Evaluating Power Architecture For Distributed Training Of Generative Adversarial Networks
HIGH PERFORMANCE COMPUTING: ISC HIGH PERFORMANCE 2019 INTERNATIONAL WORKSHOPS(2020)
摘要
The increased availability of High-Performance Computing resources can enable data scientists to deploy and evaluate data-driven approaches, notably in the field of deep learning, at a rapid pace. As deep neural networks become more complex and are ingesting increasingly larger datasets, it becomes unpractical to perform the training phase on single machine instances due to memory constraints, and extremely long training time. Rather than scaling up, scaling out the computing resources is a productive approach to improve performance. The paradigm of data parallelism allows us to split the training dataset into manageable chunks that can be processed in parallel. In this work, we evaluate the scaling performance of training a 3D generative adversarial network (GAN) on an IBM POWER8 cluster, equipped with 12 NVIDIA P100 GPUs. The full training duration of the GAN, including evaluation, is reduced from 20 h and 16 min on a single GPU, to 2 h and 14min on all 12 GPUs. We achieve a scaling efficiency of 98.9% when scaling from 1 to 12 GPUs, taking only the training process into consideration.
更多查看译文
关键词
Distributed training, Generative adversarial network, High Performance Computing, GPU, POWER8
AI 理解论文
溯源树
样例
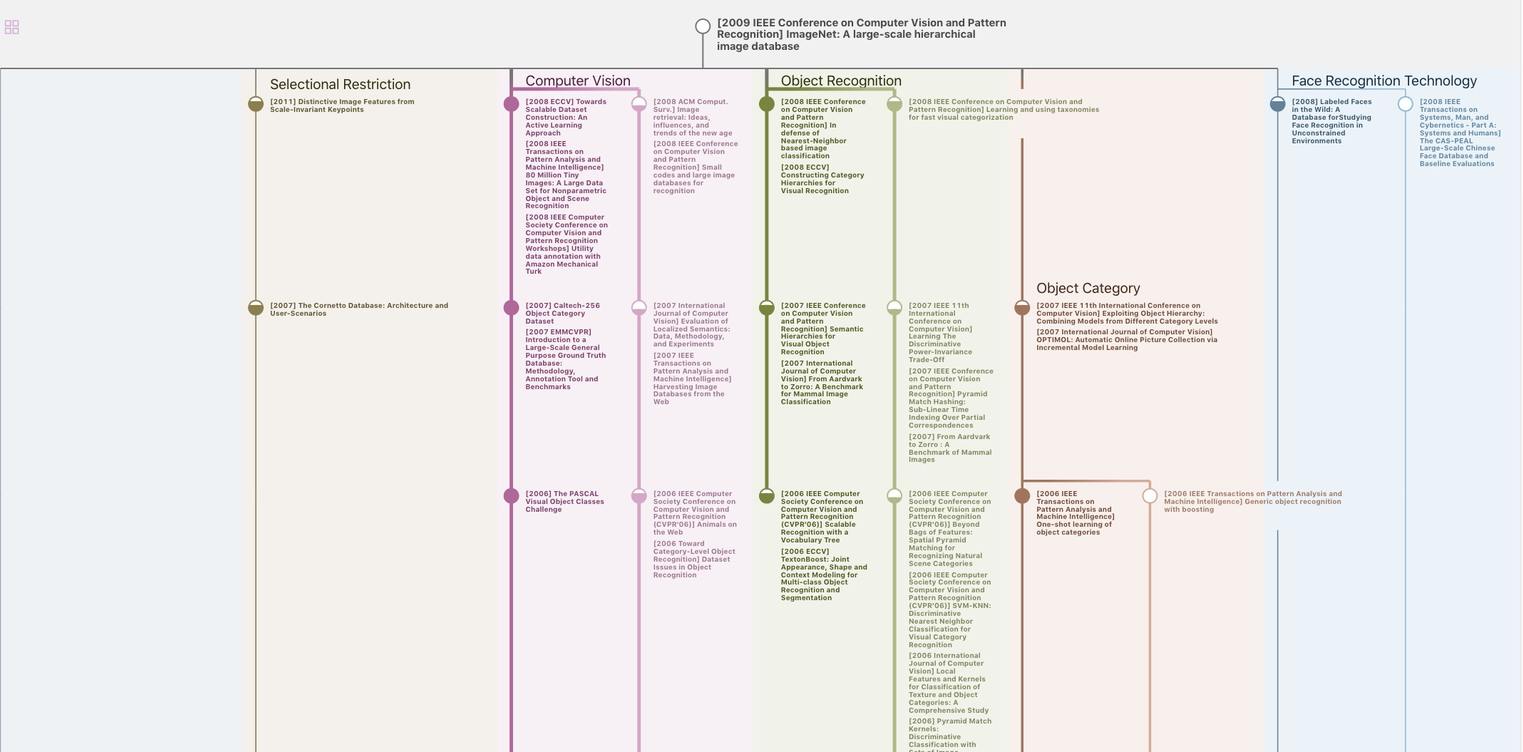
生成溯源树,研究论文发展脉络
Chat Paper
正在生成论文摘要