Analysis Of The Effect Of Various Input Representations For Lstm-Based Trajectory Prediction
2019 IEEE INTELLIGENT TRANSPORTATION SYSTEMS CONFERENCE (ITSC)(2019)
摘要
The prediction of future trajectories of the surrounding traffic participants is a key component in modern autonomous driving systems. This work presents an analysis of the impact of various representations of the input data on the prediction quality. The analyzed data comprises information recorded by the ego vehicle, including object recognition and object tracking, as well as satellite images and map information. We propose a neural network utilizing long shortterm memories (LSTMs) to capture the sequence-to-sequence nature of the underlying problem, as well as a convolutional neural network (CNN) to take the surroundings of the predicted object into account. The input to our network is both the past trajectory of the predicted object, as well as a bird's eye representation of the scene surrounding the object, fusing various types of information on the scene, e.g., a satellite image and bounding boxes of other traffic participants. We achieve Euclidean distances between the predicted position and the ground truth position of 0.47m and 6.19m for a prediction time instant that is 1 s and 6 s in the future, respectively. Additionally, we show the potential of our approach to transfer knowledge from similar road topologies to unseen intersections.
更多查看译文
关键词
autonomous driving systems,ego vehicle,object recognition,satellite image,map information,long short-term memories,sequence-to-sequence nature,convolutional neural network,bounding boxes,input representations,LSTM-based trajectory prediction,traffic participants,object tracking,CNN,bird eye representation,road topologies
AI 理解论文
溯源树
样例
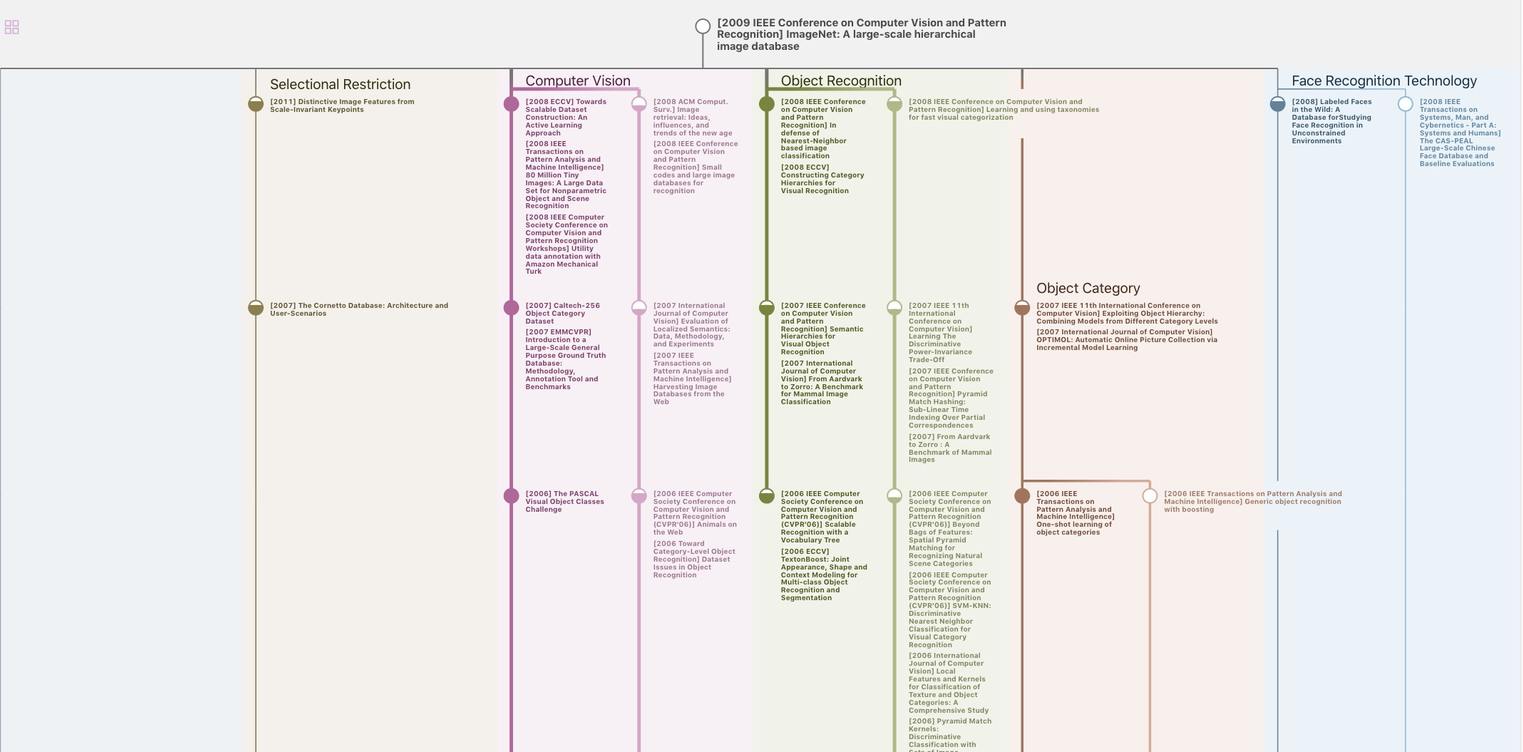
生成溯源树,研究论文发展脉络
Chat Paper
正在生成论文摘要