Maximin Active Learning with Data-Dependent Norms
2019 57TH ANNUAL ALLERTON CONFERENCE ON COMMUNICATION, CONTROL, AND COMPUTING (ALLERTON)(2019)
摘要
Overparameterized machine learning models are often fit perfectly to training data, yet remarkably generalize well to new data. However, learning good models can require an enormous number of labeled training data. This challenge motivates the study of active learning algorithms that sequentially and adaptively request labels for "informative" examples for a large pool of unlabeled data. A maximin criterion was recently proposed for active learning specifically in the overparameterized and interpolating regime. Roughly speaking, the maximin criterion selects the example that is most difficult to interpolate, as measured by an appropriate norm on the interpolating function. Data-dependent norms perform best empirically, exhibiting intriguing adaptivity to cluster structure within the data. The main contribution of this paper is to mathematically characterize this behavior. Our main results show that the maximin criterion based on data-dependent norms provably discovers clusters and also automatically generates labeled coverings of the dataset.
更多查看译文
关键词
Active Learning,Reproducing Hilbert Kernel Spaces,Data-dependent Norm
AI 理解论文
溯源树
样例
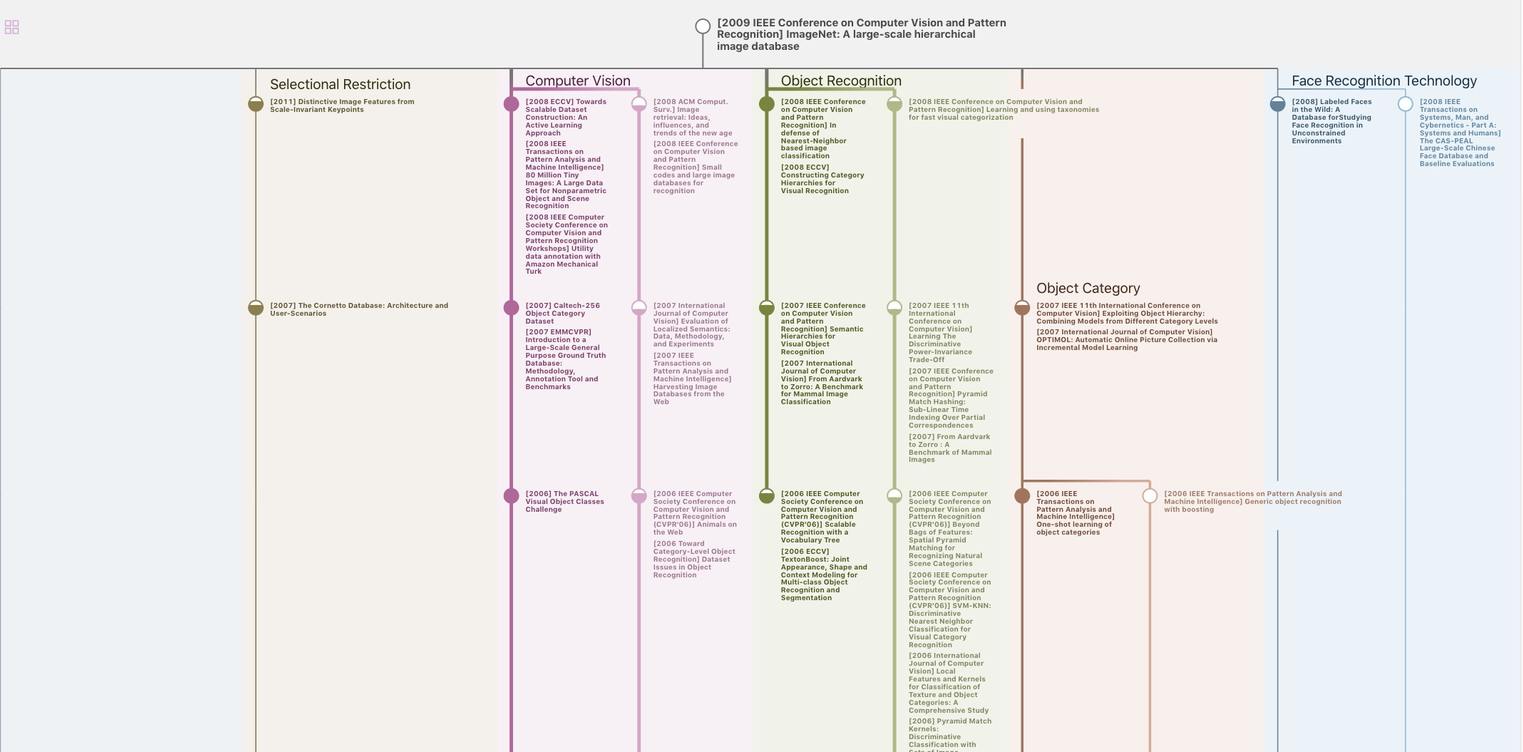
生成溯源树,研究论文发展脉络
Chat Paper
正在生成论文摘要