Learned Variable-Rate Image Compression With Residual Divisive Normalization
2020 IEEE INTERNATIONAL CONFERENCE ON MULTIMEDIA AND EXPO (ICME)(2020)
摘要
Recently deep learning-based image compression has shown the potential to outperform traditional codecs. However, most existing methods train multiple networks for multiple bit rates, which increases the implementation complexity. In this paper, we propose a variable-rate image compression framework, which employs more Generalized Divisive Normalization (GDN) layers than previous GDN-based methods. Novel GDN-based residual sub-networks are also developed in the encoder and decoder networks. Our scheme also uses a stochastic rounding-based scalar quantization. To further improve the performance, we encode the residual between the input and the reconstructed image from the decoder network as an enhancement layer. To enable a single model to operate with different bit rates and to learn multi-rate image features, a new objective function is introduced. Experimental results show that the proposed framework trained with variable-rate objective function outperforms all standard codecs such as H.265/HEVC-based BPG and state-of-the-art learning-based variable-rate methods.
更多查看译文
关键词
image compression, variable-rate, deep learning, residual coding
AI 理解论文
溯源树
样例
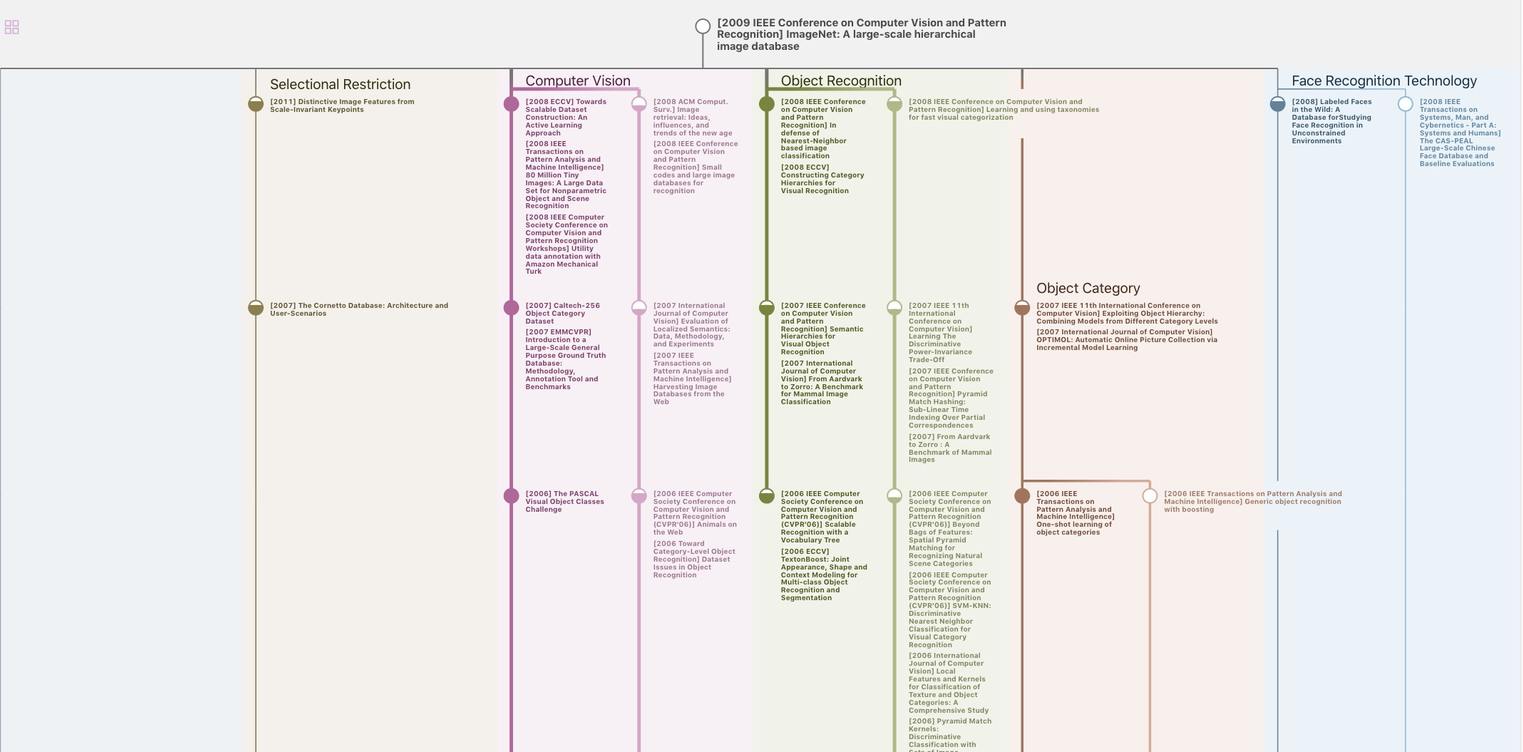
生成溯源树,研究论文发展脉络
Chat Paper
正在生成论文摘要