Driver Detection from Objective Criteria Describing the Driving Style of Race Car Drivers.
ITSC(2019)
摘要
This work sets out to investigate detecting the driver from previously introduced objective criteria, which describe the driving style of race cars drivers. The original dataset is expanded to three full race seasons. A supervised learning approach using Random Forest is tested on different variants of the available data: Direct use of the corner specific observations, averaged values for all features summarising all corners within a single lap as well as a majority-vote of all predictions from the corners within a lap. Additionally a variation of hyperparameters for the used classifier is conducted. The best classification is seen for the majority vote method in combination with 500 trees in the forest, a minimal node size of two and ten possible splitting variables per node with an accuracy value of 95.9% on the test set.
更多查看译文
关键词
majority vote method,single lap,random forest classifier,hyperparameter variation,corner specific observations,supervised learning approach,race cars drivers,objective criteria,driving style,driver detection,test set
AI 理解论文
溯源树
样例
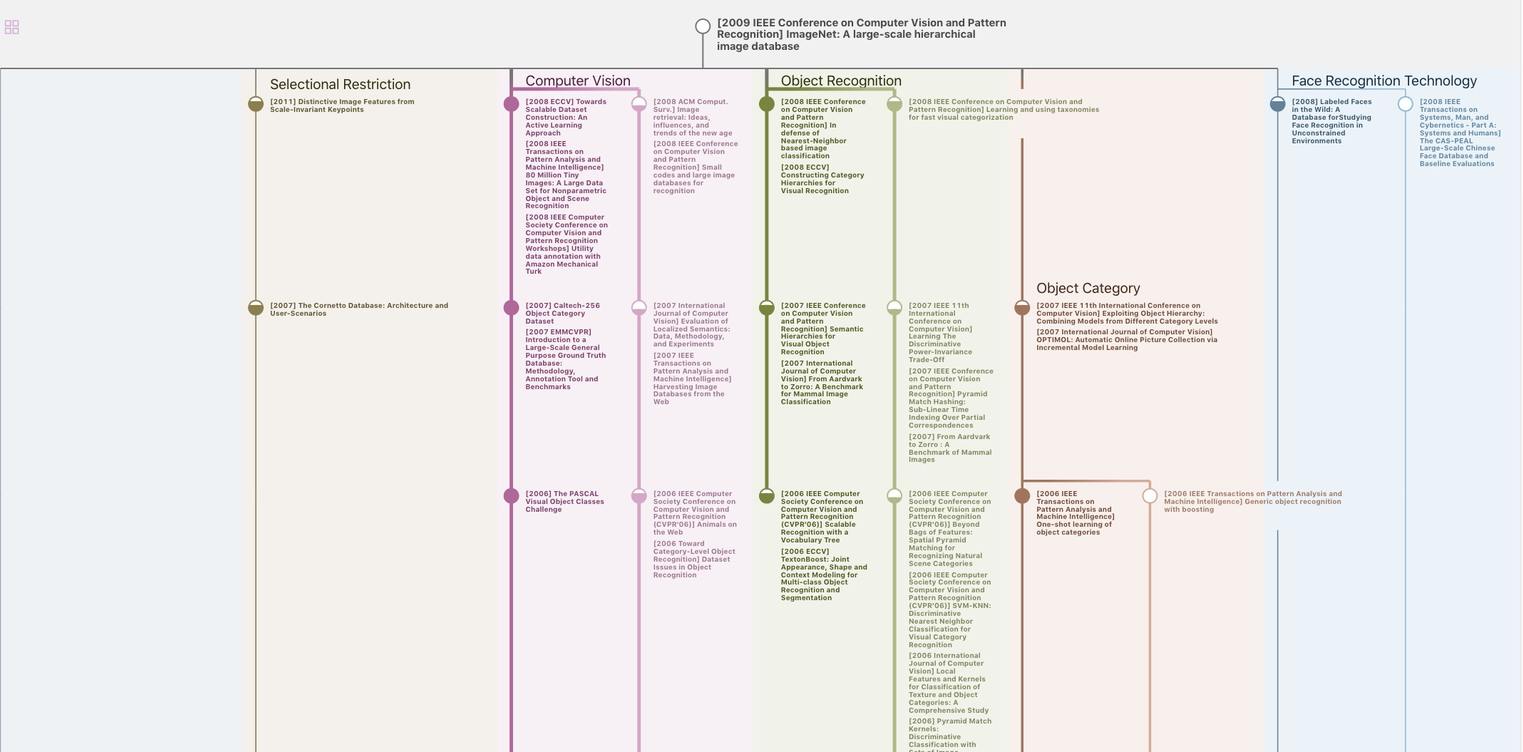
生成溯源树,研究论文发展脉络
Chat Paper
正在生成论文摘要