Combining gene expression programming and genetic algorithm as a powerful hybrid modeling approach for pear rootstocks tissue culture media formulation
Plant Methods(2019)
摘要
Background Predicting impact of plant tissue culture media components on explant proliferation is important especially in commercial scale for optimizing efficient culture media. Previous studies have focused on predicting the impact of media components on explant growth via conventional multi-layer perceptron neural networks (MLPNN) and Multiple Linear Regression (MLR) methods. So, there is an opportunity to find more efficient algorithms such as Radial Basis Function Neural Network (RBFNN) and Gene Expression Programming (GEP). Here, a novel algorithm, i.e. GEP which has not been previously applied in plant tissue culture researches was compared to RBFNN and MLR for the first time. Pear rootstocks (Pyrodwarf and OHF) were used as case studies on predicting the effect of minerals and some hormones in the culture medium on proliferation indices. Results Generally, RBFNN and GEP showed extremely higher performance accuracy than the MLR. Moreover, GEP models as the most accurate models were optimized using genetic algorithm (GA). The improvement was mainly due to the RBFNN and GEP strong estimation capability and their superior tolerance to experimental noises or improbability. Conclusions GEP as the most robust and accurate prospecting procedure to achieve the highest proliferation quality and quantity has also the benefit of being easy to use.
更多查看译文
关键词
Gene expression programming, Radial basis function neural network, Genetic algorithm, Multiple linear regression, Pyrodwarf, OHF, Pear rootstock
AI 理解论文
溯源树
样例
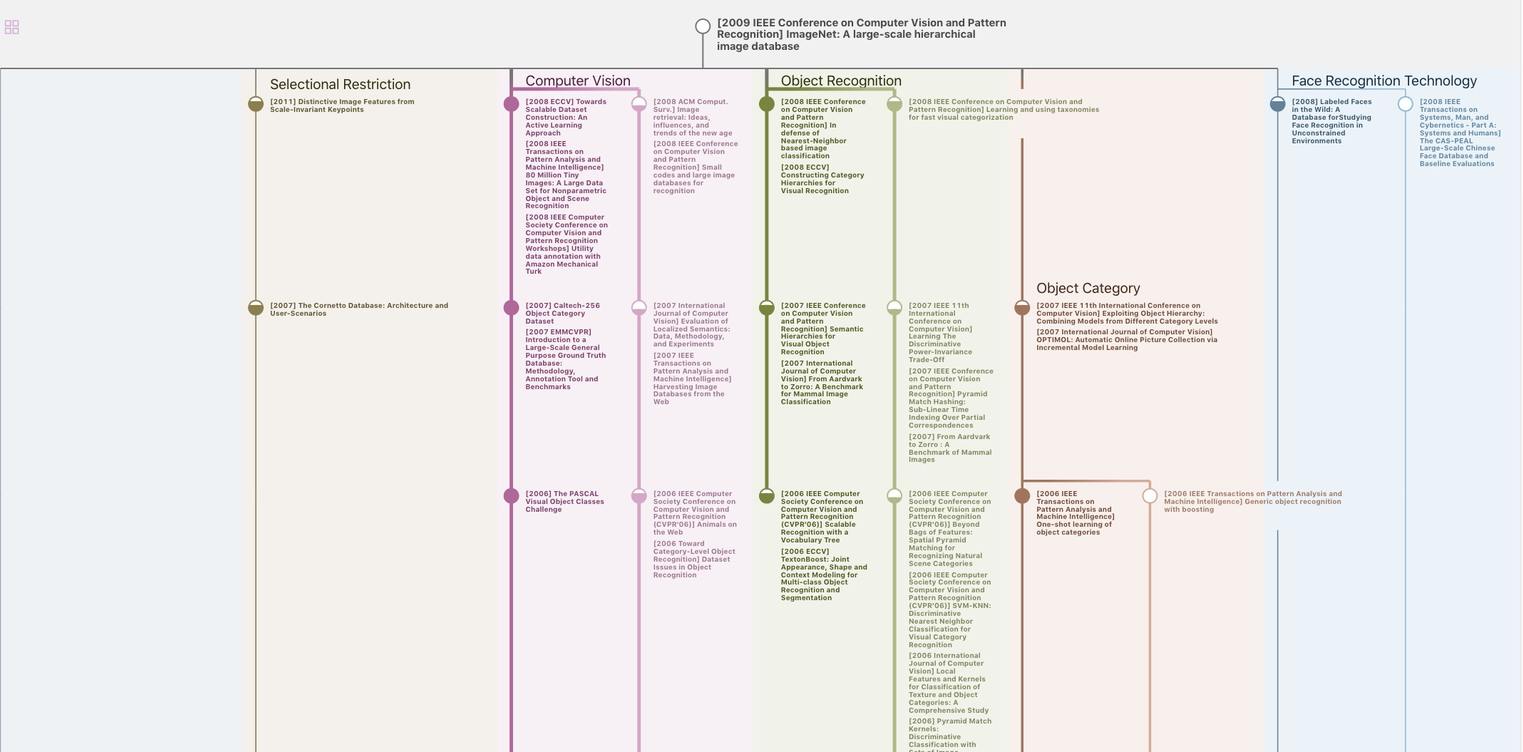
生成溯源树,研究论文发展脉络
Chat Paper
正在生成论文摘要