Analysis of Race Car Drivers' Pedal Interactions by means of Supervised Learning.
ITSC(2019)
摘要
In this paper, throttle and brake pedal interactions from ten race drivers over three seasons of professional motorsport are studied. Time series data, which were logged on race cars, are analyzed with 14 manually engineered metrics using a previously established method. It is determined to which extent race car drivers can be differentiated by their pedal interactions. Supervised learning models in form of multi layered perceptron artificial neural networks and random forest decision trees are utilized to classify the drivers. It is possible to distinguish the drivers with an accuracy of 77% from each other using the input data of one corner. Furthermore, a driver can be detected reliably over different sessions, tracks and seasons. The results indicate that race drivers, while achieving very similar lap times in a top level racing series, have a distinct driving style in terms of pedal interactions. One possible application is the analysis of the driving style at a driver-in-the-loop-simulator. By making use of the presented method, it can be determined if a driver behaves similar to reality on the pedal control input level.
更多查看译文
关键词
throttle,brake pedal interactions,race drivers,time series data,extent race car drivers,supervised learning models,multilayered perceptron artificial neural networks,random forest decision trees,level racing series,driver-in-the-loop-simulator,pedal control input level,manually engineered metrics
AI 理解论文
溯源树
样例
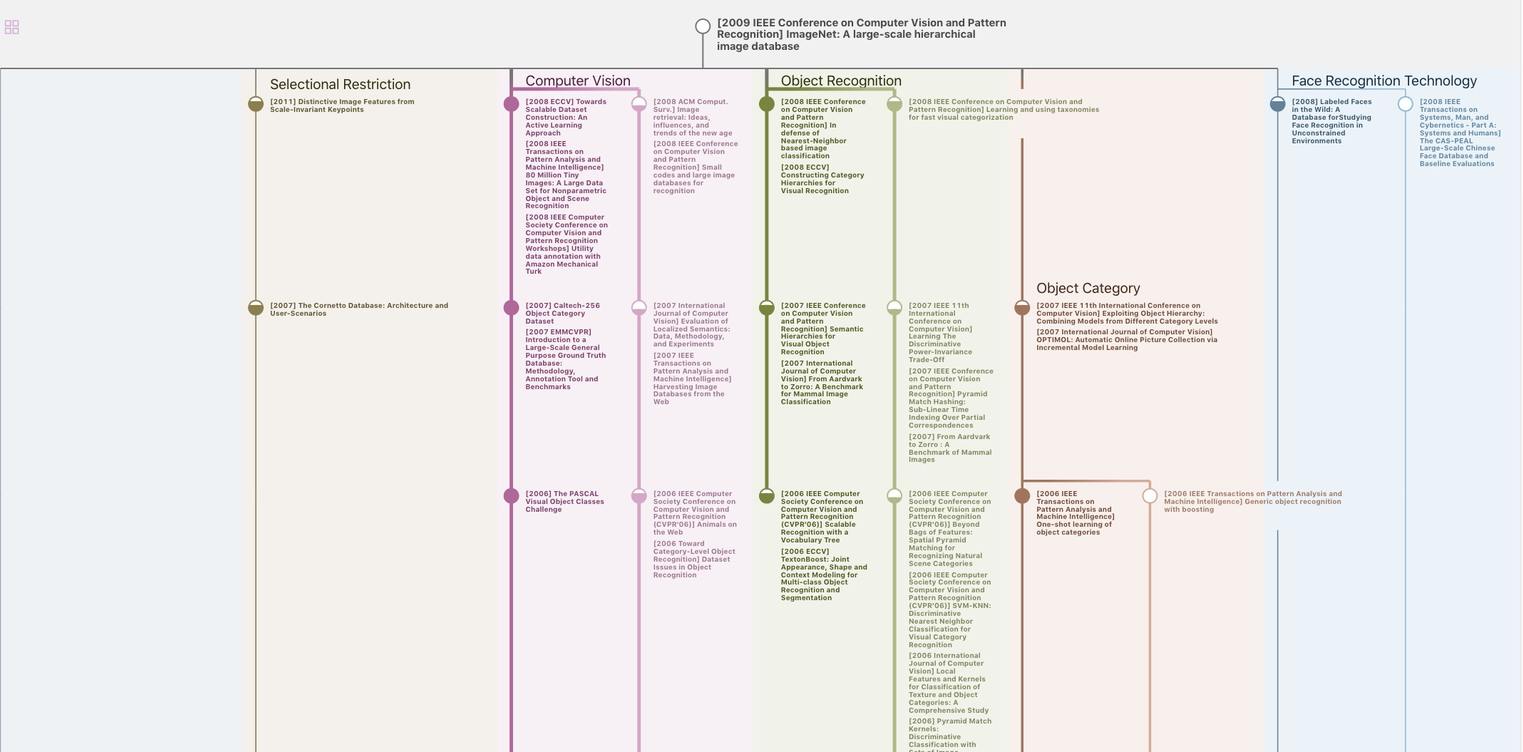
生成溯源树,研究论文发展脉络
Chat Paper
正在生成论文摘要