Multi-Lane Cruising Using Hierarchical Planning And Reinforcement Learning
2019 IEEE INTELLIGENT TRANSPORTATION SYSTEMS CONFERENCE (ITSC)(2019)
摘要
Competent multi-lane cruising requires using lane changes and within-lane maneuvers to achieve good speed and maintain safety. This paper proposes a design for autonomous multi-lane cruising by combining a hierarchical reinforcement learning framework with a novel state-action space abstraction. While the proposed solution follows the classical hierarchy of behavior decision, motion planning and control, it introduces a key intermediate abstraction within the motion planner to discretize the state-action space according to high level behavioral decisions. We argue that this design allows principled modular extension of motion planning, in contrast to using either monolithic behavior cloning or a large set of handwritten rules. Moreover, we demonstrate that our state-action space abstraction allows transferring of the trained models without retraining from a simulated environment with virtually no dynamics to one with significantly more realistic dynamics. Together, these results suggest that our proposed hierarchical architecture is a promising way to allow reinforcement learning to be applied to complex multi-lane cruising in the real world.
更多查看译文
关键词
hierarchical planning,reinforcement
AI 理解论文
溯源树
样例
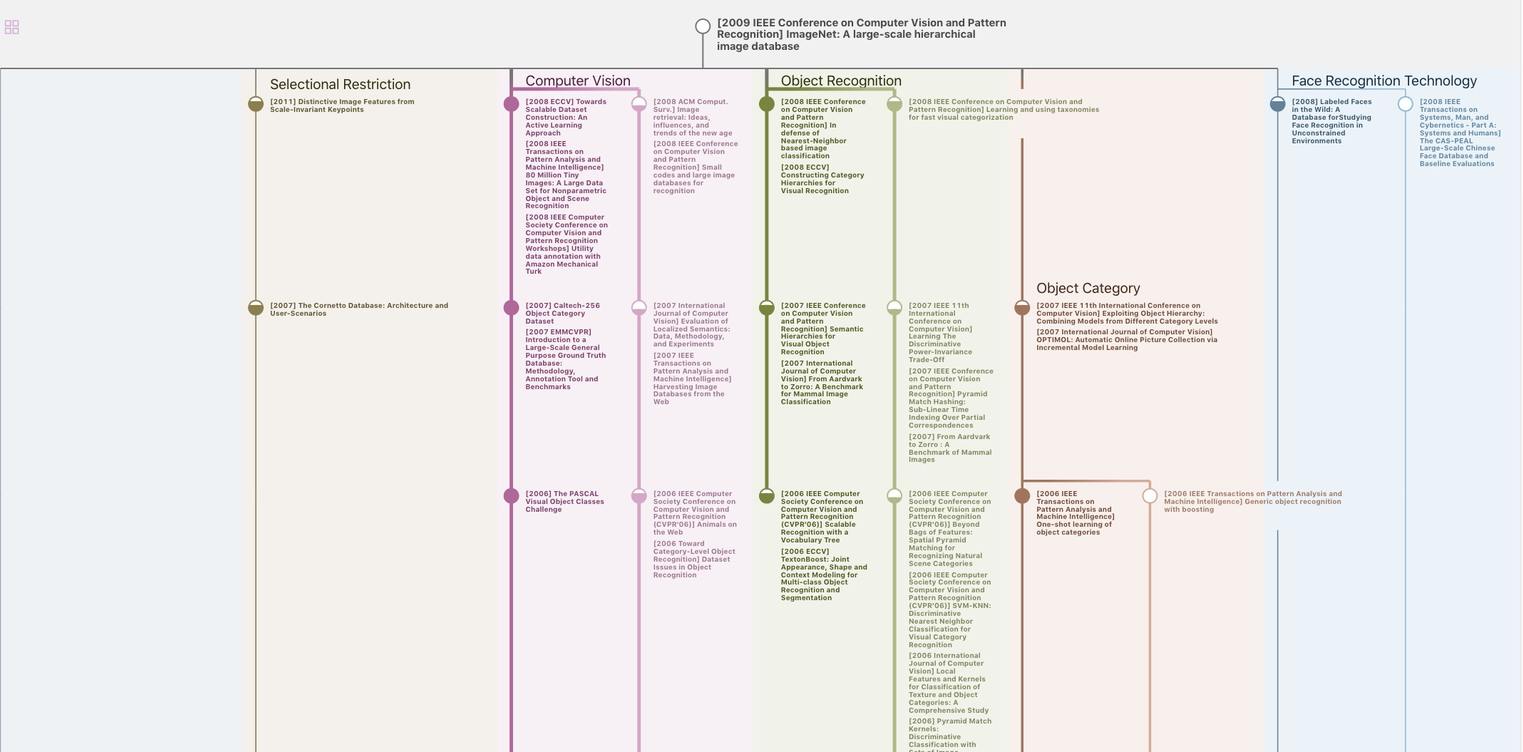
生成溯源树,研究论文发展脉络
Chat Paper
正在生成论文摘要