Extending Machine Language Models toward Human-Level Language Understanding
arxiv(2019)
摘要
Language is central to human intelligence. We review recent breakthroughs in machine language processing and consider what remains to be achieved. Recent approaches rely on domain general principles of learning and representation captured in artificial neural networks. Most current models, however, focus too closely on language itself. In humans, language is part of a larger system for acquiring, representing, and communicating about objects and situations in the physical and social world, and future machine language models should emulate such a system. We describe existing machine models linking language to concrete situations, and point toward extensions to address more abstract cases. Human language processing exploits complementary learning systems, including a deep neural network-like learning system that learns gradually as machine systems do, as well as a fast-learning system that supports learning new information quickly. Adding such a system to machine language models will be an important further step toward truly human-like language understanding.
更多查看译文
AI 理解论文
溯源树
样例
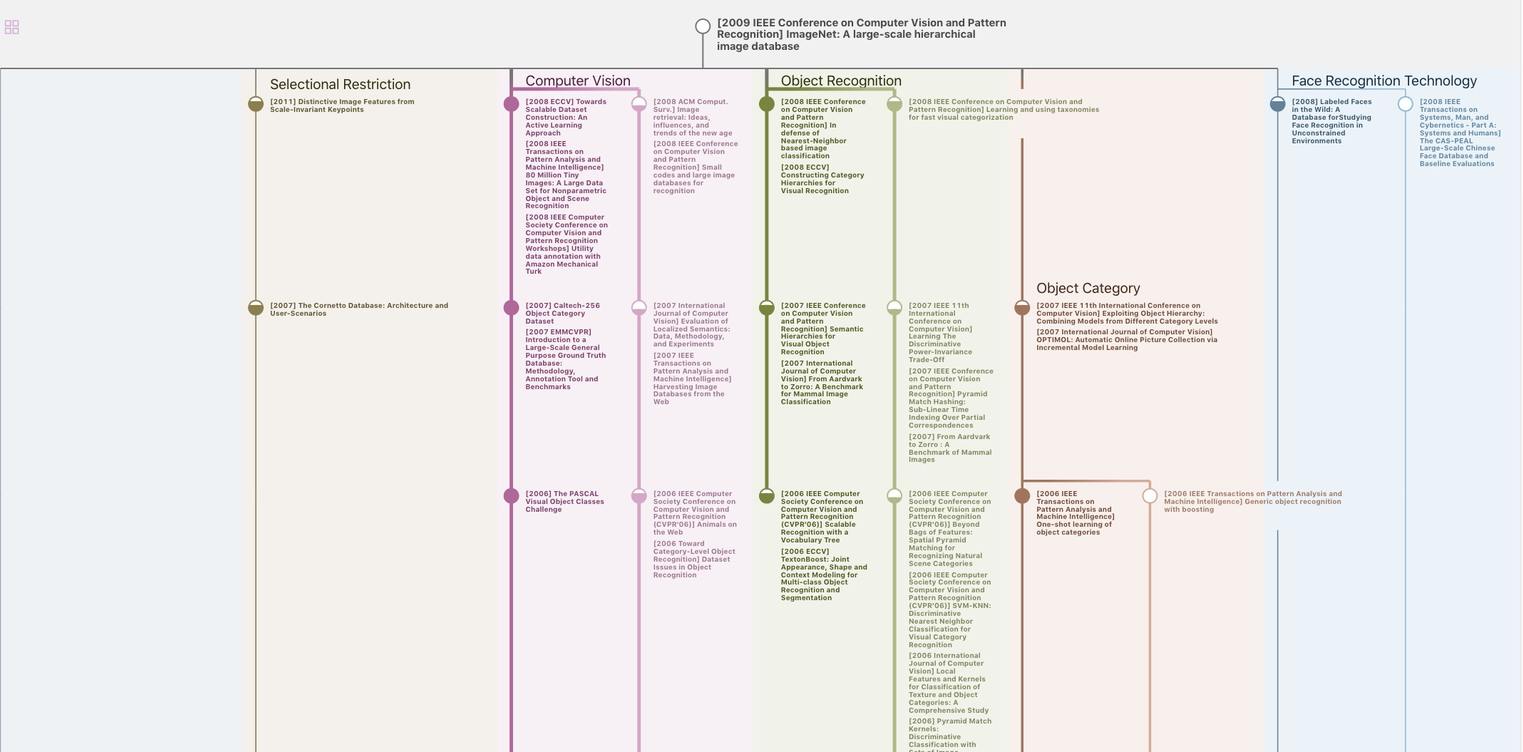
生成溯源树,研究论文发展脉络
Chat Paper
正在生成论文摘要