ABRAHAM: Machine Learning Backed Proactive Handover Algorithm Using SDN
IEEE Transactions on Network and Service Management(2019)
摘要
An important aspect of managing multi access point (AP) IEEE 802.11 networks is the support for mobility management by controlling the handover process. Most handover algorithms, residing on the client station (STA), are reactive and take a long time to converge, and thus severely impact Quality of Service (QoS) and Quality of Experience (QoE). Centralized approaches to mobility and handover management are mostly proprietary, reactive and require changes to the client STA. In this paper, we first created an Software-Defined Networking (SDN) modular handover management framework called HuMOR, which can create, validate and evaluate handover algorithms that preserve QoS. Relying on the capabilities of HuMOR, we introduce ABRAHAM, a machine learning backed, proactive, handover algorithm that uses multiple metrics to predict the future state of the network and optimize the load to ensure the preservation of QoS. We compare ABRAHAM to a number of alternative handover algorithms in a comprehensive QoS study, and demonstrate that it outperforms them with an average throughput improvement of up to 139%, while statistical analysis shows that there is significant statistical difference between ABRAHAM and the rest of the algorithms.
更多查看译文
关键词
Handover,Measurement,Quality of service,Quality of experience,Prediction algorithms,IEEE 802.11 Standard
AI 理解论文
溯源树
样例
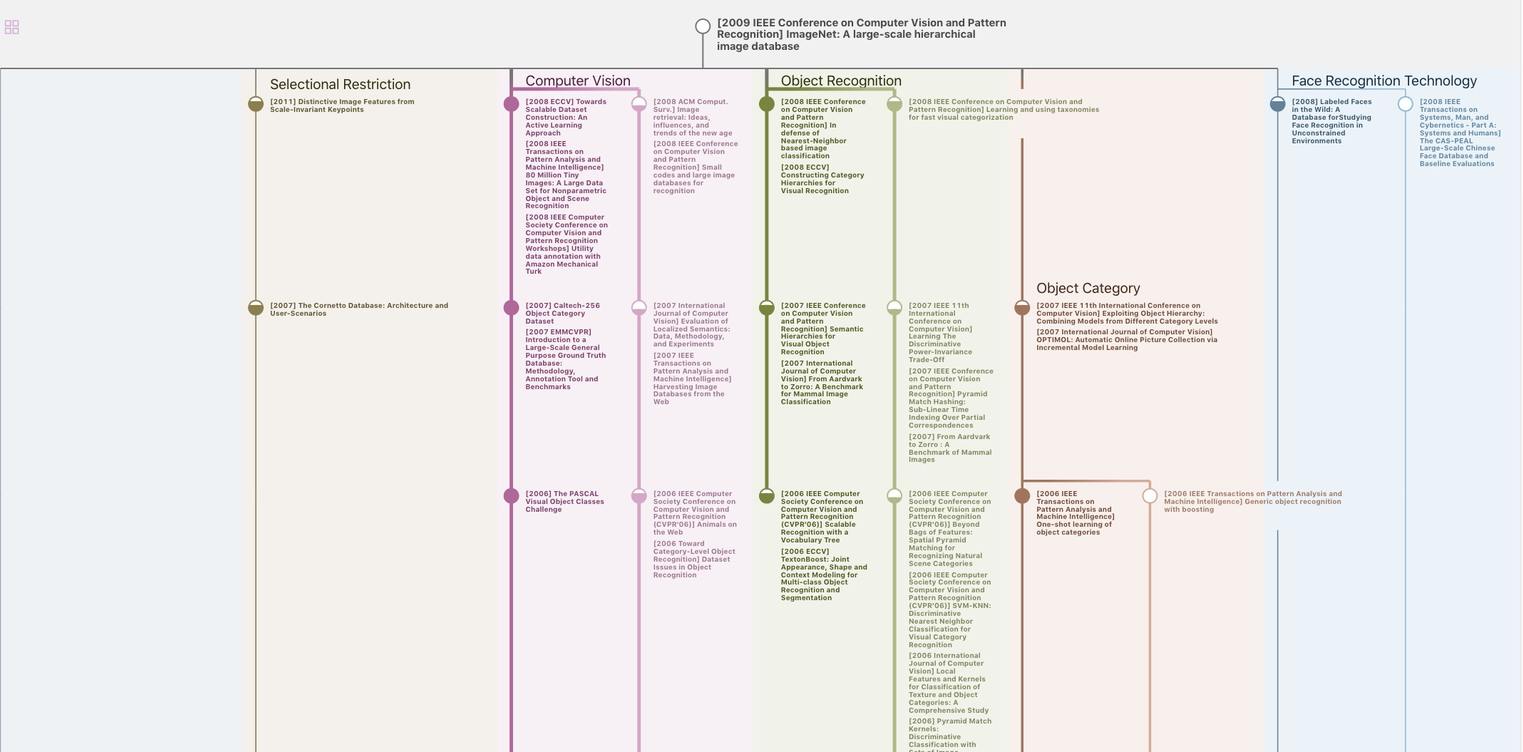
生成溯源树,研究论文发展脉络
Chat Paper
正在生成论文摘要