Convolutional Trajectory Similarity Model: A Faster Method For Trajectory Similarity Measurement
2019 IEEE INTELLIGENT TRANSPORTATION SYSTEMS CONFERENCE (ITSC)(2019)
摘要
Similarity measurement between different trajectory sequences is one of the most fundamental problems in trajectory data mining. In this paper, we propose a parameter sharing supervised model, Convolutional Trajectory Similarity Model (CTSM), based on densely connected one-dimensional convolution layers to efficiently extract spatial-temporal features and to greatly keep accuracy and orderliness computed by Dynamic Time Wrapping (DTW) similarity. We use the downsampling GPS data of cars in Beijing to construct our trajectory dataset. In the training phase, we use a large amount of data to make the model fit the DTW similarity. In the testing phase, we enable the model to predict the similarity between any two trajectories. Our experiments show that CTSM exceedingly decreases the time consumption compared with DTW similarity acceleration algorithm on both fixed-length trajectory dataset and variable-length trajectory dataset. Besides, CTSM performs better than widely used Deep Neural Network (DNN) based models, and uses a much smaller amount of parameters.
更多查看译文
关键词
DTW similarity acceleration algorithm,fixed-length trajectory dataset,variable-length trajectory dataset,CTSM,deep neural network,trajectory data mining,one-dimensional convolution layers,convolutional trajectory similarity model,dynamic time wrapping similarity,GPS data
AI 理解论文
溯源树
样例
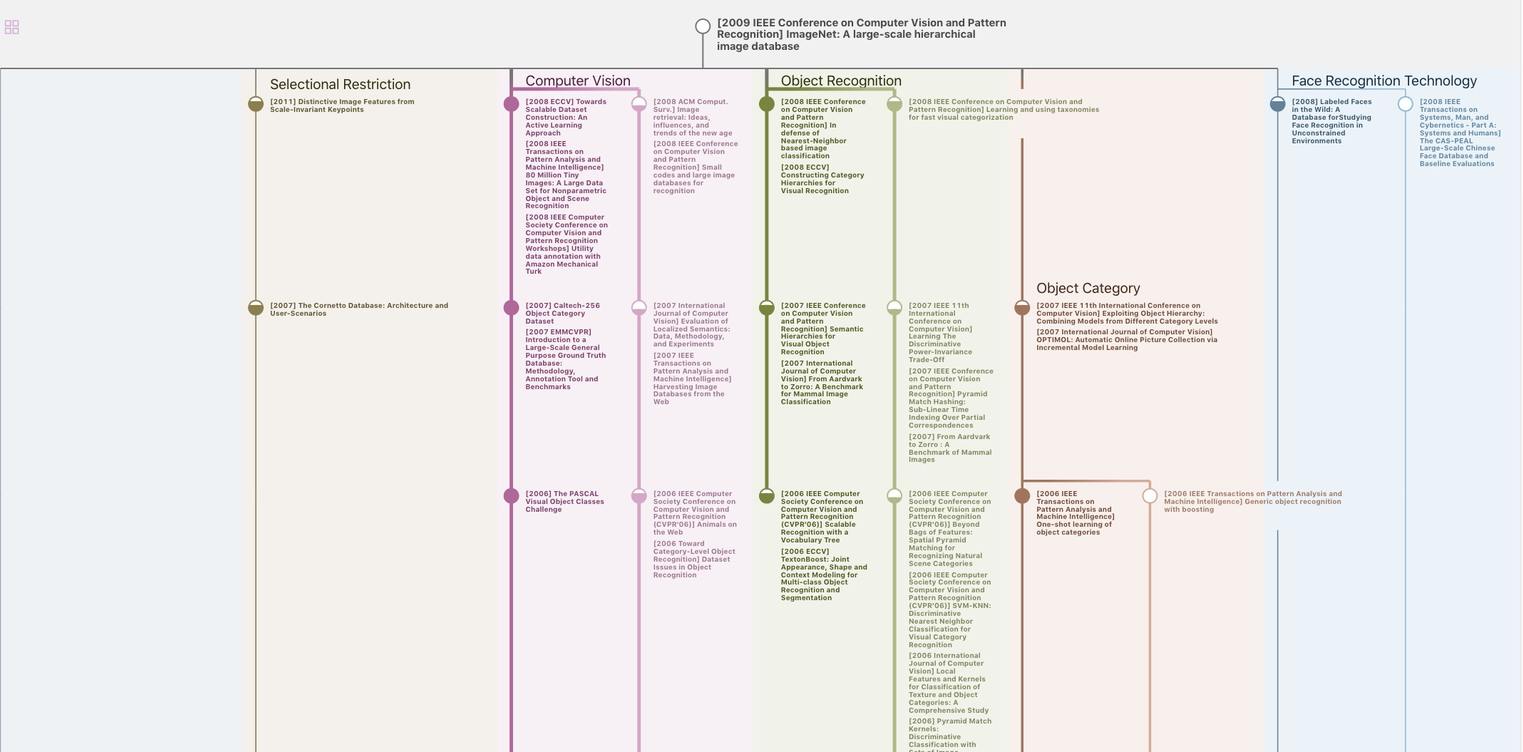
生成溯源树,研究论文发展脉络
Chat Paper
正在生成论文摘要