Is Feature Diversity Necessary in Neural Network Initialization?
arxiv(2019)
摘要
Standard practice in training neural networks involves initializing the weights in an independent fashion. The results of recent work suggest that feature "diversity" at initialization plays an important role in training the network. However, other initialization schemes with reduced feature diversity have also been shown to be viable. In this work, we conduct a series of experiments aimed at elucidating the importance of feature diversity at initialization. Experimenting on a shallow network, we show that a complete lack of diversity is harmful to training, but its effect can be counteracted by a relatively small addition of noise. Furthermore, we construct a deep convolutional network with identical features at initialization and almost all of the weights initialized at 0 that can be trained to reach accuracy matching its standard-initialized counterpart.
更多查看译文
AI 理解论文
溯源树
样例
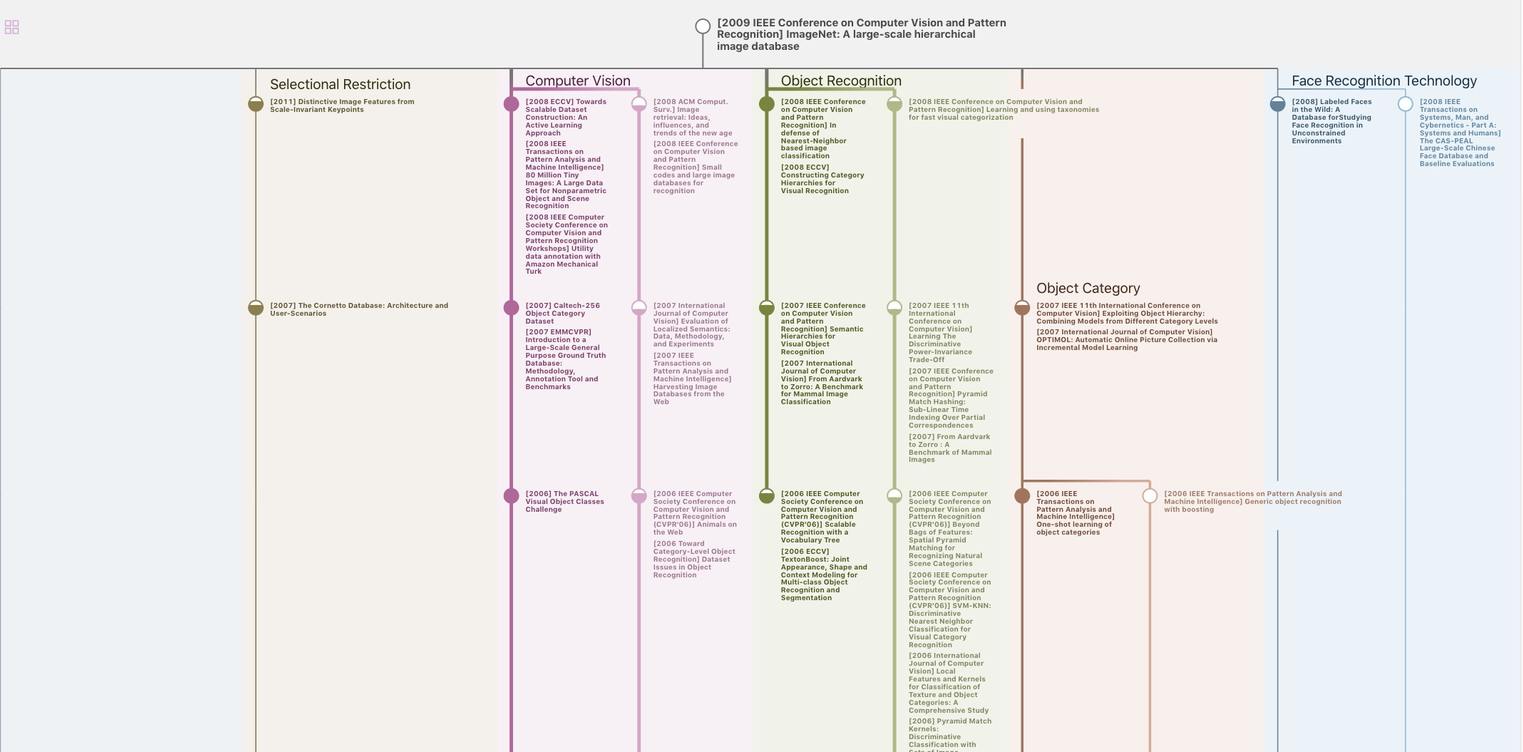
生成溯源树,研究论文发展脉络
Chat Paper
正在生成论文摘要