Linking glycemic dysregulation in diabetes to symptoms, comorbidities, and genetics through EHR data mining.
ELIFE(2019)
摘要
Diabetes is a diverse and complex disease, with considerable variation in phenotypic manifestation and severity. This variation hampers the study of etiological differences and reduces the statistical power of analyses of associations to genetics, treatment outcomes, and complications. We address these issues through deep, fine-grained phenotypic stratification of a diabetes cohort. Text mining the electronic health records of 14,017 patients, we matched two controlled vocabularies (ICD-10 and a custom vocabulary developed at the clinical center Steno Diabetes Center Copenhagen) to clinical narratives spanning a 19 year period. The two matched vocabularies comprise over 20,000 medical terms describing symptoms, other diagnoses, and lifestyle factors. The cohort is genetically homogeneous (Caucasian diabetes patients from Denmark) so the resulting stratification is not driven by ethnic differences, but rather by inherently dissimilar progression patterns and lifestyle related risk factors. Using unsupervised Markov clustering, we defined 71 clusters of at least 50 individuals within the diabetes spectrum. The clusters display both distinct and shared longitudinal glycemic dysregulation patterns, temporal cooccurrences of comorbidities, and associations to single nucleotide polymorphisms in or near genes relevant for diabetes comorbidities.
更多查看译文
关键词
EHR,comorbidities,computational biology,diabetes,diabetes subtypes,epidemiology,genotyping,global health,human,systems biology,text mining
AI 理解论文
溯源树
样例
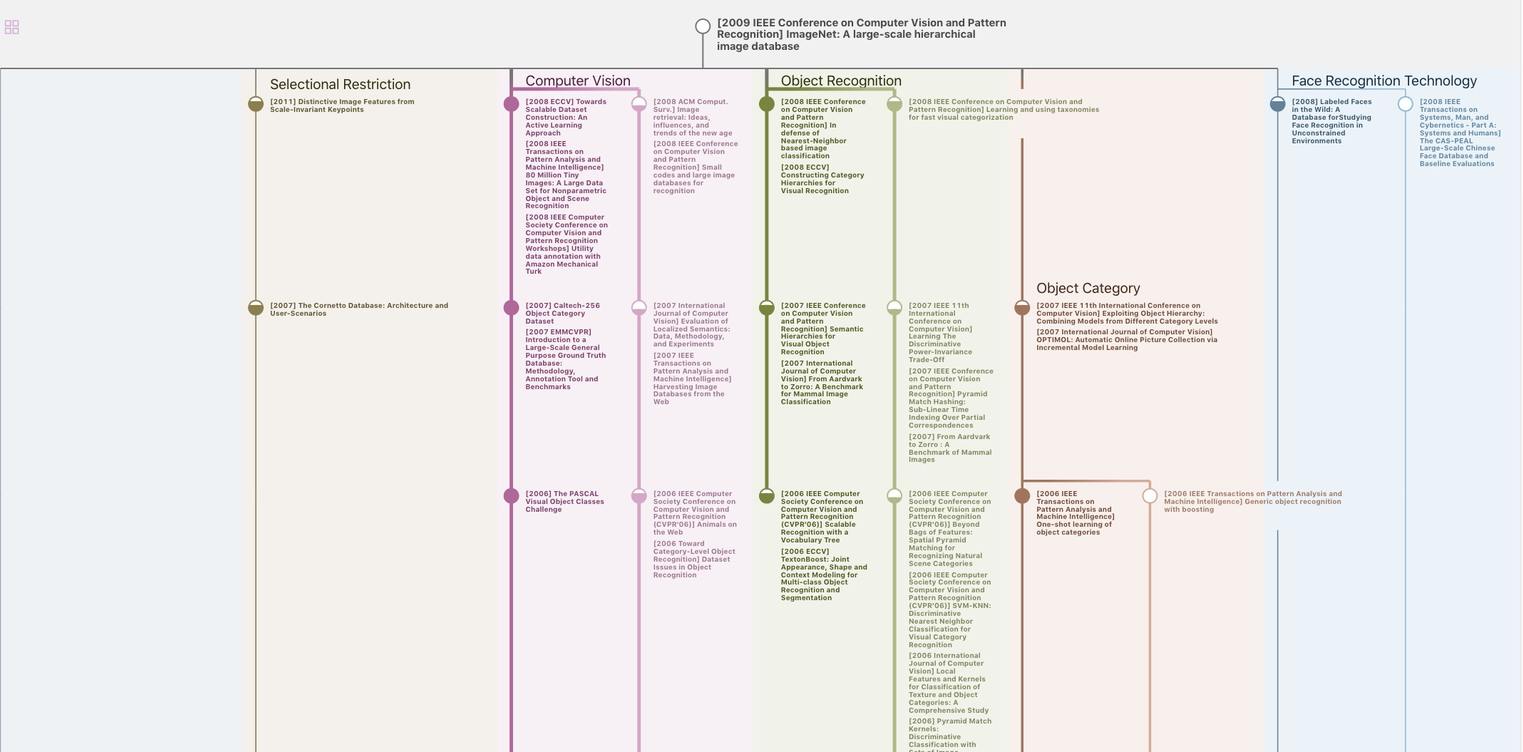
生成溯源树,研究论文发展脉络
Chat Paper
正在生成论文摘要