Saffron: Adaptive Grammar-based Fuzzing for Worst-Case Analysis
ACM SIGSOFT Software Engineering Notes(2019)
摘要
Fuzz testing has been gaining ground recently with substantial e orts devoted to the area. Typically, fuzzers take a set of seed inputs and leverage random mutations to continually improve the inputs with respect to a cost, e.g. program code coverage, to discover vulnerabilities or bugs. Following this methodology, fuzzers are very good at generating unstructured inputs that achieve high coverage. However fuzzers are less e ective when the inputs are structured, say they conform to an input grammar. Due to the nature of random mutations, the overwhelming abundance of inputs generated by this common fuzzing practice often adversely hinders the effectiveness and efficiency of fuzzers on grammar-aware applications. The problem of testing becomes even harder, when the goal is not only to achieve increased code coverage, but also to nd complex vulnerabilities related to other cost measures, say high resource consumption in an application.
We propose Saffron an adaptive grammar-based fuzzing approach to effectively and efficiently generate inputs that expose expensive executions in programs. Saffron takes as input a user-provided grammar, which describes the input space of the program under analysis, and uses it to generate test inputs. Saffron assumes that the grammar description is approximate since precisely describing the input program space is often difficult as a program may accept unintended inputs due to e.g., errors in parsing. Yet these inputs may reveal worst-case complexity vulnerabilities. The novelty of Saffron is then twofold: (1) Given the user-provided grammar, Saffron attempts to discover whether the program accepts unexpected inputs outside of the provided grammar, and if so, it repairs the grammar via grammar mutations. The repaired grammar serves as a speci cation of the actual inputs accepted by the application. (2) Based on the re ned grammar, it generates concrete test inputs. It starts by treating every production rule in the grammar with equal probability of being used for generating concrete inputs. It then adaptively re nes the probabilities along the way by increasing the probabilities for rules that have been used to generate inputs that improve a cost, e.g., code coverage or arbitrary user-de ned cost. Evaluation results show that Saffron signi cantly outperforms state-of-the-art baselines.
更多查看译文
AI 理解论文
溯源树
样例
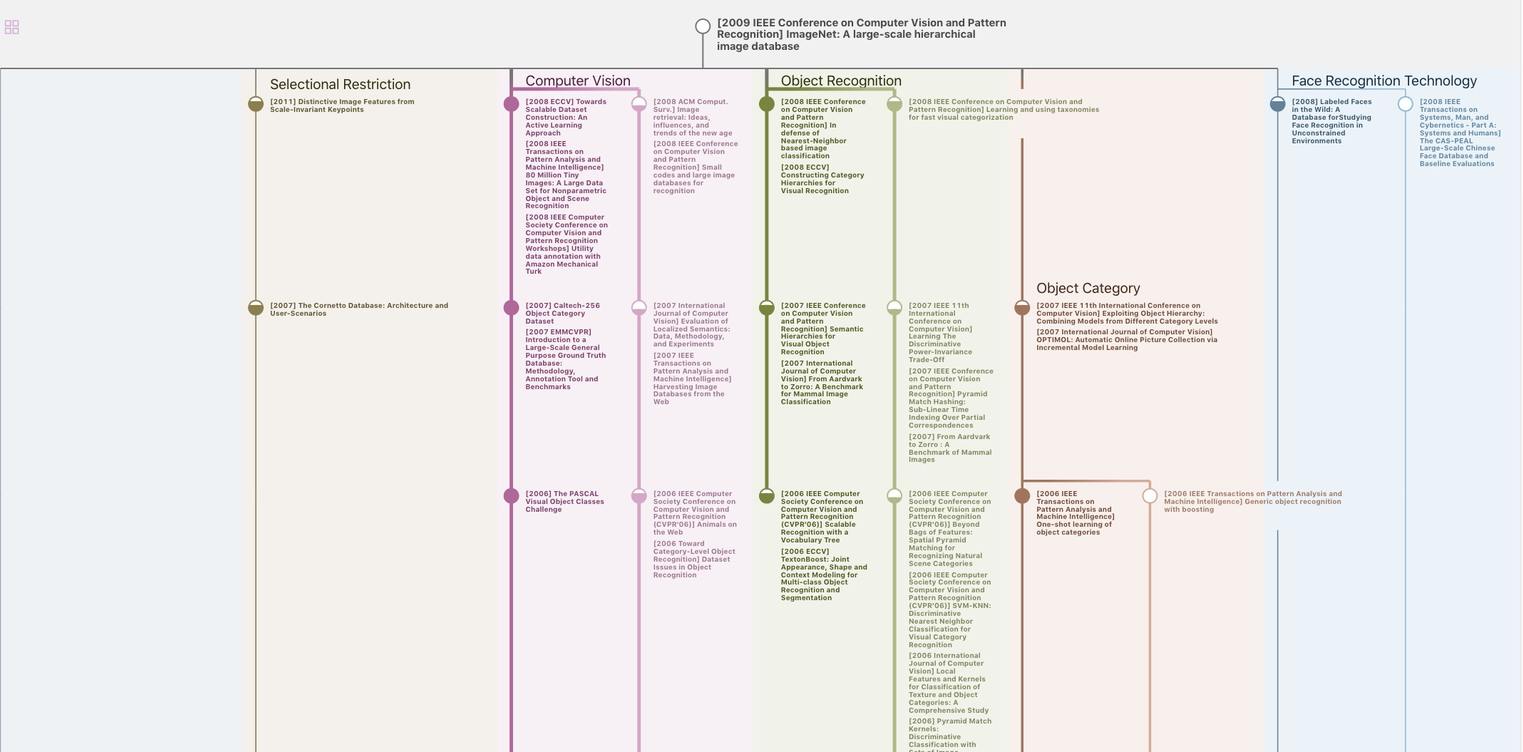
生成溯源树,研究论文发展脉络
Chat Paper
正在生成论文摘要