Managing Machine Learning Workflow Components
INTERNATIONAL JOURNAL OF SEMANTIC COMPUTING(2020)
摘要
Machine Learning Workflows (MLWfs) have become an essential and disruptive approach in problem-solving over several industries. However, the development process of MLWfs may be complex, time-consuming, and error-prone. To handle this problem, we introduce machine learning workflow management (MLWfM) as a technique to aid the development and reuse of MLWfs and their components through three aspects: representation, execution, and creation. We introduce our approach to structure MLWfs' components and metadata in order to aid component retrieval and reuse of new MLWfs. We also consider the execution of these components within a tool. A hybrid knowledge representation, called Hyperknowledge, frames our methodology, supporting the three MLWfM's aspects. To validate our approach, we show a practical use case in the Oil & Gas industry. In addition, to evaluate the feasibility of the proposed technique, we create a dataset of MLWfs executions and discuss the MLWfM's performance in loading and querying this dataset.
更多查看译文
关键词
Machine learning,workflow management,Hyperknowledge
AI 理解论文
溯源树
样例
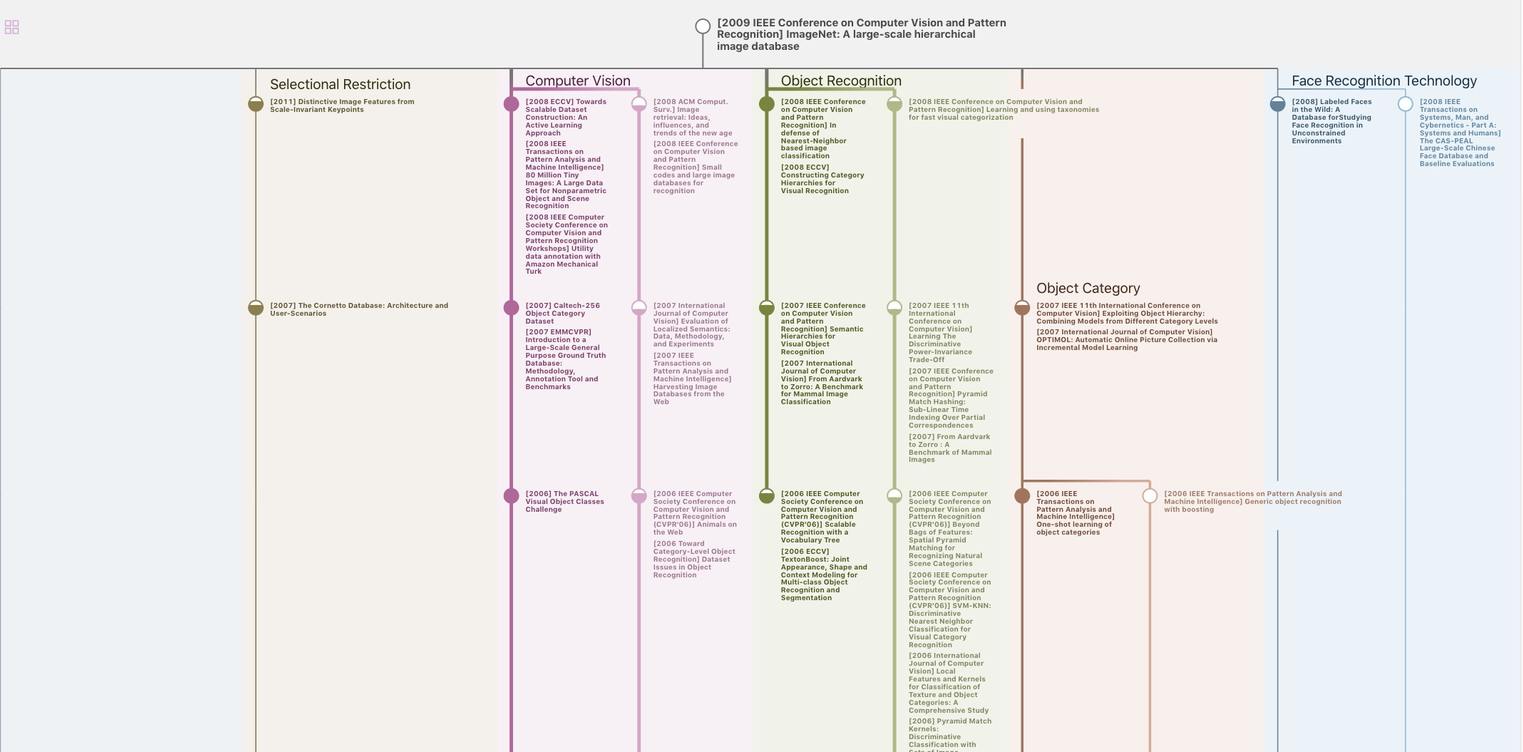
生成溯源树,研究论文发展脉络
Chat Paper
正在生成论文摘要