Taking A Look At Small-Scale Pedestrians And Occluded Pedestrians
IEEE TRANSACTIONS ON IMAGE PROCESSING(2020)
摘要
Small-scale pedestrian detection and occluded pedestrian detection are two challenging tasks. However, most state-of-the-art methods merely handle one single task each time, thus giving rise to relatively poor performance when the two tasks, in practice, are required simultaneously. In this paper, it is found that small-scale pedestrian detection and occluded pedestrian detection actually have a common problem, i.e., an inaccurate location problem. Therefore, solving this problem enables to improve the performance of both tasks. To this end, we pay more attention to the predicted bounding box with worse location precision and extract more contextual information around objects, where two modules (i.e., location bootstrap and semantic transition) are proposed. The location bootstrap is used to reweight regression loss, where the loss of the predicted bounding box far from the corresponding ground-truth is upweighted and the loss of the predicted bounding box near the corresponding ground-truth is downweighted. Additionally, the semantic transition adds more contextual information and relieves semantic inconsistency of the skip-layer fusion. Since the location bootstrap is not used at the test stage and the semantic transition is lightweight, the proposed method does not add many extra computational costs during inference. Experiments on the challenging CityPersons and Caltech datasets show that the proposed method outperforms the state-of-the-art methods on the small-scale pedestrians and occluded pedestrians (e.g., 5.20 and 4.73 improvements on the Caltech).
更多查看译文
关键词
Small-scale pedestrians, occluded pedestrians, location bootstrap, semantic transition
AI 理解论文
溯源树
样例
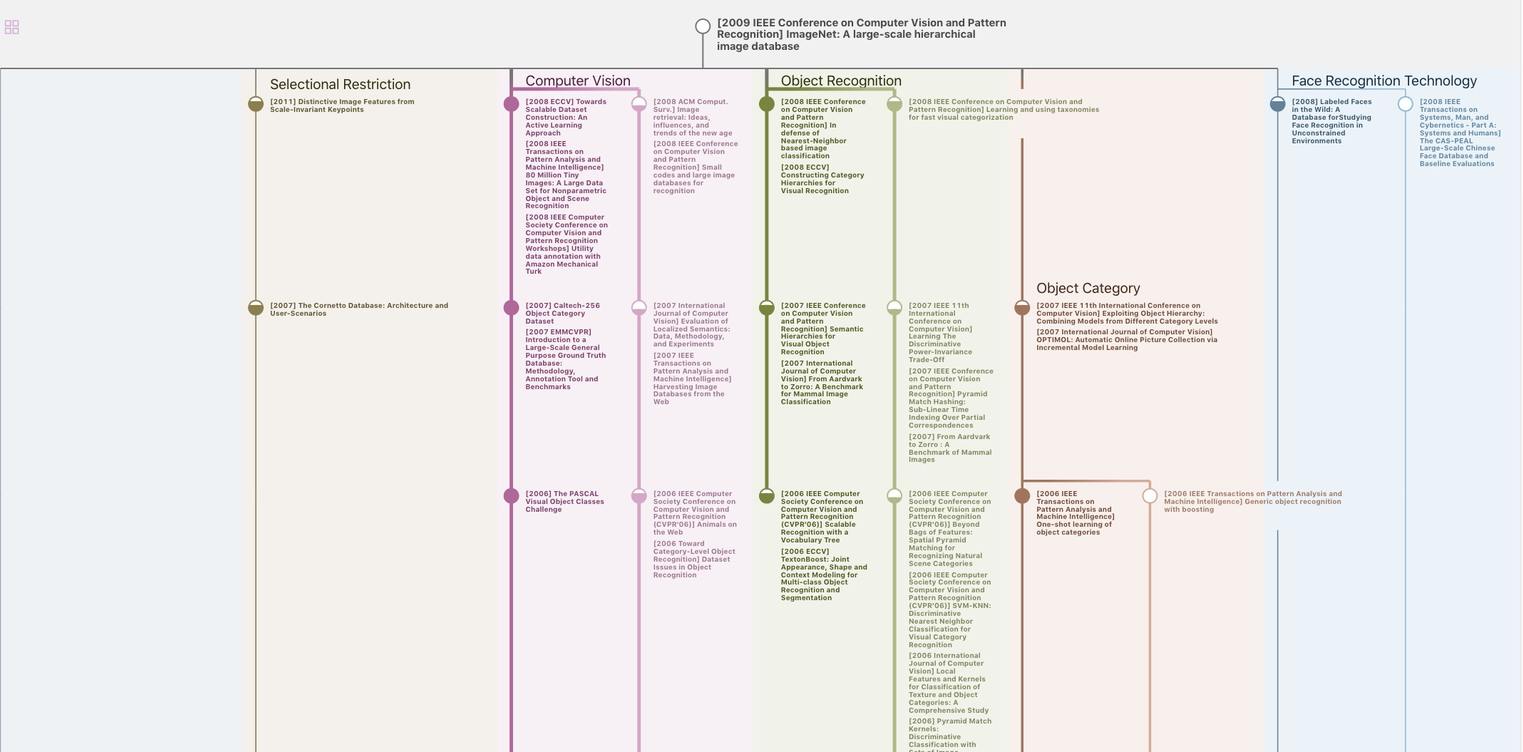
生成溯源树,研究论文发展脉络
Chat Paper
正在生成论文摘要