Unknown Signal Detection In Linear Dynamical System Noise
2019 IEEE 29th International Workshop on Machine Learning for Signal Processing (MLSP)(2019)
摘要
A machine learning approach to detecting unknown signals in time-correlated noise is presented. In the proposed approach, a linear dynamical system (LDS) model is trained to represent the background noise via expectation-maximization (EM). The negative log-likelihood (NLL) of test data under the learned background noise LDS is computed via the Kalman filter recursions, and an unknown signal is detected if the NLL exceeds a threshold. The proposed detection scheme is derived as a generalized likelihood ratio test (GLRT) for an unknown deterministic signal in LDS noise. In simple additive white Gaussian noise (AWGN), the proposed scheme reduces to an energy detector. However, experimental results on a wireless software defined radio (SDR) testbed demonstrate that the proposed scheme substantially outperforms energy detection in a time-correlated noise background.
更多查看译文
关键词
Signal detection,Kalman filter,linear dynamical system,expectation-maximization,generalized likelihood ratio test
AI 理解论文
溯源树
样例
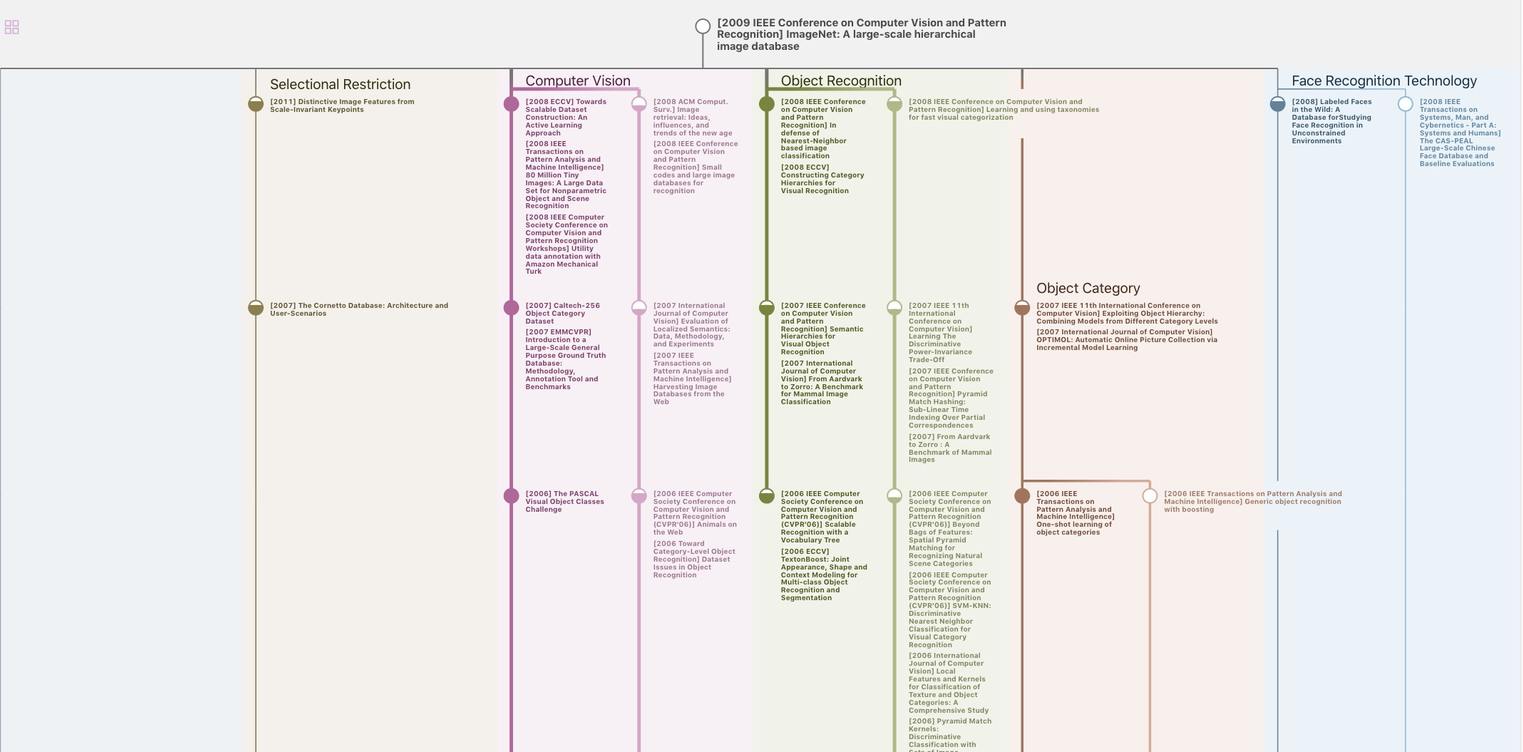
生成溯源树,研究论文发展脉络
Chat Paper
正在生成论文摘要