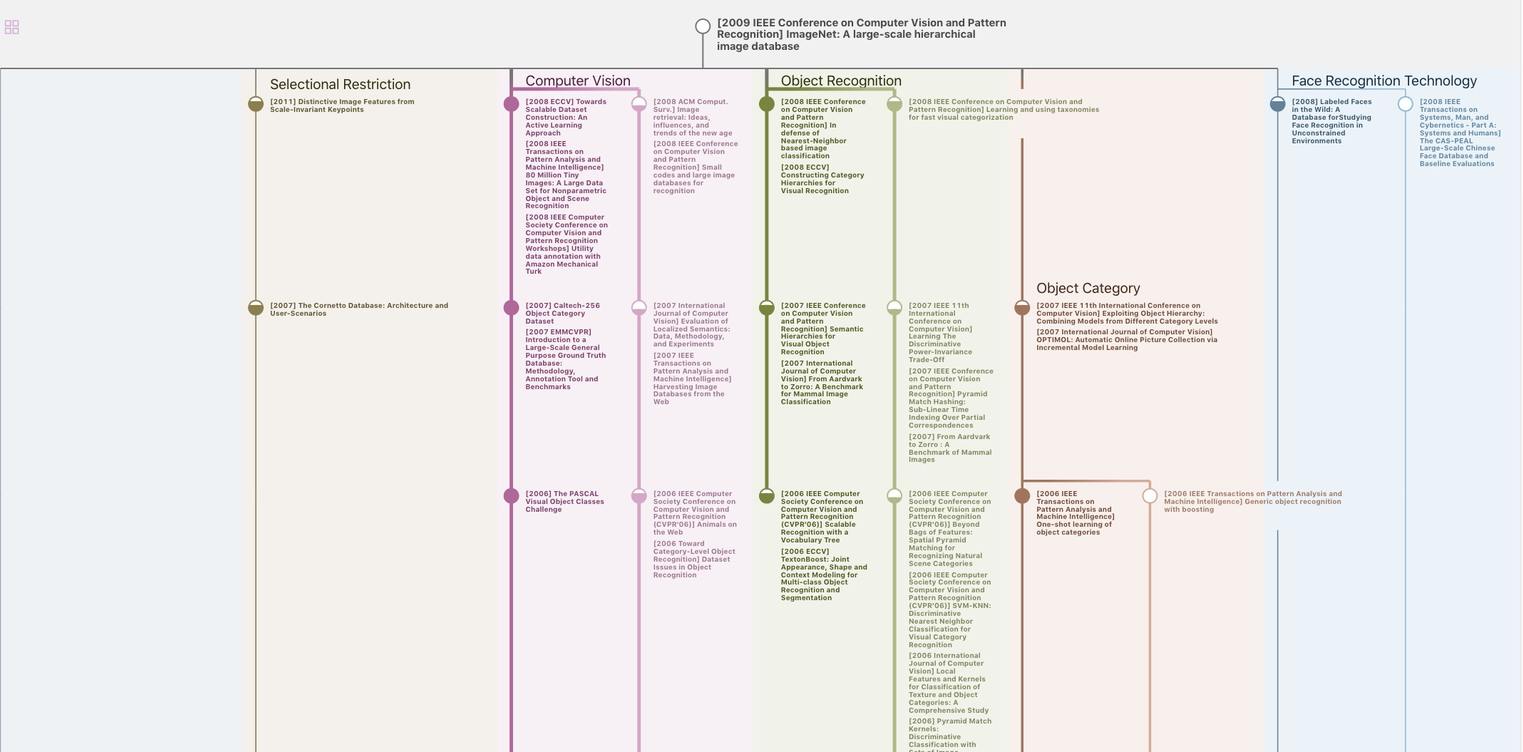
生成溯源树,研究论文发展脉络
Chat Paper
正在生成论文摘要