Calibrating Uncertainty Models For Steering Angle Estimation
2019 IEEE INTELLIGENT TRANSPORTATION SYSTEMS CONFERENCE (ITSC)(2019)
摘要
Various approaches to end-to-end vehicle control using deep neural networks have been proposed recently, examining various architectures to predict steering angles based on raw sensor data. However, most of these approaches are only used as black boxes, which work well in most scenarios and drive vehicles in real traffic, but it is unclear when they will fail. In order to use such models in larger architectures used in autonomous vehicles, they need to reason about decisions or at least provide an additional measure of confidence that captures the uncertainty of the model. In this paper, we introduce and motivate different uncertainty models, comparing Monte Carlo dropout with network architectures based on the bootstrap ensembling method and a Gaussian mixture for the task of end-to-end vehicle control. Furthermore, we evaluate the presented uncertainty models regarding their driving performance as well as their model uncertainty calibration. The model calibration can be regarded as a measure of how well an uncertainty estimates fits to the expected performance. Well calibrated uncertainty estimates are crucial when embedding deep learning models into probabilistic models.
更多查看译文
关键词
sensor data,autonomous vehicles,network architectures,end-to-end vehicle control,model uncertainty calibration,probabilistic models,steering angle estimation,deep neural networks,deep learning models
AI 理解论文
溯源树
样例
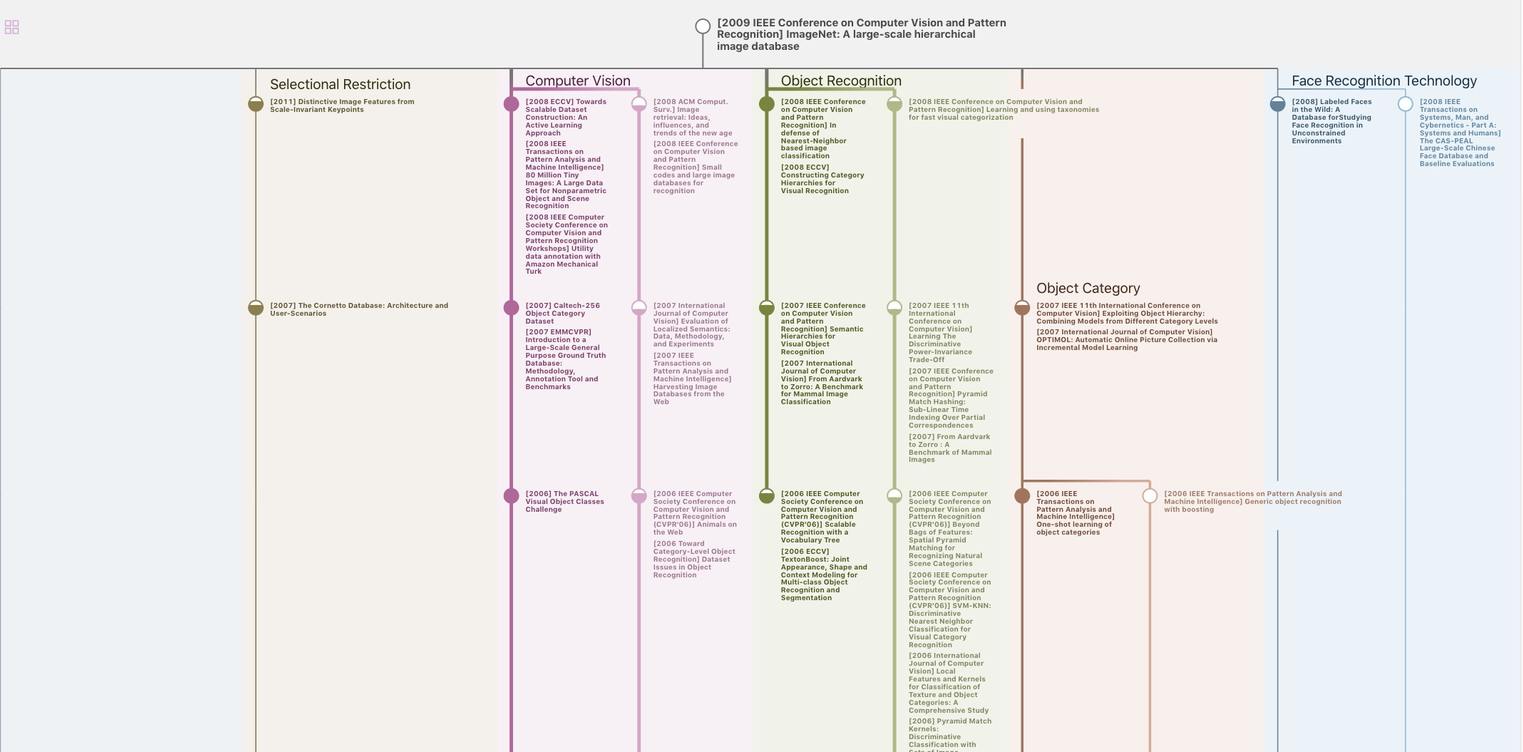
生成溯源树,研究论文发展脉络
Chat Paper
正在生成论文摘要