Privacy-Preserving Adversarial Networks
2019 57th Annual Allerton Conference on Communication, Control, and Computing (Allerton)(2019)
摘要
We propose a data-driven framework for optimizing privacy-preserving data release mechanisms to attain the information-theoretically optimal tradeoff between minimizing distortion of useful data and concealing specific sensitive information. Our approach employs adversarially-trained neural networks to implement randomized mechanisms and to perform a variational approximation of mutual information privacy. We validate our Privacy-Preserving Adversarial Networks (PPAN) framework via proof-of-concept experiments on discrete and continuous synthetic data, as well as the MNIST handwritten digits dataset. For synthetic data, our model-agnostic PPAN approach achieves tradeoff points very close to the optimal tradeoffs that are analytically-derived from model knowledge. In experiments with the MNIST data, we visually demonstrate a learned tradeoff between minimizing the pixel-level distortion versus concealing the written digit.
更多查看译文
关键词
discrete synthetic data,continuous synthetic data,privacy-preserving adversarial network framework,mutual information privacy,randomized mechanisms,neural networks,sensitive information,information-theoretically optimal tradeoff,privacy-preserving data,data-driven framework,model-agnostic PPAN approach,MNIST handwritten digit dataset
AI 理解论文
溯源树
样例
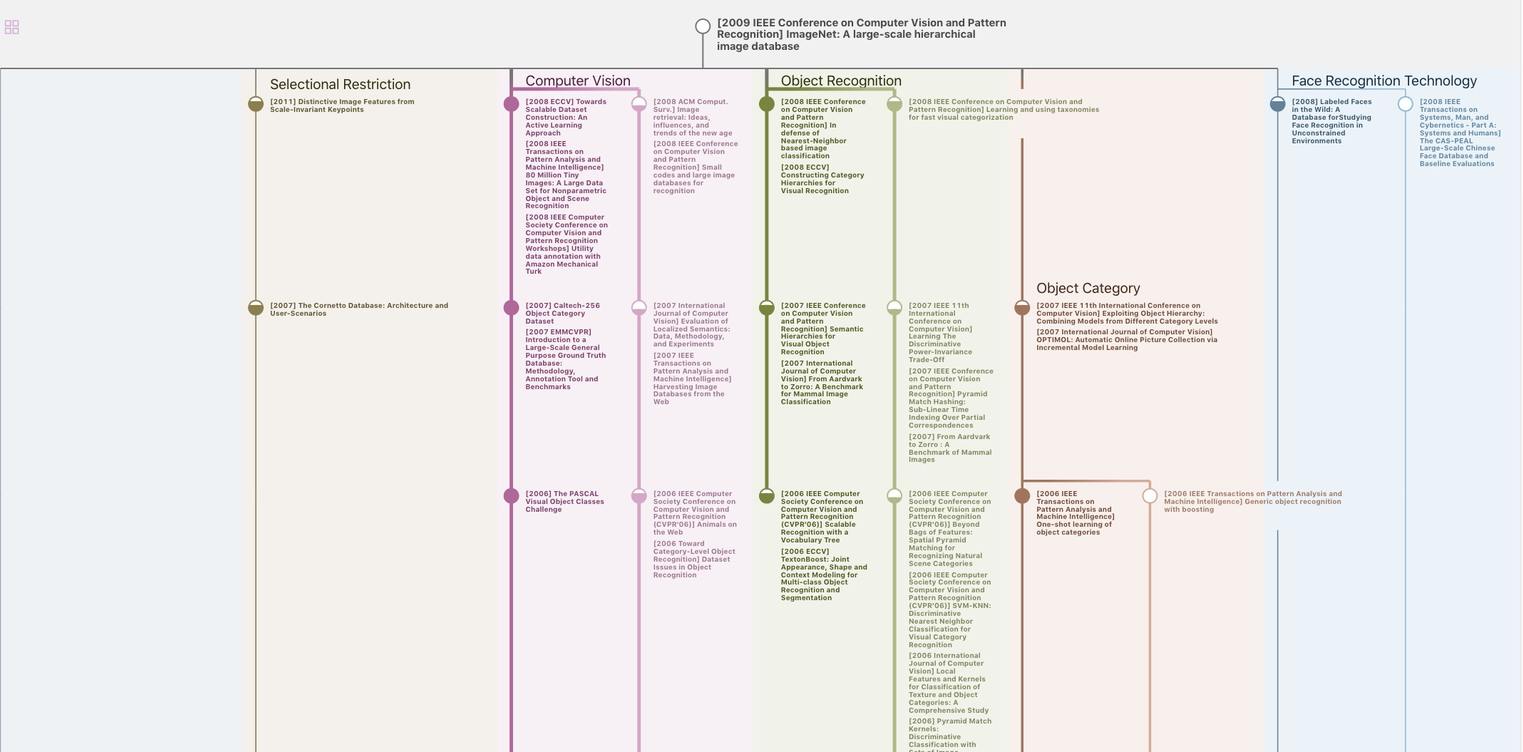
生成溯源树,研究论文发展脉络
Chat Paper
正在生成论文摘要